利用無母數分類方法辨識動態車輛
全文
(2) 利用無母數分類方法辨識動態車輛 The Recognition of Traveling Vehicles by Nonparametric Discrimination of Functional Data with Different Proximity. 研 究 生:呂永在. Student: Yung-Tsai Lu. 指導教授:周幼珍. Advisor: Yow-Jen Jou. 國 立 交 通 大 學 統計學研究所 碩 士 論 文. A Thesis Submitted to Institute of Statistics College of Science National Chiao Tung University in Partial Fulfillment of the Requirements for the Degree of Master in Statistics June 2007. Hsinchu, Taiwan, Republic of China 中華民國九十六年六月.
(3) 利用無母數分類方法辨識動態車輛 研究生:呂永在. 指導教授:周幼珍 博士. 國立交通大學統計學研究所. 摘要 塞車是日常生活中常見的問題,塞車主因之一是車道不足或車道無法 有效利用,所以辨識常塞車路段的車種,統計各車種流量,將有助於 車道、專用車道及交通號誌的建置與控管,進而有效解決塞車問題。 由於雷達微波辨識系統建置成本較影像辨識系統低廉,本文所考慮是 一組雷達微波資料集,資料包含反射波強度及車種的紀錄,資料經由 適當的截取及平移,此筆資料可視為函數資料,因此利用函數資料的 無母數分群方法來辨識車種,並比較三種不同函數資料的近似度測度, 找出辨識率最高的方法。. i .
(4) The Recognition of Traveling Vehicles by Nonparametric Discrimination of Functional Data with Different Proximity. Student:Yung-Tsai Lu. Advisor:Dr. Yow-Jen Jou. Institute of Statistic National Chiao Tung University. ABSTRACT Traffic congestion is a serious and general problem in our daily life. Real time traveling vehicle information is essential to the advanced traffic management. The recognition and statistics of traffic flow among different types of traveling vehicles would be contributive to improve traffic block. This thesis considers the dataset recorded by the Radar microwave detector with the intensity of back waves and the types of traveling vehicles. The data is treated as functional data and then classification would be proposed to be performed by nonparametric discrimination of functional data with three forms of Proximity. The proximity with lower misclassification rate would be adopted for the data. The results show that the misclassification rate is pretty satisfactory if the number of groups is two and as the number of groups increases the misclassification rate increases as expected.. ii .
(5) 誌 謝 這篇論文能順利完成,最先要感謝的是我的指導教授周幼珍老師,老師耐心 且細心的指導,讓我對研究方向愈來愈清楚,論文才能逐步完成。在生活及課業 上老師給予的建議都讓我獲益匪淺。再來要感謝卓訓榮老師及運輸所的學長及同 學們,由於大家的協助及努力,才能實測到這些雷達車輛資料,實測過程現在回 想起來還真是有趣及充滿驚奇,後續資料分析及處理也感謝大家所給予的協助。 再來要感謝洪志真、徐南蓉及胡殿中老師,老師在百忙當中抽空當我的口試 委員,詳讀我的論文且給予許多的建議,讓我的論文更趨完整,謝謝各位老師。 當然也要感謝統計所的同學們,兩年來不管是課業上的討論,還是中午吃飯 八卦聊是非或是運動打籃球都歷歷在目。俊睿、阿Q、柯董、建威、阿淳、益銘 侑峻、小B在我念書念得煩雜時陪我打打籃球,運動打發時間。雪芳、素梅、雅 莉、茞慈、花花、怡君、小米、美惠、映伶、小賴大家常一起吃飯聊天,要是沒 有你們日子還真的有點無聊。最令人印象深刻的當然是我們的沙巴之旅,我的第 一次出國旅行,玩的很開心,畢業後大家雖各奔東西為自己的理想打拚,希望還 能多多聯絡,日後能再有機會一起出國玩,記著大家十年後的約定。 最要感謝的是我的爸爸媽媽和女友雅雲,有你們在背後默默的支持及照顧, 在我情緒低落時為我加油打氣,才能讓我無後顧之憂地的完成研究所的學業,由 衷地感謝你們。. 呂永在 謹誌于 國立交通大學統計研究所 中華民國九十六年六月. iii .
(6) The Recognition of Traveling Vehicles by Nonparametric Discrimination of Functional Data with Different Proximity Student:Yung-Tsai Lu. Advisor:Yow-Jen Jou. Institute of Statistic National Chiao Tung University Hsinchu, Taiwan June 2007 Abstract Traffic congestion is a serious and general problem in our daily life. Real time traveling vehicle information is essential to the advanced traffic management. The recognition and statistics of traffic flow among different types of traveling vehicles would be contributive to improve traffic block. This thesis considers the dataset recorded by the Radar microwave detector with the intensity of back waves and the types of traveling vehicles. The data is treated as functional data and then classification would be proposed to be performed by nonparametric discrimination of functional data with three forms of Proximity. The proximity with lower misclassification rate would be adopted for the data. The results show that the misclassification rate is pretty satisfactory if the number of groups is two and as the number of groups increases the misclassification rate increases as expected.. Key words: Partial least square regression; Kernel; K Nearest Neighbors; Nonparametric Discrimination; Proximity. 1.
