多輸入多輸出無線通信頻道之盲判別
全文
(2) 多輸入多輸出無線通信頻道之盲判別 Blind Identification for Multiple-input Multiple-output Wireless Communication Channels. 研 究 生:陳益生. Student:Yi-Sheng Chen. 指導教授:林清安. Advisor:Ching-An Lin. 國 立 交 通 大 學 電 機 與 控 制 工 程 學 系 博 士 論 文. A Dissertation Submitted to Department of Electrical and Control Engineering College of Electrical and Computer Engineering National Chiao Tung University in Partial Fulfillment of the Requirements for the Degree of Doctor of Philosophy in Electrical and Control Engineering December 2006 Hsinchu, Taiwan, Republic of China. 中華民國九十五年十二月.
(3) 多輸入多輸出無線通信頻道之盲判別 研究生:陳益生. 指導教授:林清安. 國立交通大學電機與控制工程學系. 中文摘要 本論文針對多輸入多輸出頻率選擇衰減之無線通信頻道,利用不 同的編碼方式,提出了三種通道盲蔽判別的方法。判別的方法是利用 所估測出來的接收信號協方差矩陣,先計算出通道乘積矩陣,再對一 個由通道乘積矩陣所形成的厄米特矩陣取特徵分解,即可求出通道脈 衝響應矩陣。我們分別考慮了以下三種不同的編碼方式: (1) 週期性 編碼, (2) 週期性編碼加補零 ,(3) 補零。 對應於上述三種編碼 方式,通道乘積矩陣可分別由: (1) 一系列經解耦過的過定線性系統 方程組求得, (2) 一個下三角線性系統方程式求得,(3) 執行一些 簡單的減法所求得。對於前兩種判別方法,我們也設計了相對應的最 佳週期編碼序列來減少通道雜訊以及數值誤差的影響,以提高通道估 測的準確度。與子空間的方法比較起來,我們所提出的方法較為簡 單,同時判別條件也較為寬鬆。此外我們的方法還可適用於發射器數 目多於、少於、或等於接收器數目的情況。數值模擬的結果顯示我們 所提出的方法對通道階數過估的情況具有相當的強健性。. i.
(4) Blind Identification for Multiple-input Multiple-output Wireless Communication Channels Student: Yi-Sheng Chen. Advisor: Ching-An Lin. Department of Electrical and Control Engineering National Chiao-Tung University. Abstract We propose three blind identification algorithms for multiple-input multiple-output (MIMO) frequency selective fading wireless communication channels based on different precoding. The algorithms compute the channel product matrices from the estimated covariance matrix of the received data and then determine the channel impulse response matrix via an eigen-decomposition. Three precoding are considered: (i) periodic precoding, (ii) periodic precoding plus zero padding, and (iii) zero padding alone. As a result, for each of the three cases, the computation required to determine the channel product matrices are also different. The computations required are respectively (i) to solve a decoupled group of overdetermined linear systems of equations, (ii) to solve a lower triangular linear system, and (iii) to carry out a number of simple subtractions. For the first two algorithms, we also discuss the optimal design of the precoding sequences to minimize the effects of additive channel noise and numerical error, so as to increase the accuracy of channel estimation. The algorithms are simple, in terms of the amount of computations required, as compared with subspace methods; they allow a more relaxed identifiability condition and are applicable to MIMO channels with more transmitters or more receivers. Simulation results show that they are reasonably robust with respect to channel order overestimation.. ii.
(5) 致. 謝. 本論文能順利完成,首先要感謝我的指導教授林清安老師的悉心 指導,老師嚴謹的治學態度、簡潔清楚的論文寫作風格,以及堅持學 術真理的精神,可謂惠我良多,凡此種種,都足堪為益生的楷模與表 率。 此外,我也要感謝論文口試委員清大通訊所祁忠勇教授、王晉良 教授、交大電控系林源倍教授、鄭木火教授,以及交大電信系吳卓諭 研究員對本論文所提供的寶貴意見,使得本論文能夠更臻完善。 實驗室的同學們總是能在枯燥的研究生活中帶來一些調劑,建 賢、敬淵、炅嶔、正哲、文達、瑞華、江龍、建宇、傑智、育霖、威 德、哲嘉,這研究所同窗之誼,永難磨滅。 家人永遠能給我最溫暖的支持,特別是我的父母,當初若沒他們 的鼓勵與經濟上的支持,我恐怕也不會來讀博士班。此外,也容我無 法一一點名召喚所有值得感謝的人,但你們知道,我是很謝謝你們的。 四年多來的博士班生涯即將隨著這本博士論文的誕生而進入尾 聲。回首過去這段在交大的日子,是我人生中學習、成長、與進步最 快的時光,生活總是在修課與研究當中過去,日子雖然忙碌,但卻過 得相當充實而有意義。願我年老之際,再次翻開這本論文時,還能重 拾起這段美好歷程的點點滴滴。. iii.
(6) Contents Chinese Abstract . . . . . . . . . . . . . . . . . . . . . . . . . . . . . . . . . .. i. Abstract . . . . . . . . . . . . . . . . . . . . . . . . . . . . . . . . . . . . . . .. ii. Acknowledge . . . . . . . . . . . . . . . . . . . . . . . . . . . . . . . . . . . .. iii. 1 Introduction. 1. 1.1. Research Objective . . . . . . . . . . . . . . . . . . . . . . . . . . . . . . .. 1. 1.2. Literature Survey . . . . . . . . . . . . . . . . . . . . . . . . . . . . . . . .. 2. 1.3. Organization of the Dissertation . . . . . . . . . . . . . . . . . . . . . . . .. 3. 2 Blind Identification for MIMO FIR Channels. 5. 2.1. System Model and Formulation . . . . . . . . . . . . . . . . . . . . . . . .. 6. 2.2. Blind Channel Identification . . . . . . . . . . . . . . . . . . . . . . . . . .. 7. 2.2.1. The Identification Method . . . . . . . . . . . . . . . . . . . . . . .. 8. 2.2.2. Channel Order Overestimation. . . . . . . . . . . . . . . . . . . . .. 11. 2.2.3. More Transmitters Than Receivers . . . . . . . . . . . . . . . . . .. 12. Optimal Design of the Precoding Sequence . . . . . . . . . . . . . . . . . .. 12. 2.3.1. Optimality Criterion . . . . . . . . . . . . . . . . . . . . . . . . . .. 13. 2.3.2. On Selection of m . . . . . . . . . . . . . . . . . . . . . . . . . . . .. 15. 2.4. Identification Algorithm . . . . . . . . . . . . . . . . . . . . . . . . . . . .. 16. 2.5. Simulation Results . . . . . . . . . . . . . . . . . . . . . . . . . . . . . . .. 17. 2.6. Summary . . . . . . . . . . . . . . . . . . . . . . . . . . . . . . . . . . . .. 21. 2.3. 3 Blind Channel Identification for MIMO Single Carrier Zero Padding Block Transmission Systems. 26. 3.1. 27. System Model and Formulation . . . . . . . . . . . . . . . . . . . . . . . .. iv.
(7) 3.2. Blind Channel Identification . . . . . . . . . . . . . . . . . . . . . . . . . .. 28. 3.2.1. The Identification Method . . . . . . . . . . . . . . . . . . . . . . .. 29. 3.2.2. Channel Order Overestimation. . . . . . . . . . . . . . . . . . . . .. 33. 3.2.3. More Transmitters Than Receivers . . . . . . . . . . . . . . . . . .. 33. 3.2.4. Optimal Design of the Precoding Sequence . . . . . . . . . . . . . .. 34. 3.2.5. . . . . . . . . . . . . . . . . . . . . . . . . . . Computation of G−1 0. 37. 3.2.6. Identification Algorithm . . . . . . . . . . . . . . . . . . . . . . . .. 37. 3.3. Channel Equalization . . . . . . . . . . . . . . . . . . . . . . . . . . . . . .. 38. 3.4. Simulation Results . . . . . . . . . . . . . . . . . . . . . . . . . . . . . . .. 39. 3.5. Summary . . . . . . . . . . . . . . . . . . . . . . . . . . . . . . . . . . . .. 42. 4 Blind Channel Identification for MIMO Zero Padding Block Transmission Systems. 48. 4.1. System Model and Formulation . . . . . . . . . . . . . . . . . . . . . . . .. 49. 4.2. Blind Channel Identification . . . . . . . . . . . . . . . . . . . . . . . . . .. 50. 4.2.1. The Identification Method . . . . . . . . . . . . . . . . . . . . . . .. 51. 4.2.2. Identification Algorithm . . . . . . . . . . . . . . . . . . . . . . . .. 54. 4.2.3. Extension to MIMO Zero-Padding OFDM Systems . . . . . . . . .. 55. 4.3. Simulation Results . . . . . . . . . . . . . . . . . . . . . . . . . . . . . . .. 56. 4.4. Summary . . . . . . . . . . . . . . . . . . . . . . . . . . . . . . . . . . . .. 59. 5 Conclusions. 64. Appendix. 65. A. Proof of Proposition 2.2 and 2.3 . . . . . . . . . . . . . . . . . . . . . . . .. 65. B. The Eigenvalues of NTj Nj for m = N − L . . . . . . . . . . . . . . . . . . .. 67. C. Optimal Precoding Sequence for L = 1 . . . . . . . . . . . . . . . . . . . .. 68. D. A Proof of. >0. . . . . . . . . . . . . . . . . . . . . . . . . . . . . .. 69. E. A Proof of Proposition 3.1 . . . . . . . . . . . . . . . . . . . . . . . . . . .. 70. ∂f (α,β) ∂α. Biblography. 71. Vita and Publication List. 75. v.