(7) Contents 1 Introduction. 3. 2 Model Specifications and Methodology. 6. 2.1 Prerequisite Notions and Notation . . . . . . . . . . . . . . . . . . .. 6. 2.2 Three Types of Semi-Metrics . . . . . . . . . . . . . . . . . . . . . .. 7. 2.2.1. Semi-Metrics Based on Functional PCA. . . . . . . . . . . .. 7. 2.2.2. Semi-Metrics Based on Functional PLS . . . . . . . . . . . .. 9. 2.2.3. Semi-Metrics Based on Derivatives . . . . . . . . . . . . . .. 13. 2.3 Nonparametric Classification of Functional Data . . . . . . . . . . .. 15. 2.3.1. Method . . . . . . . . . . . . . . . . . . . . . . . . . . . . .. 15. 2.3.2. K Nearest Neighbors Estimator . . . . . . . . . . . . . . . .. 18. 3 Practical Example. 21. 3.1 Data Description . . . . . . . . . . . . . . . . . . . . . . . . . . . .. 21. 3.2 Data Analysis . . . . . . . . . . . . . . . . . . . . . . . . . . . . . .. 25. 4 Conclusion. 34. 2.
(8) 1. Introduction The Radar identification technology is developed fast after the World War II.. The technology is principally used in the recognition of aircrafts and tanks. In military scenarios, the need to reliably identify objective is even more stringent, since erroneous identification could easily result in friendly fire incidents. A common technique for identification of military objective is Identification Friend Foe (IFF). IFF identification is initiated when the interrogator transmits a challenge to the aircraft. Friendly aircraft are supposed to be equipped with a transponder, which replies to the challenge by transmitting an identification code to the interrogator. Some IFF modes of operation require more information to be included in the reply, such as the current aircraft altitude. Hostile aircraft will in general not be able to respond properly to the challenge because of the lack of a (compatible) transponder, and will therefore be identified as hostile (or at least not friendly). Various other identification techniques are used in combination with IFF. For example, friendly aircraft can be required to limit their flight path to pre-defined regions of airspace called corridors. Traditional radars collect and transform the information to form images such as two-dimensional Inverse Synthetic Aperture Radar (ISAR) or a sequence of onedimensional range profile from the raw data, and then classify targets based on these images. Herman (2002) bypassed the image formation and attempted target recognition directly from the received data, which is labeled as Automatic Target. 3.
(9) Recognition (ATR). A Radio Frequency System-on-Chip (RF SoC) using the theory of Frequency Modulated Continuous Waves (FMCW) combining the military Radar identification technology can be used in traffic management. The Radar vehicle recognition system is less expensive and reliable than the recognition of image system.Our approach to recognizing (or, classifying) our targets (vehicles on the road) adopts the same idea of Hermans work, i.e., attempt automatic classification directly the received data. Therefore, we use the nonparametric discrimination of functional data to solve the recognition problem. The aim is to find a robust methodology that classifies a new object into one of a prespecified set of classes. In many scientific disciplines, the observed response from experimentation may be viewed as a continuous curve rather than a scalar or a vector. Main sources from this kind of data are real time monitoring of processes and certain types of longitudinal studies. In the terminology of Ramsay and Silverman (1997), they are called functional data sets. Although the underlying data are made up intrinsically by curves, the observed data are discretized representations of these curves. Therefore, each data curve is represented by a (large) finite-dimensional vector. The practical Radar data set can be treated as the functional data. So the nonparametric discrimination of functional data is adopted suitably for the Radar data set. The rest of this thesis is organized as follows: Section 2 introduces the nonparametric discrimination of functional data with three types of proximity. The three types of proximity contain the PCA-type, PLS-type and Derivatives-type semi4.
(10) metrics. The method of PLS regression is also mentioned compendiously. Section 3 describes the Radar data set and uses the method in the Section 2 to classify the vehicles and lanes. Some analysis results are also mentioned. The conclusion would be proposed in the Section 4.. 5.
(11) 2. Model Specifications and Methodology Functional data sets appear in many areas of science. Although each data point. may be seen as a large finite-dimensional vector it is preferable to think of them as functional data. First we introduce some notation of functional data, then give a brief introduction of nonparametric discrimination with three types of proximity.. 2.1. Prerequisite Notions and Notation. We first introduce some common notation and the terminology that generally used in mathematics. Definition 1. A random variable X is called functional random variable (f.r.v.) if it takes values in an infinite dimensional space. An observation x of X is called a functional data. The X denotes a random curve, X = {X (t), t ∈ T}. Definition 2. k.k is a semi-norm on some space F as long as: 1.∀x ∈ F, kx k ≥ 0. 2.∀(a, x ) ∈ R × F, kax k = |a|kx k. 3.∀(x , y ) ∈ F × F, kx + y k ≤ kx k + ky k. Definiton 3. d is a semi-metric on some space F as long as: 1.∀x ∈ F, d(x , x ) = 0. 2.∀(x , y ) ∈ F × F, d(x , y ) ≥ 0. 3.∀(x , y , z ) ∈ F × F × F, d(x , y ) ≤ d(x , z ) + d(z , y ). Note that in fact, a semi-norm k.k does not have the property that kx k = 0 imply x = 0. Similarly, a semi-metric does not have the property that d(x , y ) = 0 imply 6.
(12) x = y.. 2.2. Three Types of Semi-Metrics. Because most available functional datasets are curves, we will describe three types of semi-metrics well adapted for this kind of data in the following subsection. 2.2.1. Semi-Metrics Based on Functional PCA. Functional Principal Components Analysis (FPCA) is a good tool for computing proximities between curves in a reduced dimensional space. As long as E[ X 2 (t)dt] R. is finite, the FPCA of the f.r.v. X has the following expansion (Dauxois, etc. 1982): X =. ∞ Z X. . X (t)vk (t)dt vk. k=1. with v1 , v2 , . . ., being the orthogonal eigenfunctions of the covariance operator ΓX (s, t) = E(X (s)X (t)) associated with the eigenvalues λ1 ≥ λ2 ≥ . . .. Then the truncated version of the above expansion of X is X˜ (q) =. q Z X. . X (t)vk (t)dt vk .. k=1. The main goal is to find truncated version such that E. Z. 2. . (X (t) − Pq X (t)) dt. is minimized over all projections Pq of X into q-dimensional spaces. According to the classical L2 -norm, we can define a parametric class of semi-norms and semi7.
(13) metrics in the following way: kx kqP CA =. sZ . x˜ (q) (t). 2. v u q Z 2 uX dt = t x (t)vk (t)dt k=1. v u q Z 2 uX P CA t dq (Xi , x ) = [Xi (t) − x (t)] vk (t)dt k=1. In general, ΓX is unknown and then, the vk0 s too. However, the covariance operator can be well approximated by its empirical version ΓnX (s, t) = 1/n. n X. Xi (s)Xi (t).. i=1. The eigenvectors of ΓnX are consistent estimators of eigenvectors of ΓX . In practice, we never observe exactly {x i = {x i (t); t ∈ T }}i=1,...,n but a discrete version n. x i = (x i (t1 ), . . . , x i (tJ ))0. o. i=1,...,n. . So we can approximate the integral in a discrete. way Z. [Xi (t) − x (t)] vk (t)dt ≈. J X. wj (Xi (tj ) − x (tj ))vk (tj ). j=1. where w1 , . . . wJ are weights which define the approximate integration. A standard choice is wj = tj − tj−1 . Similarly, the semi-metric dPq CA (x i , x i0 ) can be approximated by its empirical version v 2 u uX q J X u dPq CA (x i , x i0 ) = t wj (x i (tj ) − x i0 (tj ))vk (tj ) k=1. j=1. where v1 , v2 , . . . , vq , are the W-orthonormal eigenvectors of the covariance matrix Γn W = 1/n. n X. x i x 0i W. i=1. associated with the eigenvelues λ1,n ≥ λ2,n ≥ . . . ≥ λq,n , and W = diag(w1 , . . . , wJ ). 8.
(14) 2.2.2. Semi-Metrics Based on Functional PLS. In this subsection we first introduce partial least squares (PLS) regression and different forms of PLS, then give a brief introduction of semi-metric based on functional PLS. Partial Least Squares Regression: Partial Least Squares is a widespread method for modeling relations between a set of dependent variables and a large set of predictors. PLS generalizes and combines features from principal component analysis and multiple regression. It originated in social sciences (particularly economy, Herman Wold 1966) but become popular first in chemometrics due to Herman’s son Svante, (Geladi and Kowalski, 1986). It was first presented as an algorithm analog to the power method (used for computing eigenvectors) but was suitably interpreted in statistical framework. (Frank and Friedman, 1993; Hoskuldsson, 1988; Helland, 1990; Tenenhaus, 1998). PLS usually is used to predict response variable Y from predictors X and describe their common structure. If X is full rank, ordinary multiple regression can be applied. When the number of predictors is larger than the number of observations, X would be singular and the ordinate multiple regression is no longer practicable. Several methods have been developed to solve this problem, e.g. principal component regression. But the method is chosen to explain the variation of X, nothing guarantees that the choice to explain X are suitable for Y. PLS regression finds components from X that are also connected with Y. It searches for a. 9.
(15) set of components (latent vectors) that performs a simultaneous decomposition of X and Y with the constraint that these components explain as much as possible of the covariance between X and Y. Let X be the zero-mean (n × N) matrix and Y be the zero-mean (n × M) matrix, where n denotes the number of data sample. PLS decomposes X and Y into the form X = TP0 + E Y = UQ0 + F. (1). where the T, U are (n × p) matrix of the p extracted components, the (N × p) matrix P and the (M × p) matrix Q represent loading matrices and the (n × N) matrix E and the (n × M) matrix F are the residual matrices. PLS which is based on the nonlinear iterative partial least squares (NIPALS) algorithm finds weight vectors w, c such that [cov(t, u)]2 = [cov(Xw, Yc)]2 = max(|r|=|s|=1) [cov(Xr, Ys)]2 where cov(t, u) = t0u/n denotes the sample covariance between the components t and u . The NIPALS algorithm starts with random initial value of the component u and repeats a sequence of following steps until convergence. Step 1. w = X0 u/(u0 u) (estimate X weights) Step 2. kwk → 1 Step 3. t = Xw (estimate X component) Step 4. c = Y 0 t/(t0t) (estimate Y weights) 10.