(8) List of Figures 2.1. An MIMO channel model . . . . . . . . . . . . . . . . . . . . . . . . . . .. 6. 2.2. Channel NRMSE versus number of samples . . . . . . . . . . . . . . . . .. 22. 2.3. Channel NRMSE versus output SNR . . . . . . . . . . . . . . . . . . . . .. 22. 2.4. ˆ − L) . . . . . . . . . . . . . . . . . . . . . . . . Channel NRMSE versus (L. 23. 2.5. 3-input 2-output model: channel NRMSE versus number of samples . . . .. 23. 2.6. 3-input 2-output model: channel NRMSE versus output SNR . . . . . . . .. 24. 2.7. Symbol error rate versus output SNR . . . . . . . . . . . . . . . . . . . . .. 24. 2.8. Comparison of NRMSE and symbol error rate, number of input samples = 1200 . . . . . . . . . . . . . . . . . . . . . . . . . . . . . . . . . . . . . . .. 2.9. 25. Comparison of NRMSE and symbol error rate, number of input samples = 200 . . . . . . . . . . . . . . . . . . . . . . . . . . . . . . . . . . . . . . . .. 25. 3.1. An MIMO SC-ZP block transmission baseband model with periodic precoding 27. 3.2. Channel NRMSE versus number of symbol blocks . . . . . . . . . . . . . .. 43. 3.3. Channel NRMSE versus output SNR . . . . . . . . . . . . . . . . . . . . .. 43. 3.4. ˆ − L) . . . . . . . . . . . . . . . . . . . . . . . . Channel NRMSE versus (L. 44. 3.5. Channel NRMSE versus number of symbol blocks . . . . . . . . . . . . . .. 44. 3.6. Channel NRMSE versus output SNR . . . . . . . . . . . . . . . . . . . . .. 45. 3.7. Channel NRMSE versus output SNR . . . . . . . . . . . . . . . . . . . . .. 45. 3.8. BER versus output SNR . . . . . . . . . . . . . . . . . . . . . . . . . . . .. 46. 3.9. Comparison with the subspace method . . . . . . . . . . . . . . . . . . . .. 46. 3.10 Channel NRMSE versus number of symbol blocks . . . . . . . . . . . . . .. 47. 4.1. An MIMO SC-ZP block transmission baseband model . . . . . . . . . . . .. 49. 4.2. An MIMO ZP-OFDM baseband model . . . . . . . . . . . . . . . . . . . .. 56. vi.
(9) 4.3. Color noise case . . . . . . . . . . . . . . . . . . . . . . . . . . . . . . . . .. 60. 4.4. Channel NRMSE versus output SNR . . . . . . . . . . . . . . . . . . . . .. 60. 4.5. Channel NRMSE versus output SNR . . . . . . . . . . . . . . . . . . . . .. 61. 4.6. ˆ − L) . . . . . . . . . . . . . . . . . . . . . . . . Channel NRMSE versus (L. 61. 4.7. An OFDM system case . . . . . . . . . . . . . . . . . . . . . . . . . . . . .. 62. 4.8. An OFDM system: symbol error rate versus output SNR . . . . . . . . . .. 62. 4.9. An OFDM system: symbol error rate versus output SNR . . . . . . . . . .. 63. vii.
(10) Chapter 1 Introduction. 1.1. Research Objective. Multiple-input multiple-output (MIMO) communication systems employing multiple transmit and receive antennas have received much attention due to the potential improvement in data transmission rate and link reliability they can offer. However, to exploit the potential advantage of MIMO systems, accurate channel state information is required. Channel can be identified or estimated using training signal which requires additional bandwidth. As a means to eschewing the need of training signal and the associated bandwidth requirement, blind identification of MIMO channels has been the focus of much research. Many blind identification algorithms have been proposed in recent years (see [1, 2] for a detailed review). Existing algorithms for blind identification of MIMO finite impulse response (FIR) channels can be classified into second-order statistics methods [8]-[12],[20]-[22], higherorder statistics methods [3]-[5], and deterministic methods [6, 7]. Among these three types of methods, blind identification based on second-order statistics has been widely studied because it requires fewer data samples than the high-order statistics approach and it avoids poor estimation accuracy under low SNR, a common shortcoming of deterministic methods. Existing second-order statistics methods for MIMO systems, e.g., the subspace methods. 1.
(11) [8, 9], [27], [29]-[30], the linear prediction methods [10]-[12], and the matrix outer product decomposition methods [20]-[22], either impose restrictive assumptions on the channel to be identified or require large amount of computations, that may not be realistic in practical applications. The goal of this research is to develop blind identification algorithms for MIMO channels, that are simple in computation and less restrictive in assumptions. It is hoped that the algorithms developed are thus more practical from an application point of view.. 1.2. Literature Survey. It is well-known that cyclostationarity of the received data is the key to all blind identification based on second-order statistics [1, 2]. Cycloststionarity can be induced either at the receiver, by oversampling or multiple antennas, or at the transmitter, by various coding methods. An advantage of transmitter-induced cyclostationarity is that the resulting identification methods require less restrictive assumption on the channel, for example, channels with nonminimum phase zeros can be handled. One effective way to induce cyclostationarity at the transmitter is by periodic precoding. Blind identification methods for general MIMO FIR channels using periodic precoding are found in [17, 18]. In [17], Chevreuil and Loubaton proposes a scheme that multiplies the input sequence by a constant modulus complex exponential precoding sequence to induce conjugate cyclostationarity at the transmitter. The scheme reduces the MIMO channel identification problem to several SIMO ones, which are then solved by the subspace method [24]. Each SIMO channel is required to be free from common zeros. However, the method in [17] allows only real input symbols and the identifiability condition is irreducible and column reduced. B¨olcskei et. al. [18] proposes a method for identifying each of the scalar channels individually up to a phase ambiguity using non-constant modulus periodic precoding sequences. The method imposes no restriction on channel zeros and is insensitivity to channel order overestimation. However, no systematic procedure for the design of the precoding sequences is given. In this dissertation, we propose an identification method based on periodic precoding, which allows complex input symbols and gives an optimal design of the precoding sequence. 2.
(12) Single carrier zero padding (SC-ZP) block transmission systems, another communication systems, are used to remove interblock interference (IBI) [13, 14, 25, 27]. In the literature, to the best of our knowledge, there is only one paper, by Zeng and Ng [27], that proposes a subspace method for blind identification of MIMO SC-ZP block transmission systems. The method can be used to identify the channel impulse response matrix up to a matrix ambiguity when the channel is irreducible and the channel noise is uncorrelated and white. In this dissertation, we first propose an identification method for MIMO SC-ZP systems based on periodic precoding, which can further relax the identifiability condition and reduce the computational load, compared with the method in [27]. In addition, we also propose another simplified identification method for such systems without periodic precoding. This simplified method can also apply to MIMO zero padding orthogonal frequency division multiplexing (ZP-OFDM) systems.. 1.3. Organization of the Dissertation. The dissertation is organized as follows. In Chapter 2, we propose a blind identification method for general MIMO FIR channels based on periodic precoding. We also discuss the optimal design of the precoding sequence which takes into account the effect of additive channel noise and numerical error. We also propose a blind identification method for MIMO FIR channels in SC-ZP block transmission systems based on periodic precoding and discuss the optimal design of the precoding sequence in Chapter 3. In Chapter 4, we first propose a blind identification for SC-ZP block transmission systems without periodic precoding. Extension of this method to ZP-OFDM systems is given subsequently. Chapter 5 concludes this dissertation and discusses the related future research. Notations used in this dissertation are quite standard: Bold uppercase is used for matrices, and bold lowercase is used for vectors. AT and A∗ denote the transpose and conjugate transpose of A, respectively. 0M ×N denotes the zero matrix of dimension M × N, and IM denotes the identity matrix of dimension M × M. A ⊗ B is the Kronecker product of A and B. 0M ×N is the zero matrix of dimension M × N. The symbols R and C stand for the set of real numbers and the set of complex numbers, respectively. In addition, we define 3.