(16) Step 5. kck → 1 Step 6. u = Yc (estimate Y component) Specially, u = y if M = 1, Y is a one-dimensional vector that is denoted by y. In this case the NIPALS procedure converges in a single iteration. It can be shown that the weight vector w also corresponds to the first eigenvector of the following series of equations: w ∝ X0u ∝ X0Yc ∝ X0 YY 0t ∝ X0 YY 0Xw. This shows that the weight vector w is the right singular vector of the matrix X0 Y. Similarly, the weight vectors c is the left singular vector of X0 Y. Then the latent vectors t and u are given as t=Xw and u=Yc, where the weight vector c is defined in steps 4 and 5 as stated above. Similarly, the extraction of t, u or c estimates can be derived. Forms of PLS: PLS is an iterative process. After the latent vectors t and u being extracted, the matrices X and Y are extracted by subtracting their rank-one approximations based on t and u. Different extractions form several variants of PLS. By equation (1) the loading vectors p and q are computed as coefficients of regressing X on t and Y on u, respectively. Then, the loading vectors can be solved by p = X0 t/(t0 t) and q = Y0 u/(u0 u). 1.) PLS Mode A: The PLS Mode A is based on rank-one deflation of individual matrices using the corresponding latent and loading vectors. In this case, the X and Y matrices are extracted X = X−tp0 and Y = Y −uq0 . This method was originally proposed 11.
(17) by Herman Wold (1966) to model the relations between the different sets of data. 2.) PLS1 and PLS2: PLS1 (either dependent variable or independent variable consists of a single variable) and PLS2 (both variables are multidimensional) are used as PLS regression method. The form of PLS is the most popular PLS approach. The main feature of the approach is that the relation between of X and Y is asymmetric. The main assumptions of the form of PLS are: p (i) The latent vectors {ti }i=1 are good predictors of Y; p denotes the number of extracted latent vectors. (ii) a linear inner relation between the latent vectors t and u exists; that is, U = TD + H where D is the (p × p) diagonal matrix and H is the residual matrix.. The asymmetric assumption of the independent and dependent variable relation is transformed into a deflation scheme. The latent vectors {ti }pi=1 are good predictors of Y. Then the latent vectors are used to deflate Y, that is, a component of the regression of Y on t is removed from Y at each iteration of PLS. X = X − tp0 and Y = Y − tt0 Y/(t0 t) = Y − tc0 where the weight vector c is defined in step 4 of NIPALS. This way of deflation will ensure that the extracted latent vectors {ti }pi=1 are mutually orthogonal. 12.
(18) 3.) SIMPLS: In order to avoid deflation steps at each iteration of PLS1 and PLS2, Jong (1993) has introduced another form of PLS denoted SIMPLS. The SIMPLS ap˜ i }pi=1 which are applied to the original proach directly finds the weight vectors {w n op. matrix X. The criterion of the mutually orthogonal latent vectors ˜ti. i=1. is kept.. Semi-Metrics Based on Functional PLS: Let v1q , . . . , vpq be the vectors of RJ performed by multivariate partial least squares regression (MPLSR) where q denotes the number of the factors and p the number of scalar responses. Then the semi-metric based on the MPLSR is defined as. v 2 u uX p J X u dPq LS (x i , x i0 ) = t wj (x i (tj ) − x i0 (tj ))vkq (tj ) k=1. j=1. where w1 , . . . , wJ are weights which define the approximate integration. A standard choice is wj = tj − tj−1 . When we consider only one scale response (p = 1), the proximity between two discrete curves is due to only one direction, which seems inadequate with regard to the complexity of functional data. However, as soon as we consider multivariate response, such a family of semi-metrics allows to obtain very good results, which is the case in the curves discrimination context. 2.2.3. Semi-Metrics Based on Derivatives. One semi-metrics is to consider a distance between curves among their derivatives. The semi-metric based on derivatives of two observed curves x i and x i0 can. 13.
(19) be defined as dqderiv (x i , x i0 ). =. sZ . (q). (q). 2. x i (t) − x i0 (t) dt. where x (q) denotes the qth derivative of x . Note that dderiv (x i , 0) is the classical L2 0 norm of x . The computation of successive derivatives is very sensitive numerically. In order to overcome the numerical stability problem, we can use a B-spline (Boor, 1978) approximation for the curves. Once we have obtained an analytical Bspline expansion for each curve, the successive derivatives are directly computed by differentiating several times their analytic form. Let {B1 , ..., BB } be a B-spline basis, then the discrete approximative form of the curve x i = (x i (t1 ), . . . , x i (tJ ))0 is as follows: _. _. _. β i = (β i1,..., β iB ) = arg. J X. inf. (α1 ,...,αB )∈RB j=1. x i (tj ) −. B X. αb Bb (tj ). b=1. !2. .. This produces a good approximation of the solution of the minimization problem arg. inf. (α1 ,...,αB )∈RB. Z. x i (t) −. B X. αb Bb (t). b=1. !2. dt.. Therefore, the approximative form of the curve x i = (x i (t1 ), . . . , x i (tJ ))0 is xˆ i (.) =. B X. βˆib Bb (.). b=1. Because the analytic expression of the Bb0 s is well-known, the successive derivatives can be exactly computed and we can differentiate easily the approximated curves: (q) xˆ i (.). =. B X. b=1. 14. (q) βˆib Bb (.)..
(20) Then semi-metric based on derivatives of two observed curves x i and x i0 , can be computed by dderiv q. 2.3. (x i , x i0 ) =. sZ . (q). (q). 2. xˆ i (t) − xˆ i0 (t) dt.. Nonparametric Classification of Functional Data. Classification or discrimination of functional data is in the situation when we observe a f.r.v. X and a categorical response Y which gives the group of each functional observation. The main purpose is to predict the group when we observe a new functional data. In next section we hope to find a robust method for assigning each functional observation to some homogeneous group. We review the nonparametric discrimination method that is proposed by Ferraty and Vieu (2006). 2.3.1. Method. Let (Xi , Yi )i=1,...,n be a sample of n independent pairs, identically distributed as (X , Y) and valued in F × G, where G = {1, . . . , G} and (F, d) is a semi-metric vector space (X is a f.r.v. and d is a semi-metric). The notation (x i , y i ) denotes the observation of the pair (Xi , Yi ).. General classification rule (Bayes rule). Given a functional observation x , the purpose is to estimate the posterior probability pg (x ) = P (Y = g |X = x ), g ∈ G.. 15.