(13) the following operations that will be used in the derivation of the main result. First, for any m × m matrix A = [ak,l ]0≤k,l≤m−1, define Γj (A) = [a0,j a1,j+1 · · · am−1−j,m−1 ]T for 0 ≤ j ≤ m − 1, i.e., Γj (A) is the vector formed from the jth super-diagonal of A. Second, for any Jn×Jn matrix B = [Bk,l ]0≤k,l≤n−1, where Bk,l is a block matrix of dimension J ×J, define Υj (B) = [BT0,j BT1,j+1 · · · BTn−1−j,n−1]T for 0 ≤ j ≤ n − 1, i.e., Υj (B) is the matrix formed from the jth block super-diagonal of B.. 4.
(14) Chapter 2 Blind Identification for MIMO FIR Channels In this chapter, we propose a blind identification method for MIMO FIR channels based on periodic precoding. It is shown that, by properly choosing the precoding sequence, the MIMO FIR channels, with K transmitters and J receivers, can be identified up to a unitary matrix ambiguity. The main solution procedure contains two steps: (1) solve the channel product matrices from a series of linear equations obtained from the covariance matrix of the received data, and (2) take eigen-decomposition of a Hermitian matrix formed from those channel product matrices to estimate the channel. The channel identifiability condition needs not be irreducible or column reduced [21], and there can be more receivers (J ≥ K) or more transmitters (J < K). The performance of the algorithm, and indeed the identifiability, depends on the choice of the precoding sequence. We propose a method for optimal selection of the precoding sequence which takes into account the effects of additive channel noise and numerical error in covariance matrix estimation. Simulation results show that the algorithm are reasonably robust with respect to channel order overestimation.. 5.
(15) p(n) w1 (n) ? u1 (n) ? x1 (n) s1 (n)- t1 (n) - MIMO FIR .. . .. .. . . p(n) . wJ (n) .. ? uK (n) Channel ? xJ (n) sK (n)- tJ (n) - Figure 2.1. An MIMO channel model. 2.1. System Model and Formulation. We consider the linear MIMO baseband model of a communication channel with K transmitters and J receivers shown in Figure 2.1, where each source symbol sequence is multiplied by a P -periodic sequence, p(n), before transmission. The transmitted signal is uk (n) = p(n)sk (n),. k = 1, 2, · · · , K,. (2.1). where p(n + P ) = p(n), ∀ n. The discrete time model describing the relation between the transmitted signal uk (n) and the received signal xj (n) has the form of an MIMO FIR filter with additive noise: xj (n) =. Ljk K . hjk (l)uk (n − l) + wj (n),. j = 1, 2, · · · , J,. (2.2). k=1 l=0. where hjk (l), l = 1, 2, · · · , Ljk , is the impulse response of the channel between the kth transmitter and the jth receiver, and wj (n) is the channel noise seen at the input of the jth receiver. If we define u(n) = [u1 (n) u2 (n) · · · uK (n)]T , s(n) = [s1 (n) s2 (n) · · · sK (n)]T , x(n) = [x1 (n) x2 (n) · · · xJ (n)]T , and w(n) = [w1 (n) w2 (n) · · · wJ (n)]T , then the equations (2.1) and (2.2) can be written more compactly as u(n) = p(n)s(n),. x(n) =. L . H(l)u(n − l) + w(n),. (2.3). l=0. where H(l) ∈ CJ×K is the channel coefficient matrix whose jkth element is hjk (l), and L = maxj,k {Ljk } is the order of the MIMO channel. We assume that H(L) = 0J×K . Group the sequence of x(n) as x ¯(n) = [x(P n)T , x(P n + 1)T , · · · , x(P n + P − 1)T ]T ∈ CJP , and let w(n), ¯ u ¯(n), ¯ s(n) be similarly defined, we have ¯(n) + H1 u ¯(n − 1) + w(n), ¯ x ¯(n) = H0 u 6. (2.4).
(16) where H0 is an JP × KP block lower-triangular Toeplitz matrix with [H(0)T H(1)T · · · H(L)T 0TJ×K · · · 0TJ×K ]T ∈ CJP ×K as its first block column (i.e., the first K columns), and H1 is an JP × KP block upper-triangular Toeplitz matrix with [0J×K · · · 0J×K H(L) H(L − 1) · · · H(1)] ∈ CJ×KP as its first block row (i.e., the first J rows). Since p(n) is periodic, u ¯(n) = G¯ s(n) for all n, where G = diag[p(0)IK , p(1)IK , · · · , p(P − 1)IK ] ∈ RKP ×KP is a diagonal matrix. Then (2.4) can be written as x ¯(n) = H0 G¯ s(n) + H1 G¯ s(n − 1) + w(n), ¯. (2.5). We assume that the receivers are synchronized with the transmitters. In addition, the following assumptions are made throughout this chapter.. (A1) s(n) and w(n) are white zero-mean vector sequences, and s(n) and w(n) are temporally and spatially uncorrelated. More precisely, E[s(k)s(j)∗ ] = δ(k − j)IK ∈ RK×K , E[w(k)w(j)∗] = δ(k − j)σw2 IJ ∈ RJ×J , E[s(k)w(j)∗ ] = 0K×J , ∀ k, j, where δ(·) is the Kronecker delta function. ˆ of the channel order L is known and the period P > L ˆ + 1. (A2) An upper bound L (A3) The channel impulse response matrix H = [H(0)T H(1)T · · · H(L)T ]T is full column rank, i.e., rank(H)=K.. In the next section, we will derive an algorithm for blind identification of the MIMO channel impulse response matrix H using second-order statistics of the received data.. 2.2. Blind Channel Identification. In this section, we derive the proposed method for the case under assumptions (A1), (A2), (A3) and noiseless case. We show that by appropriately selecting the periodic precoding sequence, any MIMO channel satisfying (A3) is identifiable up to an K × K unitary matrix ambiguity. The effect of noise and optimal design of the precoding sequence are discussed in Section 2.3. 7.
(17) 2.2.1. The Identification Method. We first derive the proposed method for the case where the channel order L is known with P > L + 1, there are more receivers, i.e., J ≥ K, and the noise is absent. We discuss the cases of channel order overestimation and more transmitters than receivers (i.e., K > J) in Section 2.2.2 and 2.2.3, respectively. From (2.5) and assumption (A1), the covariance matrix of x ¯(n) can be written as (noiseless case) x(n)¯ x(n)∗ ] = H0 G2 H∗0 + H1 G2 H∗1 . Rx¯ = E[¯. (2.6). Let J ∈ RP ×P be the matrix whose first sub-diagonal are all one and all remaining entries are zero, i.e.,. ⎡ ⎢ ⎢ ⎢ ⎢ ⎢ J=⎢ ⎢ ⎢ ⎢ ⎣. 1 0 .. .. ⎤. ···. 0. ··· .. . 1 .. . ···. 0 .. .. 0. 0. 0. 0. 0. 0. 0 .. . . .. . . .. ···. 1. ⎥ ⎥ ⎥ ⎥ ⎥ ⎥ ∈ RP ×P . ⎥ ⎥ ⎥ ⎦. 0. Then the block Toeplitz structures of H0 and H1 allow us to write H0 = Lk=0 Jk ⊗ H(k). and H1 = Lk=0 (JT )P −k ⊗H(k), respectively. In addition, we define Gp = diag[p(0), p(1), · · · , p(P − 1)] ∈ RP ×P . Hence H0 G2 H∗0 can be written as
(18) L l 2
(19) ∗ k J J ⊗ H(k) G ⊗ I ⊗ H(l) K p k=0 l=0
(20)
(21)
(22) 2. L L k = Gp ⊗ IK (JT )l ⊗ H(l)∗ k=0 l=0 J ⊗ H(k). L L k 2 T l
(23) ∗ = k=0 l=0 J Gp (J ) ⊗ (H(k)H(l) ) ,. H0 G2 H∗0 =. L. (2.7). where we have used the identies (A ⊗ B)∗ = A∗ ⊗ B∗ and (A ⊗ B)(C ⊗ D) = (AC) ⊗ (BD) [36, p.190]. Similarly, H1 G2 H∗1 can be written as H1 G2 H∗1 =. L L .
(24) (JT )P −k G2p JP −l ⊗ (H(k)H(l)∗ ) .. (2.8). k=0 l=0. The following proposition shows that the matrices Jk G2p (JT )l and (JT )P −k G2p JP −l have special structures that allow decomposition of (2.6) into a group of decoupled equations. 8.
(25) Roughly speaking, the jth block super-diagonal part of (2.6) involves only the unknown “channel product matrices”, H(k)H(k+j)∗, k = 0, 1, · · · , L−j. For example, the equations corresponding to the diagonal blocks (j = 0) involve only H(k)H(k)∗ , k = 0, 1, · · · , L. In the proposed identification algorithm, these “channel product matrices” are computed first by solving linear equations, and then the channel impulse response matrix H is computed via eigenvalue-eigenvector decomposition. Proposition 2.1 : Let 0 ≤ k, l ≤ L be two non-negative integers. Then (a) For l = k + j, where 0 ≤ j ≤ L − k, both Jk G2p (JT )l and (JT )P −k G2p JP −l are upper triangular matrices with only the respective jth upper diagonals nonzero, and.
(26) · · 0 p(0)2 p(1)2 · · · p(P − 1 − k − j)2 ]T , Γj Jk G2p (JT )l = [0 ·. k entries. Γj (JT )P −k G2p J.
(27) P −l. (2.9). P −k−j entries. = [p(P − k)2 p(P − k + 1)2 · · · p(P − 1)2 0 · · · 0 ]T .. k entries. (2.10). P −k−j entries.
(28).
(29) (b) For l < k, both Γj Jk G2p (JT )l and Γj (JT )P −k G2p JP −l are lower triangular with zero diagonal matrices. Proof : See [16]. It follows from (2.9) and (2.10) that.
(30).
(31) Γj Jk G2p (JT )l + Γj (JT )P −k G2p JP −l ⎧ ⎪ ⎪ − k)2 · · · p(P − 1)2 p(0)2 · · · p(P − 1 − k − j)2 ]T ⎨ [p(P. = k entries P −k−j entries ⎪ ⎪ ⎩ 0 if j = l − k (P −j)×1. if. j =l−k ≥0 .. (2.11) Since Υj. .
(32)
(33).
(34) Jk G2p (JT )l ⊗ H(k)H(l)∗ = Γj Jk G2p (JT )l ⊗ H(k)H(l)∗. (2.12). and Υj. .
(35)
(36).
(37) (JT )P −k G2p JP −l ⊗ H(k)H(l)∗ = Γj (JT )P −k G2p JP −l ⊗ H(k)H(l)∗ ,. 9. (2.13).
(38) it follows from (2.6)-(2.8) and (2.11)-(2.13) that Υj (Rx¯ ) can be derived as follows. Υj (Rx¯ ).
(39) = Υj H0 G2 H∗0 + H1 G2 H∗1
(40)
(41)
(42)
(43). = Lk=0 Ll=0 Υj Jk G2p (JT )l ⊗ (H(k)H(l)∗ ) + Υj (JT )P −k G2p JP −l ⊗ (H(k)H(l)∗ ).
(44).
(45). = Lk=0 Ll=0 {Γj Jk G2p (JT )l + Γj (JT )P −k G2p JP −l } ⊗ H(k)H(l)∗. L−j = k=0 [p(P − k)2 · · · p(P − 1)2 p(0)2 · · · p(P − 1 − k − j)2 ]T ⊗ H(k)H(k + j)∗. L−j = k=0 [p(P − k)2 IJ · · · p(P − 1)2 IJ p(0)2 IJ · · · p(P − 1 − k − j)2 IJ ]T H(k)H(k + j)∗ (2.14) The right hand side of (2.14) is a linear combination of block columns with the channel product matrices, H(k)H(k + j)∗ , as coefficients. If we define, for 0 ≤ j ≤ L, Fj = [(H(0)H(j)∗)T (H(1)H(j + 1)∗ )T · · · (H(L − j)H(L)∗ )T ]T ∈ CJ(L−j+1)×J , (2.15) then (2.14) can be written in a more compact form as ∀ 0 ≤ j ≤ L,. Υj (Rx¯ ) = Mj Fj. where Mj ∈ RJ(P −j)×J(L−j+1) is defined as ⎡ p(P − 1)2 p(P − 2)2 p(0)2 ⎢ ⎢ ⎢ p(1)2 p(0)2 p(P − 1)2 ⎢ ⎢ ⎢ p(2)2 p(1)2 p(0)2 ⎢ .. .. .. ⎢ Mj = ⎢ . . . ⎢ ⎢ ⎢ p(P − 3 − j)2 p(P − 4 − j)2 p(P − 5 − j)2 ⎢ ⎢ ⎢ p(P − 2 − j)2 p(P − 3 − j)2 p(P − 4 − j)2 ⎣ p(P − 1 − j)2 p(P − 2 − j)2 p(P − 3 − j)2. ··· ··· ··· .. . ··· ··· ···. (2.16). ⎤. p(P − L + j)2. ⎥ ⎥ p(P − L + j + 1) ⎥ ⎥ ⎥ p(P − L + j + 2)2 ⎥ ⎥ .. ⎥ ⎥ ⊗ IJ . . ⎥ ⎥ 2 ⎥ p(P − L − 3) ⎥ ⎥ 2 ⎥ p(P − L − 2) ⎦ 2 p(P − L − 1) 2. (2.17) We note that Mj , 1 ≤ j ≤ L, is obtained from M0 by deleting its last jJ rows and last jJ columns. Since P > L + 1, the (L + 1) equations in (2.16) are overdetermined and for the noise free case, these equations are consistent. We note that the matrix Mj , j = 0, 1, · · · , L, is completely determined by the precoding sequence. By appropriately selecting the precoding 10.
(46) sequence, we can make each Mj full column rank. Then the solution Fj can be obtained as Fj = (MTj Mj )−1 MTj Υj (Rx¯ ) .. (2.18). If Fj , 0 ≤ j ≤ L, are computed from (2.18), then we have the channel product matrices H(k)H(l)∗ for 0 ≤ k ≤ l ≤ L. We now consider the computation required to determine the channel impulse response matrix H from Fj . Let Q be the Hermitian matrix defined by Υj (Q) = Fj for j = 0, 1, · · · , L, and we know the channel impulse response matrix H = [H(0)T H(1)T · · · H(L)T ]T . Clearly we have Q = HH∗ .. (2.19). Since rank(H) = K by assumption (A3), Q has rank K. Since Q is Hermitian and positive semidefinite, Q has K positive eigenvalues, say, λ1 , · · · , λK . We can expand Q as K Q= ( λj dj )( λj dj )∗ ,. (2.20). j=1. where dj is a unit norm eigenvector of Q associated with λj > 0. We can thus choose the channel impulse response matrix to be = [ λ1 d1 λ2 d2 · · · λK dK ] ∈ CJ(L+1)×K . H. (2.21). We note H can only be identified up to a unitary matrix ambiguity U ∈ CK×K [20, 21], = HU, since H H ∗ = HH∗ = Q. The ambiguity matrix U is intrinsic to methods i.e., H for blind identification of multiple input systems using only second-order statistics [20, 21]. This ambiguity U can be solved using a short training sequence.. 2.2.2. Channel Order Overestimation. So far we have assumed that the channel order L is known. If only an upper bound ˆ ≥ L is available with P > L ˆ + 1, then following the same process given in Section 2.2.1, L ˆ + 1) × J(L ˆ + 1) matrix Q can be similarly constructed as in (2.19). the corresponding J(L ˆ ˆ ˆ The last (L−L) block columns (i.e., (L−L)J columns) of Q are zero, so are its last (L−L) 11.
(47) block rows. Hence again, Q is of rank K and has K positive eigenvalues with the associated ˆ ˆ = [dT 0 · · · 0]T ∈ CJ(L+1) where d ∈ CJ(L+1) . Thus, we can eigenvectors all of the form d. determine the channel impulse response matrix, up to a unitary matrix ambiguity, from the K eigenvectors associated with the K positive eigenvalues of Q. In the noise free case, we can, in theory, also determine the actual channel order.. 2.2.3. More Transmitters Than Receivers. In the above discussions, we assume that there are more receivers than transmitters, i.e., J ≥ K. If there are more transmitters, i.e., K > J, then either J(L + 1) ≥ K or K > J(L + 1). If J(L + 1) ≥ K, then H is a tall matrix and assumption (A3) is generically satisfied [34]. Hence the proposed method still applies. If K > J(L+1), then rank(H) < K and assumption (A3) does not hold. Hence the proposed method is applicable to the more transmitters case, provided the additional condition J(L + 1) ≥ K is satisfied. We note that if the channel has more transmitters than receivers, channel equalization and source separation may be difficult even if accurate channel estimate is available. In addition, we note that in the proposed method, the channel impulse response matrix H is only assumed to be full column rank (A3). Hence the channel needs not be irreducible or column reduced [21].. 2.3. Optimal Design of the Precoding Sequence. In Section 2.2, we see that in order to identify the channel, the precoding sequence must be selected so that the resulting matrix Mj is full column rank such that Fj can be exactly solved as (2.18). However, when noise is present, the covariance matrix Rx¯ contains the contribution of noise and numerical error is present in the estimation of Rx¯ in practice. This implies that (2.16) usually has no solution and (2.18) becomes a least squares approximate solution. The choice of Mj will affect error in the computation of Fj since different MTj Mj in (2.18) usually have different condition numbers. In this section, we discuss the optimal design of the precoding sequence, which takes into account the effect 12.