(21) Once the G probabilities are estimated (ˆ p1 (x ), . . . , pˆG (x )), the classification rule consists of assigning an incoming functional observation x to the group with highest estimated posterior probability yˆ (x ) = arg max pˆg (x ). g∈G This classification rule is called Bayes rule. To use a suitable kernel estimator make precise discrimination of functional data.. Kernel estimator of posterior probabilities. Before defining the kernel-type estimator of the posterior probabilities, we remark that pg (x ) = P (I[Y=g] |X = x ), with I[Y=g] equal to 1 if Y = g and 0 otherwise. Therefore we can use kernel-type estimator introduced for the prediction via conditional expectation. pˆg (x ) = pˆg,h (x ) =. n P. I[Y=g] K (h−1 d (x , Xi )) i=1 n P. i=1. ,. K (h−1 d (x , Xi )). where K is the kernel and h is the bandwidth (a strictly positive smoothing parameter). The kernel posterior probability estimate can be rewritten as pˆg,h (x ) =. K (h−1 d (x , Xi )) wi,h (x ) with wi,h (x ) = P . n −1 d (x , X )) K (h i {i:Yi =g} X. i=1. In order to compute the quantity pˆg,h (x ) we use only the Xi ’s belonging to both the group g and the ball centered at x and of radius h. pˆg,h (x ) =. X. wi,h (x ) where I = {i : Yi = g} ∩ {i : d (x , Xi ) < h} .. i∈I. 16.
(22) The closer Xi is to x the larger the quantity K (h−1 d (x , Xi)). Hence, the closer Xi is to x the larger the weight wi,h (x ). So, among the Xi ’s lying to the gth group, the closer Xi is to x and the larger is its effect on the gth estimated posterior probability. As long as K is nonnegative, the kernel estimator has the following interesting properties : (i) 0 ≤ pˆg,h (x ) ≤ 1, (ii). P. g∈G. pˆg,h (x ) = 1,. which ensure that the estimated probabilities are forming a discrete distribution.. Choosing the bandwidth. According to the shape of the kernel estimator, we have to choose the smoothing parameter h. A usual way for an automatic choice of h is constructed from minimizing a loss function Loss as: hLoss = arg min Loss(h), h. where the function Loss can be built from pˆg,h (x )’s and y i ’s. The misclassification rate is a nature choice among different types of Loss functions. Therefore, the functional classification can be performed by the following procedure: Learning step: for h ∈ H for i = 1, 2, . . . , n g = 1, 2, . . . , G 17.
(23) pˆg,h (x i ) =. Pn. enddo. K (h−1 d(x i ,x i0 )) i0 :y i0 =g} {P n K(h−1 d(x i ,x i0 )) i0 =1. enddo enddo hLoss = arg min Loss(h) h∈H. Predicting step: Let x be a new functional observation and yˆ (x ) be its estimated group: yˆ (x ) ← arg max {ˆ pg,hLoss (x )} g∈G where H ⊂ R is a set of suitable values for h and K is a known kernel. 2.3.2. K Nearest Neighbors Estimator. The choices of the bandwidth h and the semi-metric d have great influence on the behavior of the kernel estimator. It is inefficient to choose bandwidth h among the positive real number subset from a computational point of view. So, we consider a general and simple way which is the k nearest neighbors (kNN) version of kernel estimator. Then we can replace a choice of real parameter among an infinite number of values with an integer parameter k (among a finite subset). The main idea of the kNN estimator is to replace the parameter h with hk which is the bandwidth allowing us to take into account k terms in the weighted average. The pg at x is estimate by pˆg,k (x ) =. . −1 {i:y i =g} K hk d (x , x i ) Pn −1 K h d (x , x ) i i=1 k. Pn. 18. .
(24) where hk is a bandwidth such that the number of {i : d (x , x i ) ≤ hk } is k. That is, the minimization problem on h over a subset of R is replaced with a minimization on k over a finite subset {1, 2, . . . , K}: kLoss = arg. min. Loss(k) and hLoss ← hkLoss ,. k∈{1,2,...,K}. where the loss function Loss is now built from pˆg,k (x i )’s and y i ’s. In order to choose the tuning parameter k, we must introduce a loss function (Loss). The loss function allows us to build a local version of the kNN estimator. The main goal is to compute the quantity:. pLCV (x ) = g. Pn. {i:y i =g} K Pn. i=1. K. . . d(x ,x i ) hLCV (x i0 ). d(x ,x i ) hLCV (x i0 ). . . where i0 = arg min d (x , x i ) and hLCV (x i0 ) is the bandwidth corresponding to i=1,2,...,n. the optimal number of neighbors at x i0 obtained by the following cross-validation procedure: kLCV (x i0 ) = arg min LossLCV (k, i0 ), k. where LossLCV (k, i0 ) =. G X. g=1. I[y. (−i ). i0. 0 =g] − pg,k (x i0 ). and (−i ). pg,k 0 (x i0 ) =. Pn. {i:y i =g,i6=i0 } K Pn. i=1,i6=i0 K. . . d(x i0 ,x i ) hk (x i0 ). d(x i0 ,x i ) hk (x i0 ). . 2. . .. As long as we set up the appropriate semi-metric and kernel function K(.), the prediction procedure is finished. 19.