(48) ˆ x¯ , so as to increase the accuracy of Fj and of noise and numerical error in estimating R thus reduce the channel estimation error.. 2.3.1. Optimality Criterion. Now we consider the general case that noise is present and discuss the design of the precoding sequence p(n). From (2.4) and assumption (A1), the covariance matrix of the received signal is Rx¯ = H0 G2 H∗0 + H1 G2 H∗1 + σw2 IJ ⊗ IP .. (2.22). From (2.22) and (2.6), we see that noise has only contribution to the diagonal entries of Rx¯ . Therefore the (L + 1) decoupled groups of equations in (2.16) remain unchanged, except for the j = 0 group, which becomes.
(49) Υ0 (Rx¯ ) = Υ0 H0 G2 H∗0 + H1 G2 H∗1 + σw2 Υ0 (IJ ⊗ IP ) = M0 F0 + Y,. (2.23). ˆ 0 , the least squares approxiwhere Y = σw2 [IJ IJ · · · IJ ]T ∈ RJP ×J . Thus from (2.18), F mation of F0 , can be written by ˆ 0 = (MT M0 )−1 MT (M0 F0 + Y) = F0 + (MT M0 )−1 MT Y = F0 + Z, F 0 0 0 0. . (2.24). Υ0 (Rx ¯). which is F0 plus a perturbation term due to noise. The perturbation term Z is the least squares solution of the equation M0 Z = Y. We note that if every column of Y is orthogonal ˆ 0 = F0 . But that is impossible since to every column of M0 , then Z = 0, which implies F the entries of M0 are positive and those of Y are nonnegative. Therefore, we seek to appropriately choose the precoding sequence p(n) such that every column of Y is as close to being orthogonal to that of M0 as possible. To this end, we first define qki and yi shown below as the columns of M0 and Y, respectively: q q · · · q q q · · · q · · · q q · · · q 0J 1J LJ M0 = 01 02 11 12 L1 L2 , M0 (:,1:J). M0 (:,J+1:2J). Y = σw2 [IJ IJ · · · IJ ]T = [y1 y2 · · · yJ ]. 13. (2.25). M0 (:,LJ+1:(L+1)J). (2.26).
(50) Then, due to the special structure of the block matrix M0 and Y, it is easy to check that qki is orthogonal to yj , i.e., qTki yj = 0 for j = i, e.g., qT01 y2 = [p(0)2 0 · · · 0 · · · p(P − 1)2 0 · · · 0][0 σw2 0 · · · 0 · · · 0 σw2 0 · · · 0]T = 0, . J entries. J entries. and each qTki yi assumes the same value, σw2. J entries. P −1 n=0. J entries. p(n)2 , for k = 0, 1, · · · , L, i = 1, 2, · · · , J,. e.g., qT01 y1. 2. 2. 0][σw2. σw2. = [p(0) 0 · · · 0 · · · p(P − 1) 0 · · · 0 ···0 ··· 0 · · · 0] = . J entries. J entries. J entries. J entries. T. σw2. P −1 . p(n)2 .. n=0. Thus we only need to consider the relation between columns of q01 and y1 (the case of k = 0 and i = 1). Define the correlation coefficient γ=. qT01 y1 . q01 2 y1 2. (2.27). Since γ is nonnegative and by Cauchy-Schwarz inequality, 0 ≤ γ ≤ 1. In order to make the perturbation term Z small, we choose q01 so that the correlation coefficient γ is as small as possible. Based on this point of view, we formulate the optimal selection problem as minimizing γ subject to P −1 1 |p(n)|2 = 1, P n=0. |p(n)|2 ≥ τ > 0,. ∀ 0 ≤ n ≤ P − 1.. (2.28). (2.29). Roughly, constraint (2.28) normalizes the power gain of the precoding sequence of each transmitter to 1; constraint (2.29) requires that at each instant, the power gain is no less than τ . Note that the problem of selecting the precoding sequence is identical to the SISO case considered in [16]. Thus the optimal precoding sequence p(n) is a two-level sequence with a single peak in one period [16]. More specifically, for each m, 0 ≤ m ≤ P − 1, ⎧ ⎨ P (1 − τ ) + τ , n = m (2.30) p(n) = ⎩ √τ , n = m, 0 ≤ n ≤ P − 1 is an optimal precoding sequence. Because the precoding sequence is periodic with period P , the single peak can be placed at any one of the P positions which yield the same γ = 14.
(51) √. 1 . P (1−τ )2 +τ (2−τ ). Note that γ decreases as τ decreases, which implies that the noise effect in. the estimation of covariance matrix Rx¯ is minimized and thus identification performance improves. However the peak location m does significantly affect the numerical condition of the linear equation (2.16). We discuss the selection of m next.. 2.3.2. On Selection of m. We now consider the selection of m. We know that different choices of m result in different matrix Mj and affect the numerical computation of Fj , j = 1, 2, · · · , L, in (2.18) and ˆ 0 in (2.24), since different MT Mj may have different condition number. If the condition F j number is large, then the matrix MTj Mj is ill-conditioned and the computations in (2.18) and (2.24) are sensitive to data error. Let µ = max κ(MTj Mj ), 0≤j≤L. (2.31). where κ(A) is the condition number of A. Our goal is to choose m so as to minimize the largest condition number of the corresponding matrices MTj Mj , j = 0, 1, · · · , L. Since the peak appears at one of the P possible positions in the periodic precoding sequence, there are P precoding sequences which may result in P different µ. The following result shows that some choices of m are to be avoided since they result in some Mj being rank deficient and thus µ = ∞. Proposition 2.2 : At least one Mj , 0 ≤ j ≤ L, is not full column rank if and only if P − L + 1 ≤ m ≤ P − 2. Proof : See Appendix A. Hence if we choose, either 0 ≤ m ≤ P − L or m = P − 1, then each Mj is full column rank and the channel is identifiable. The following result shows that we can classify the remaining choices into 2 groups that are relevant to the optimal choice of m. Proposition 2.3 : (a) Each of the (P − L) choices, m = 0, m = 1, · · · , m = P − L − 1, results in the same µ denoted by µ1 . (b) The two choices m = P − L and m = P − 1 result in the same µ denoted by µ2 . Also 15.
(52) µ2 ≥ µ1 . Proof : See Appendix A. From Proposition 2.3, we know if µ2 > µ1 , then we choose case (a); if µ2 = µ1 , we proceed to compare the second largest condition numbers of the set of matrices {MTj Mj }Lj=0 for these two cases and choose the case whose value is smaller. If they are again equal, the same procedure can be done by comparing the third largest condition numbers and so on. Moreover, for 0 ≤ m ≤ P − L − 1 (case (a)), since the condition numbers of MTj Mj are the same for each fixed j, j = 0, 1, · · · , L, (see Appendix A), we can use m = 0 to represent case (a). Similarly, m = P − 1 can be used to represent case (b). Hence the optimal selection of m reduces to one of two cases: m = 0 or m = P − 1. In other words, the optimal precoding sequence has a peak either at the beginning or at the end.. 2.4. Identification Algorithm. So far, we have proposed a method for blind identification of MIMO FIR channels using periodic precoding sequence. It is shown that, by properly choosing the precoding sequence, the MIMO FIR channels, with K transmitters and J receivers, can be identified up to a unitary matrix ambiguity. The proposed algorithm requires solving linear equations and computing the nonzero eigenvalues and eigenvectors of a Hermitian positive semidefinite matrix. Since the cyclostationarity is induced at the transmitter, the identifiability condition imposed on the channel is minimum: it only requires that channel impulse response matrix H is full column rank. The channel identifiability condition needs not be irreducible or column reduced. The channel can have more receivers or more transmitters. The performance of the algorithm depends on the precoding sequence which is optimally designed to reduce the effects of noise and numerical error in estimating the covariance matrix of the received data. We summarize the proposed method as the following algorithm. Algorithm : 1) Use the precoding sequence p(n) in (2.30) with optimal selection of m = 0 or m = P − 1 to form the matrix Mj in (2.17), j = 0, 1, · · · , L. ˆ x¯ = 2) Estimate the covariance matrix Rx¯ via the time average R 16. 1 S. S i=1. x ¯(i)¯ x(i)∗ , where.