(25) The misclassification rate for the learning sample (x i , y i )i=1,2,...,n (the sample of curves for which the groups are observed) will be used to assess the performance of the predicted results, the procedure is described as follows: for i = 1, 2, . . . , n y LCV ← arg i. max. g∈{1,2,...,G}. pLCV (x i ) g. enddo Misclas ←. 1 n. n P. i=1. I[y 6=y LCV ] i i. 20.
(26) 3. Practical Example The procedures described in previous sections will be applied to a real data. set. We consider a real data set which is recorded by Radar microwave detector. The collection of the data would be introduced in section 4.1. Then some analysis results would be shown in section 4.2.. 3.1. Data Description. The practice data set are collected near the section 1 of Singlong Road, Jhubei City, Hsinchu County by vehicle Radar microwave detector, which is a side-looking configuration. There are four lanes on the Singlong Road. The collection time is from 10:00 AM to 5:00 PM on October 11, 2006. These vehicles with speed 40 km/hour are recorded on the four lanes of Singlong Road. There are 248 observed curves of vehicles recorded. We do registration in the original dataset, and take the peak of intensity of back wave as the marker. So the observed curves are shifted and truncated, then we take the adjusted curves as the analytic curves. Each of curves represents the intensity of back wave at 30 discrete observed points and the types of vehicles. So we observe n = 248 pairs (x i , y i )i=1,...,n where x i = (x i (t1 ) , x i (t2 ) , . . . , x i (t30 ))0 correspond to the ith discrete functional data and y i give the class of the ith observation. Given a new functional observation x , our main goal is to predict the corresponding class of vehicle and lane y LCV . The classes of vehicles contain small and large vehicles. The small vehicles are the sedans. However, the large vehicles contain cranes, buses, trucks, goods 21.
(27) wagons, etc. The Figure 1 displays intensity of back wave for the two vehicle classes on the lane 3. The 10 solid curves and 10 dashed curves represent the sample of small and large vehicles, individually. The functional data of different lanes and vehicles are shown in Figure 2 and Figure 3.. 10. Vehicle on Lane 3. 6 2. 4. Voltage. 8. Small Large. 0. 5. 10. 15. 20. 25. 30. Times (0.01024 sec). Figure 1: The intensity of back wave for the two vehicle classes on the lane 3. The 10 solid curves and 10 dashed curves represent the sample of small and large vehicles, individually.. 22.
(28) 6. Small Large. 2. Voltage. 10. Vehicle on Lane 1. 0. 5. 10. 15. 20. 25. 30. Times (0.01024 sec). 6. Small Large. 2. Voltage. 10. Vehicle on Lane 2. 0. 5. 10. 15. 20. 25. 30. Times (0.01024 sec) Figure 2: The intensity of back wave for the two vehicle classes on the lane 1 and lane 2. The 10 solid curves and 10 dashed curves represent the sample of small and large vehicles, individually.. 23.
(29) 6. Small Large. 2. Voltage. 10. Vehicle on Lane 3. 0. 5. 10. 15. 20. 25. 30. Times (0.01024 sec). 6. Small Large. 2. Voltage. 10. Vehicle on Lane 4. 0. 5. 10. 15. 20. 25. 30. Times (0.01024 sec) Figure 3: The intensity of back wave for the two vehicle classes on the lane 3 and lane 4. The 10 solid curves and 10 dashed curves represent the sample of small and large vehicles, individually.. 24.
(30) 3.2. Data Analysis. Our principal aim is to predict the class of vehicle and lane relative to the new observed functional data. In order to measure the performance of our functional nonparametric discrimination method, we set up two samples from the collected data sets. One is the learning sample, and the other is the testing sample. The learning sample allows us to estimate the posterior probabilities. The testing sample is useful for assessing the discriminant power. We can get the predicted groups of testing sample. The misclassification rate can be evaluated by the proportion of predicted groups not equal to the observed groups. Repeating this procedure 5000 times, we will get 5000 misclassification rates. The distribution of these misclassification rates can give a good idea of the power of nonparametric discrimination of functional data with various semi-metrics. Three types of semi-metrics are considered, and each of them has different forms being used. The different forms of three proximity measures are as follows: (i) PLS-type semi-metrics with a number of factors taking its values in 2, 3, 4, 5, 6, 7, 8 and 9 successively. (ii) PCA-type semi-metrics with a number of components taking its values in 2, 3, 4, 5, 6, 7 and 8 successively. (iii) Derivative-type semi-metrics with a number of derivatives equal to zero (classical L2 -norm).. 25.
(31) We first consider the recognition of traveling vehicles with fixed lane and speed. In order to measure the performance of our functional nonparametric discrimination method, we build randomly two samples from the original dataset. The learning sample and testing sample have 18 functional observations for each of classes of vehicles. Repeating the procedure 5000 times, we will get 5000 misclassification rates. The Figure 4 displays the box-plot of misclassification rates for three types of semi-metrics proximity. The Figure 5 displays the scatter plot of mean and variance of misclassification rates for three types of semi-metrics proximity. Our goal is to predict the classes of traveling vehicles correctly. So we hope to find the robust methodology with lower misclassification rate. From the Figure 4 and Figure 5 we prefer PLS-type semi-metrics with 2 factors and PCA-type semi-metrics with 3 components to the others. The proportion that we can correctly recognize the class of traveling vehicle is about 84%. The Figure 6 displays the classification rate over 5000 runs with 2 classes of vehicles. The columns denote the real classes and the rows denote the predicted classes. The correct classification rates and misclassification rates are both exhibited in the Figure 6. The diagonal rates denote the correct classification rate over the 5000 runs. Others denote the misclassification rate over the 5000 runs.. 26.