(53) S is the number of data block (i.e., SP is the number of samples for each transmitter). 3) Compute Fj , formed by the channel product matrices, for j = 0, 1, · · · , L, using (2.18). 4) Form the matrix Q as in (2.19), and obtain the channel impulse response matrix (2.21) by computing the K largest eigenvalues and the associated eigenvectors of Q.. 2.5. Simulation Results. In this section, we use several examples to demonstrate the performance of the proposed method. The channel normalized root-mean-square error (NRMSE) is defined as I 1 1 (i) − H2 , H NRMSE = F HF I i=1. (2.32). (i) (1)T · · · H (i) = [H (i) (0)T H (i) (L)T ]T is the where · F denotes the Frobenius norm. H estimate of channel impulse response matrix H after removing the unitary matrix ambiguity by the least squares method [20, 21], and I = 500 is the number of Monte Carlo runs. The input source symbols are independent and identically distributed (i.i.d.) QPSK signals. The channel noise is temporally and spatially white Gaussian. The signal-to-noise ratio (SNR) at the output is defined as SNR =. P −1. 1 P. E[t(n)22 ] , E[w(n)22 ] n=0. where t(n) = [t1 (n) · · · tJ (n)]T. is the signal component of the received signal (see Figure 2.1). 1) Simulation 1 – optimal selection of precoding sequences In this simulation, we use the following model ⎤ ⎡ ⎤ ⎡ −1.45 + 0.21i −1.35 + 0.21i 1.34 − 0.55i 1.67 + 0.12i ⎦ z −1 ⎦+⎣ H(z) = ⎣ 0.62 − 0.31i −0.76 + 0.43i −0.69 + 0.25i −0.51 − 0.33i . ⎡ +⎣. H(0). −0.31 + 0.15i −0.41 − 0.16i. ⎤ ⎦ z −2. −0.29 + 0.21i −0.25 − 0.14i. . H(1). (2.33). H(2). to demonstrate the effect of different precoding sequences on the performance of the proposed method. In experiment 1, the first sequence is chosen as {0.767 1.07 1.07 1.07}, 17.
(54) which satisfies (2.28) and (2.29). The second and third sequences are chosen based on (2.30) for P = 4 and τ = 0.5878 with the two possible peak positions: m = 0 and m = 3. By computation, the corresponding µ for the three cases are 40.0, 4.66 and 22.1, respectively. Thus m = 0 is the optimal selection. Figure 2.2 shows that for SNR=10 dB, there are about 5∼7 dB and 5∼9 dB difference in NRMSE between the optimal one and two others. In experiment 2, we use the precoding sequences that satisfy (2.30) with m = 0, but with different τ to test the effect of τ on the identification performance. Figure 2.3 shows that for each sequence, when the number of samples (for each transmitter) is fixed at 1000, the NRMSE decreases as SNR increases and is roughly constant for SNR ≥ 20 dB. Figure 2.3 also shows that the identification performs better for smaller τ , which is consistent with the conclusion at the end of Section 2.3.1. 2) Simulation 2 – channel order overestimation In this simulation, we use the following channel model ⎤ ⎡ ⎤ ⎡ ⎤ ⎡ −0.4851 0.9387 0.7276 −0.1280 0.4851 0.3200 ⎦ z −1 + ⎣ ⎦ z −2 ⎦+⎣ H(z) = ⎣ 0.8823 0.8729 0.2941 −0.4364 −0.3676 0.2182 . H(0). H(1). H(2). (2.34) ˆ 0 ≤ (L ˆ − L) ≤ 6, we choose P = L ˆ + 2, SNR=10 given in [19]. For each upper bound L, dB, and 1000 samples (for each transmitter) for simulation. The precoding sequences are chosen as (2.30) with m = 0 and τ = 0.2, 0.4, 0.6, and 0.8. Figure 2.4 shows the NRMSE increases with increasing channel order overestimation. We see the proposed method is quite robust to channel order overestimation when τ is small. For example, with τ = 0.4, ˆ − L) increases from 0 to 3, the NRMSE increases from -25.5dB to -21dB, which when (L is still a low value. 3) Simulation 3 – a 3-input 2-output channel. 18.
(55) In this simulation, we use the 3-input 2-output model ⎡ ⎤ ⎤ ⎡ ⎤ ⎡ −0.44 0.35 0.14 0.13 0.01 0.08 1.6 0.88 0.66 ⎦ z −1 + ⎣ ⎦ z −2 ⎦+⎣ H(z) = ⎣ −0.14 0.37 0.23 0.26 0.02 0.16 0.8 0.44 0.33. H(0). H(1). H(2). (2.35) to illustrate the performance of the proposed method for channel with more transmitters than receivers. Note that H is full column rank, but the channel is not irreducible [21] because H(0) is not full rank, and it is not column reduced [21] either because H(2) is not full rank. In experiment 1, the precoding sequences (P = 4, τ = 0.5878) are given as in (2.30) with m = 0 and m = 3, respectively. Figure 2.5 shows that the NRMSE decreases as the number of data samples increases for SNR=10 dB. As expected, m = 0 case (the optimal selection) is better than m = 3 case. In experiment 2, we use the precoding sequences that satisfy (2.30) with m = 0, but with different τ to test the effect of τ on the identification performance. Figure 2.6 shows that for each sequence, when the number of samples (for each transmitter) is fixed at 1000, the NRMSE decreases as SNR increases and is roughly constant for SNR ≥ 25 dB. Figure 2.6 also shows the identification performs better for smaller τ . 4) Simulation 4 – channel equalization performance In this simulation, we use the channel model given in (2.34) to demonstrate the performance of the proposed method for channel equalization. We use the precoding sequences that satisfy (2.30) with m = 0, but with different τ to test the effect of τ on the equalization performance. For simplicity, we use the minimum mean square error (MMSE) equalizer. The equalizer is a 17-tap Wiener filter with 12-tap reconstruction delay whose jth output uˆj (k) is an estimate of uj (k) for j = 1, 2, · · · , K. Since the precoding scheme is applied at the transmitter, we need to multiply uˆj (k) by the corresponding p(k)−1 to obtain an estimate of sj (k) for j = 1, 2, · · · , K. The number of samples is 1200. We first identify the channel using the first 400 samples and then do equalization. Figure 2.7 shows that under low SNR, the proposed method performs better when τ is large; however, under high SNR, the proposed method performs better when τ is low. A 19.
(56) possible explanation is as follows. Channel estimates become more accurate as τ becomes smaller, but the gains p(k)−1 = √1 , τ. k = 1, 2, · · · , P −1 become larger and result in larger noise amplification at the receiver.. Both channel estimation error and channel noise contribute to the (maximum likelihood) detection performance, i.e., the symbol error rate. In the low SNR region, the detrimental effect of noise amplification outweighs the benefit of small estimation error; whereas in the high SNR region, accurate channel estimation weighs more than the noise amplification effect. Hence we choose a small τ when SNR is high and a large τ when SNR is low. 5) Simulation 5 – Comparisons with other methods In this simulation, we generate 100 2-input 4-output random channels with order L = 2; each element in the channel impulse response matrix is a complex circular Gaussian random variable with unit variance. We compare the proposed method with a generalized space time block codes (GSTBC)[23] based method. Both methods require periodic precoding sequences. For the proposed method, the precoding sequence is chosen as {1.500 0.767 0.767 0.767}; whereas the entries in the precoding sequence for the GSTBC method is chosen as random entries with modulus 1 for each random channel simulation [23]. The performance of the proposed method is also compared with a linear prediction (LP)[2, chap. 6] based method, and an outer product decomposition algorithm (OPDA)[20]. Both methods do not require a periodic precoder. MMSE equalizers are used for the proposed method, LP method, and OPDA method. For the GSTBC method, we use the customized equalizer proposed in [23]. Figure 2.8(a) shows that when the number of samples is 1200 (for each transmitter), the identification performance of the proposed method is better than those of the other three methods excepting the GSTBC method for SNR ≥ 13 dB. However, Figure 2.8(b) shows the equalization performance of the proposed method is only better than those of the LP and OPDA methods and worse than the GSTBC method. The inconsistency of the channel estimation and equalization performance of the proposed method and the GSTBC method for SNR ≤ 13 dB may be due to the different precoding sequences and equalizers used. Figure 2.9 shows that when the number of samples is 200 (for each transmitter), the identification and equalization performance of the proposed method is better than that of the GSTBC method for SNR ≤ 15 dB. Figure 2.9 shows that 20.
(57) when the number of samples is small, the proposed method has better performance than the GSTBC method under low SNR.. 2.6. Summary. In this chapter, we have proposed a blind identification method for general MIMO FIR channels using periodic precoding. We optimally design the precoding sequence against the effects of channel noise and numerical error, so as to increase the accuracy of channel estimation. The channel identifiability only requires that channel impulse response matrix H is full column rank, which is more relaxed than irreducible or column reduced. In addition, the channel can have more receivers or more transmitters. This algorithm can be used in the case of channel order overestimation. Simulation results are used to demonstrate the performance of the proposed method.. 21.
(58) −6 m=0 m=3 non−optimal p(n). −8. Channel NRMSE(dB). −10 −12 −14 −16 −18 −20 −22 −24 100. 200. 300. 400. 500 600 700 Number of Samples. 800. 900. 1000. Figure 2.2. Channel NRMSE versus number of samples. −10 τ=0.2 τ=0.4 τ=0.6 τ=0.8. channel NRMSE(dB). −15. −20. −25. −30. −35. 0. 5. 10. 15. 20 SNR(dB). 25. 30. 35. Figure 2.3. Channel NRMSE versus output SNR. 22. 40.
(59) −10 −12. channel NRMSE(dB). −14 −16 −18 −20 −22 −24 τ=0.2 τ=0.4 τ=0.6 τ=0.8. −26 −28 −30. 0. 1. 2 3 4 overestimated channel order. 5. 6. ˆ − L) Figure 2.4. Channel NRMSE versus (L. −12 m=0 m=3. −13. channel NRMSE(dB). −14 −15 −16 −17 −18 −19 −20 −21 −22 100. 200. 300. 400. 500 600 700 number of Samples. 800. 900. 1000. Figure 2.5. 3-input 2-output model: channel NRMSE versus number of samples. 23.
(60) −12 τ=0.2 τ=0.4 τ=0.6 τ=0.8. −14. channel NRMSE(dB). −16 −18 −20 −22 −24 −26 −28 −30. 0. 5. 10. 15. 20 SNR(dB). 25. 30. 35. 40. Figure 2.6. 3-input 2-output model: channel NRMSE versus output SNR. 0. 10. −1. 10. symbol error rate. −2. 10. −3. 10. −4. 10. τ=0.2 τ=0.4 τ=0.6 τ=0.8 perfect channel knowledge. −5. 10. −6. 10. 0. 5. 10. 15. 20 SNR(dB). 25. 30. 35. Figure 2.7. Symbol error rate versus output SNR. 24. 40.
(61) 0. 5. 10 proposed method GSTBC method OPDA method LP method. 0. proposed method GSTBC method OPDA method LP method. −1. 10. −2. −10. 10. symbol error rate. channel NRMSE(dB). −5. −15 −20. −3. 10. −4. 10. −25 −5. 10. −30 −35. −6. 0. 5. 10 SNR. 15. 10. 20. (a) Channel NRMSE versus output SNR. 0. 5. 10 SNR(dB). 15. 20. (b) Symbol error rate versus output SNR. Figure 2.8. Comparison of NRMSE and symbol error rate, number of input samples = 1200. 0. −2. 10. proposed method GSTBC method. proposed method GSTBC method. −4 −1. −6. 10. symbol error rate. channel NRMSE(dB). −8 −10 −12 −14. −2. 10. −3. 10. −16 −4. −18. 10. −20 −22. −5. 0. 2. 4. 6. 8. 10 SNR. 12. 14. 16. 18. 10. 20. (a) Channel NRMSE versus output SNR. 0. 2. 4. 6. 8. 10 SNR(dB). 12. 14. 16. 18. 20. (b) Symbol error rate versus output SNR. Figure 2.9. Comparison of NRMSE and symbol error rate, number of input samples = 200. 25.
(62) Chapter 3 Blind Channel Identification for MIMO Single Carrier Zero Padding Block Transmission Systems In this chapter, we propose a blind identification method based on periodic precoding for another transmission systems, single carrier zero padding (SC-ZP) block transmission systems. The method uses periodic precoding on the source signal before transmission. The estimation of the channel impulse response matrix consists of two steps: (1) obtain the channel product matrices by solving a lower-triangular linear system and (2) obtain the channel impulse response matrix by computing the positive eigenvalues and eigenvectors of a Hermitian matrix formed from the channel product matrices. The method can be used in the case of channel order overestimation and is applicable to MIMO channels with more transmitters or more receivers. A sufficient condition for identifiability is simply that the channel impulse response matrix is full column rank. The design of the precoding sequence which minimizes the noise effect in covariance matrix estimation is proposed and the effect of the optimal precoding sequence on channel equalization is discussed. Simulations are used to demonstrate the performance of the method.. 26.
(63) 3.1. System Model and Formulation p(n) ?s1 (n) v1 (n) -. S/P. .. p(n) . ?sK (n) vK (n) .. .. ¯s1 (i) === =⇒. 1:M. S/P. ¯ 1 (i) u u1 (n) ZP ====⇒ P/S N :1. .. .. .. . ¯sK (i) === =⇒. w1 (n) ?x1 (n) t1 (n)- -. ¯ K (i) u ZP ====⇒ P/S. 1:M. MIMO. .. .. . .. . w (n) .. J Channel ?xJ (n) uK (n) tJ (n) FIR. N :1. Figure 3.1. An MIMO SC-ZP block transmission baseband model with periodic precoding. Consider the K-input J-output discrete time SC-ZP block transmission baseband model shown in Figure 3.1. At the transmitter, the kth input signal vk (n) is first multiplied by a positive P -periodic sequence, p(n) ∈ R, to obtain sk (n) = p(n)vk (n), where p(n+P ) = p(n), ∀ n. Then sk (n) is passed through a serial-to-parallel block whose output is ¯ sk (i) = [sk (iM) sk (iM + 1) · · · sk (iM + M − 1)]T .. (3.1). Then ¯ sk (i) is passed through a zero padding prefilter F1 = [IM 0TP ×M ]T ∈ R(M +P )×M whose output is u ¯k (i) = F1¯ sk (i) = [ ¯ sk (i)T . 0 · · · 0 ]T = [uk (iN) · · · uk (iN + M − 1). . M entries P entries. M entries. 0 · · · 0 ]T ,. (3.2). P entries. where N = M + P . Finally, u ¯k (i) is converted to uk (n) via a parallel-to-serial block and transmitted through the MIMO FIR channel. At the receiver, the jth received signal is xj (n) = tj (n) + wj (n), where tj (n) is the signal component at the output and wj (n) is the channel noise seen at the jth receiver. If we define x(n) = [x1 (n) x2 (n) · · · xJ (n)]T ∈ CJ , then x(n) can be written as x(n) =. L . H(l)u(n − l) + w(n) = t(n) + w(n),. (3.3). l=0. where u(n) = [u1 (n) u2 (n) · · · uK (n)]T , w(n) = [w1 (n) w2 (n) · · · wJ (n)]T , t(n) = [t1 (n) t2 (n) · · · tJ (n)]T , and H(l) ∈ CJ×K is the channel coefficient matrix whose jkth 27.
(64) element hjk (l), l = 0, 1, · · · , Ljk , is the impulse response from the kth transmitter to the jth receiver, and L = maxj,k {Ljk } is the order of the MIMO channel. We assume that ¯(i) = [x(iN)T x(iN + 1)T · · · x(iN + N − H(L) = 0J×K . Group the sequence of x(n) as x ¯(i) ∈ CKN and w(i) ¯ ∈ CJN similarly as x ¯(i), we have 1)T ]T ∈ CJN , and define u ¯(i) + H1 u ¯(i − 1) + w(i), ¯ x ¯(i) = H0 u. (3.4). where H0 is a JN × KN block lower-triangular Toeplitz matrix with the first block column being [H(0)T H(1)T · · · H(L)T 0TJ×K · · · 0TJ×K ]T ∈ CJN ×K , and H1 is a JN × KN block upper-triangular Toeplitz matrix with the first block row being [0J×K · · · 0J×K H(L) H(L− 1) · · · H(1)] ∈ CJ×KN . We assume that the receivers are synchronized with the transmitters. In addition, the following assumptions are made throughout this chapter.. (B1) The source signal v(n) = [v1 (n) v2 (n) · · · vK (n)]T ∈ CK is a zero mean white sequence with E[v(m)v(n)∗ ] = δ(m − n)IK ∈ RK×K , where δ(·) is the Kronecker delta function. The noise is white zero mean with E[w(m)w(n)∗ ] = δ(m − n)σw2 IJ ∈ RJ×J . In addition, the source signal is uncorrelated with the noise w(n), i.e., E[v(m)w(n)∗ ] = 0K×J , ∀ m, n. ˆ of the channel order L is known, P = L ˆ + 1, and M > P is a (B2) An upper bound L multiple of P . (B3) The channel impulse response matrix H = [H(0)T H(1)T · · · H(L)T ]T is full column rank, i.e., rank(H) = K.. In the next section, we derive an algorithm for blind identification of the MIMO channel impulse response matrix H using second-order statistics of the received data.. 3.2. Blind Channel Identification. In this section, we derive the proposed method under assumptions (B1), (B2), and (B3). We discuss an optimal design of the precoding sequence, which takes into account 28.