(32) 0.5 0.4 0.3 0.2 0.0. 0.1. MISCLSSIFICATION RATES. plsr2. plsr4. plsr6. plsr8. pca2. pca4. pca6. pca8. SEMI−METRICS. Figure 4: Dynamic vehicle recognition data discrimination over 5000 runs with 2 classes of vehicles.. 27.
(33) plsr9. plsr7. 0.26. plsr6. plsr5. 0.24. plsr4. 0.20. 0.22. plsr3. 0.16. 0.18. Mean of Misclassification Rate. 0.28. plsr8. pca8. pca6 pca5 pca7. deriv0 pca4 pca2 pca3. plsr2. 0.0020. 0.0025. 0.0030. 0.0035. 0.0040. 0.0045. 0.0050. 0.0055. Var of Misclassification Rate. Figure 5: Scatter plot of mean and variance of misclassification rate over 5000 runs with 2 classes of vehicles.. 28.
(34) Figure 6: The classification rate over 5000 runs with 2 classes of vehicles. The columns denote the real classes and the rows denote the predicted classes.. 29.
(35) Our second aim is to classify the lanes and dynamic vehicles with fixed speed. In order to measure the performance of our functional nonparametric discrimination method, we also build randomly two samples from the original dataset. The numbers of functional observations of small vehicles on lane 1, 2, 3 and 4 take its value in 10, 10, 10 and 10. Then the numbers of functional observations of large vehicles on lane 1, 2, 3 and 4 take its value in 10, 10, 10 and 5. The size of learning sample and testing sample are the same. We also repeat 5000 times above procedure to get 5000 misclassification rates. The Figure 7 and 8 are analogous to the Figure 4 and Figure 5. We tend to regard PLS-type semi-metrics with 2 or 3 factors as the proximity for these data. The correct classification rate is about 42%, a dramatic drop due to increasing in number of classes. The Figure 9 displays the classification rate over 5000 runs with 8 classes of vehicles and lanes. The columns denote the real classes and the rows denote the predicted classes. The correct classification rates and misclassification rates are both exhibited in the Figure 9. The diagonal rates denote the correct classification rate over the 5000 runs. Others denote the misclassification rate over the 5000 runs.. 30.
(36) 0.8 0.7 0.6 0.4. 0.5. Misclassification Rates. plsr2. plsr4. plsr6. plsr8. pca2. pca4. pca6. pca8. Semi−Metrics. Figure 7: Dynamic vehicle recognition data discrimination over 5000 runs with 8 classes of vehicles and lanes.. 31.
(37) 0.66. plsr9. deriv0 plsr8. 0.64. plsr6. pca6 pca8. plsr5. pca2. pca7 pca5. 0.62. pca3. pca4. plsr4. plsr3. 0.60. Mean of Misclassification Rate. plsr7. plsr2. 0.0022. 0.0024. 0.0026. 0.0028. Var of Misclassification Rate. Figure 8: Scatter plot of mean and variance of misclassification rate over 5000 runs with 8 classes of vehicles and lanes.. 32.
(38) Figure 9: The classification rate over 5000 runs with 8 classes of vehicles and lanes. The columns denote the real classes and the rows denote the predicted classes.. 33.
(39) 4. Conclusion This research uses three semi-metrics proximity to deal with nonparametric dis-. crimination of functional data. The result shows PLS-type semi-metrics proximity is more appropriate for the Radar microwave data set of the recognition of traveling vehicles. The vehicles functional data can be treated as multivariate data with 30 predictors, then PCA and PLS methodology implement data reduction with 2 or 3 dimension. But their recognition rate is higher than that with more factors. The large vehicles contain cranes, buses, trucks, goods wagons, etc. These vehicles have distinct forms, but we classify them analog. This may cause the recognition rate to be not as high as we expect. No matter how we expect that the nonparametric discrimination of functional data with these three semi-metrics proximity contribute to improve traffic jam and build the lane for a special purpose.. 34.
(40) References [1] Boor, C. A. (1978). A practical guide to splines. Springer, New York. [2] Dauxois, J., Pousse, A., and Romain, Y. (1982) Asymptotic theory for the principal component analysis of a random vector function: some application to statistical inference. Journal of Multivariate analysis, 12, 136-154. [3] Ferraty, F. and Vieu, P. (2006). Nonparametric functional data analysis. New York: Springer. [4] Frank, I.E., and Friedman, J.H. (1993). A statistical view of chemometrics regression tools. Technometrics, 35, 109-148. [5] Geladi,P., and Kowlaski, B. (1986). Partial least square regression: A tutorial. Analytica Chemica Acta, 35, 1-17. [6] Helland, I.S. (1990). PLS regression and statistical models. Scandivian Journal of Statistics, 17, 97-114. [7] Herman, S. (2002). A Particle Filtering Approach to Joint Passive Radar Trucking and Target Classification, Doctoral Dissertation, Department of Electrical and Computer Engineering, Univ. of Illinois at Urbana-Champaign, Urbana, IL.. 35.
(41) [8] Herman, S. and Moulin P. (2002). A particle filtering approach to joint radar tracking and automatic target recognition. in Proc. IEEE Aerospace Conference, (Big Sky, Montana), March 10-15. [9] Hoskuldsson, P. (1988). PLS regression methods. Journal of Chemometrics, 2, 211-288. [10] Jong, S. (1993) SIMPLS: an alternative approach to partial least squares regression. chemometrics and Intelligent Laboratory Systems, 18, 251-263. [11] Ramsay, J., and Silverman, B. (1997). Functional data analysis. New York: Springer. [12] Tenenhaus, M. (1998). La regrsssion PLS. Paris: Technip. [13] Wold, H. (1966). Estimation of principal components and related models by iterative least squares. In P.R. Krishnaiaah (Ed.). Multivariate Analysis, 391420. New York: Academic Press. [14] Wold, H. (1975). Path models with latent variables: The NIPALS approach. In H.M. Blalock et al., editor, Quantitative sociology: international perspectives on mathematical and statistical model building, 307-357. New York: Academic Press.. 36.
(42)
數據
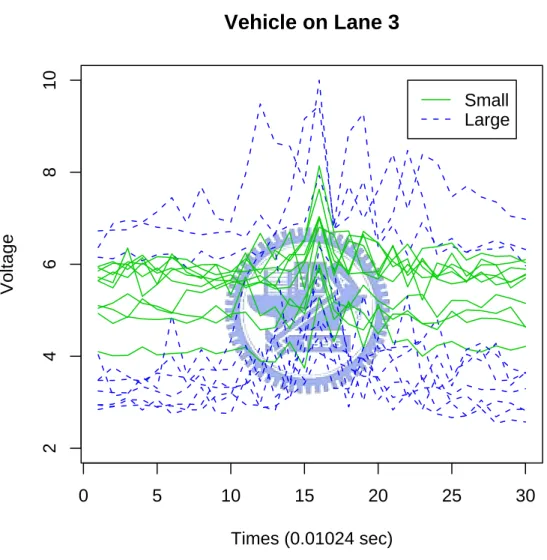

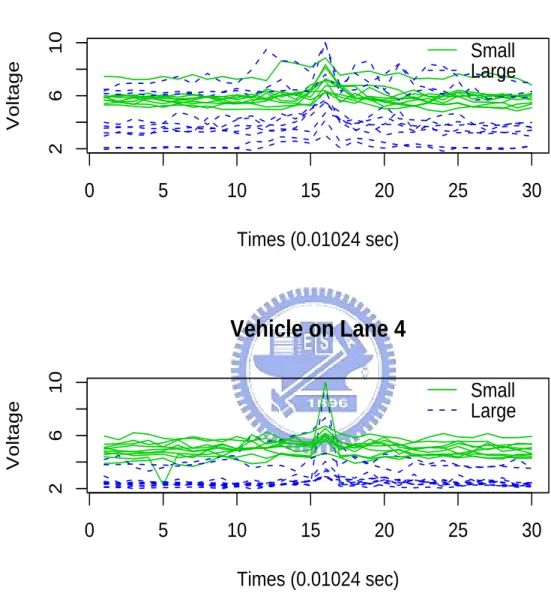
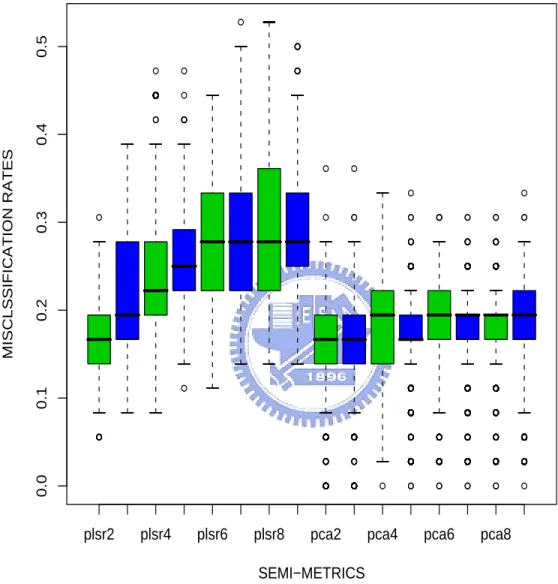
相關文件
The prototype consists of four major modules, including the module for image processing, the module for license plate region identification, the module for character extraction,
mathematical statistics, statistical methods, regression, survival data analysis, categorical data analysis, multivariate statistical methods, experimental design.
In this paper, we extend this class of merit functions to the second-order cone complementarity problem (SOCCP) and show analogous properties as in NCP and SDCP cases.. In addition,
automated cartography 自動製圖 automated data processing 自動數據處理 automated digitising 自動數碼化 automated feature recognition 自動要素識別 automated
Sexual Abuse of Children with Autism: Factors that Increase Risk and Interfere with Recognition of Abuse.... ASD –
“Big data is high-volume, high-velocity and high-variety information assets that demand cost-effective, innovative forms of information processing for enhanced?. insight and
Our main goal is to give a much simpler and completely self-contained proof of the decidability of satisfiability of the two-variable logic over data words.. We do it for the case
Good Data Structure Needs Proper Accessing Algorithms: get, insert. rule of thumb for speed: often-get