(65) the noise effect in the estimation of covariance matrix of the received data, so as to increase the accuracy in the computation of the Hermitian matrix HH∗ and thus reduce the channel estimation error. With the proposed optimal precoding sequence, the computation of HH∗ becomes particularly simple. Taking eigen-decomposition of HH∗, we obtain the channel impulse response matrix H up to a unitary matrix ambiguity.. 3.2.1. The Identification Method. We first derive the proposed method for the case where the channel order L is known with P = L + 1, there are more receivers, i.e., J ≥ K, and the noise is absent. The cases of channel order overestimation and more transmitters than receivers (i.e., K > J) are given in Section 3.2.2 and 3.2.3, respectively. The effects of noise and optimal design of the precoding sequence are discussed in Section 3.2.4. From (3.4), we know that only the last L block columns of H1 are non-zero and zeros are padded in the last P = L + 1 block rows of u ¯(i − 1) and u ¯(i) (see (3.2)). Hence the ¯ (i − 1) equals the zero vector, which can be seen from (3.5) below. product H1 u ⎡ ⎢ ⎢ ⎢ ⎢ ⎢ ⎢ ⎢ ⎢ ⎢ ⎢ ⎢ ⎢ ⎢ ⎣. H1. . ⎤ ⎡. u ¯ (i−1). . 0 · · · 0 H(L) · · · H(1) u ¯((i − 1)N) ⎥⎢ .. .. ⎥ .. ⎢ . . 0 ··· 0 0 ⎥⎢ . ⎥ .. .. ⎥⎢ .. . 0 H(L) ⎥ ⎢ 0 ··· . . ⎥⎢ ⎥⎢ 0 ··· 0 ··· 0 0 ⎥⎢ u ¯ ((i − 1)N + M − 1) ⎥⎢ .. .. .. ⎥⎢ ⎢ 0 ··· 0 . . . ⎥⎢ 0 ⎥⎣ ⎦ ˙ 0 · · · 0 · · · 0 0 0˙˙ L blocks. 29. ⎤ ⎥ ⎥ ⎥ ⎥ ⎥ ⎥ ⎥=0 ⎥ ⎥ ⎥ ⎥ ⎥ ⎦. (3.5).
(66) Hence (3.4) can be expressed in a simple form (noiseless case): x ¯(i). ⎡. . x(iN) ⎢ .. ⎢ ⎢ . ⎢ ⎢ ⎢ x(iN + L) ⎢ .. ⎢ ⎢ . ⎢ ⎢ ⎢ x(iN + M − 1) ⎢ ⎢ .. ⎢ . ⎣ x(iN + N − 1). ⎤. ⎡. H0. ⎤ ⎡. . H(0) ⎥ ⎢ .. . . ⎥ ⎢ . ⎥ ⎢ . ⎥ ⎢ ⎥ ⎢ ⎥ ⎢ H(L) · · · H(0) ⎥ ⎢ ⎥ ⎢ . . .. . . . . . ⎥=⎢ ⎥ ⎢ ⎥ ⎢ ⎥ ⎢ H(L) · · · ⎥ ⎢ ⎥ ⎢ .. ⎥ ⎢ . ⎦ ⎣. u ¯ (i). . u(iN) ⎥⎢ .. ⎥⎢ ⎥⎢ . ⎥⎢ ⎥⎢ ⎥ ⎢ u(iN + L) ⎥⎢ .. ⎥⎢ ⎥⎢ . ⎥⎢ ⎥⎢ ⎥ ⎢ u(iN + M − 1) H(0) ⎥⎢ ⎥⎢ .. . . ⎥⎢ . 0 . ⎦⎣ ˙ H(L) · · · H(0) 0˙˙. ⎤ ⎥ ⎥ ⎥ ⎥ ⎥ ⎥ ⎥ ⎥ ⎥ = He¯s(i), ⎥ ⎥ ⎥ ⎥ ⎥ ⎥ ⎦ (3.6). where He is the sub-matrix formed from the first M block columns of H0 and ¯s(i) = ¯ (i). Because [u(iN)T u(iN + 1)T · · · u(iN + M − 1)T ]T is the first M block entries of u u(iN) = [u1 (iN) u2 (iN) · · · uK (iN)]T (see the line below (3.3)) and uk (iN) = sk (iM) for k = 1, 2, · · · , K (see (3.2)), u(iN) = [s1 (iM) s2 (iM) · · · sK (iM)]T s(iM). Similarly, u(iN + m) = s(iM + m) for m = 1, 2, · · · , M − 1. Hence ¯s(i) = [s(iM)T s(iM + 1)T · · · s(iM + M − 1)T ]T . ¯(i). Let xf (i) = [x(iN)T x(iN + 1)T · · · x(iN + L)T ]T be the first J(L + 1) rows of x Then xf (i) = Hf sf (i),. (3.7). where Hf ∈ CJ(L+1)×K(L+1) is the sub-matrix formed from the first (L + 1) block columns and block rows of He , and sf (i) = [s(iM)T s(iM + 1)T · · · s(iM + L)T ]T . Also we know for k = 1, 2, · · · , K, sk (iM) = p(iM)vk (iM) = p(0)vk (iM) from (3.1) and assumption (B2). Hence s(iM) = [p(0)v1 (iM) p(0)v2 (iM) · · · p(0)vK (iM)]T = p(0)v(iM), where v(iM) = [v1 (iM) v2 (iM) · · · vK (iM)]T . Similarly, s(iM + n) = p(n)v(iM + n) for n = 1, 2, · · · , L. Therefore (3.7) can be written as. 30.
(67) ⎡. xf (i). . x(iN) ⎢ ⎢ ⎢ x(iN + 1) ⎢ ⎢ .. ⎢ . ⎣ x(iN + L). ⎤. Hf. ⎡. . ⎤ ⎡. H(0) ⎥ ⎢ ⎥ ⎢ ⎥ ⎢ H(1) H(0) ⎥ = ⎢ ⎥ .. ⎢ .. .. . ⎥ ⎢ . . ⎦ ⎣ H(L) H(L − 1) · · · H(0) . p(0)H(0) ⎢ ⎢ ⎢ p(0)H(1) = ⎢ ⎢ .. ⎢ . ⎣ p(0)H(L). . ⎤. p(0)v(iM) ⎥⎢ ⎥⎢ ⎥ ⎢ p(1)v(iM + 1) ⎥⎢ ⎥⎢ .. ⎥⎢ . ⎦⎣ p(L)v(iM + L). Hp. ⎡. sf (i). ⎤ ⎡. ⎥ ⎥ ⎥ ⎥ ⎥ ⎥ ⎦ vf (i). . v(iM) ⎥⎢ ⎥⎢ ⎥ ⎢ v(iM + 1) p(1)H(0) ⎥⎢ ⎥⎢ .. .. .. . ⎥⎢ . . ⎦⎣ v(iM + L) p(1)H(L − 1) · · · p(L)H(0). ⎤ ⎥ ⎥ ⎥ ⎥. ⎥ ⎥ ⎦ (3.8). Define S ∈ RJ(L+1)×J(L+1) as the matrix whose first block sub-diagonal entries are all IJ , i.e.,. ⎡ ⎢ ⎢ ⎢ ⎢ ⎢ S=⎢ ⎢ ⎢ ⎢ ⎣. 0 IJ 0 .. . 0. ⎤. ···. 0. ··· .. . IJ .. . ···. 0 .. .. 0 0. 0. ···. 0. 0 .. . . . .. . . IJ. ⎥ ⎥ ⎥ ⎥ ⎥ ⎥ ∈ RJ(L+1)×J(L+1) . ⎥ ⎥ ⎥ ⎦. 0. Rewrite (3.8) as xf (i) = [p(0)H p(1)SH · · · p(L)SL H]vf (i) = Hp vf (i).. (3.9). Taking expectation of xf (i)xf (i)∗ , we get the covariance matrix Rf = E[xf (i)xf (i)∗ ] = Hp H∗p .. (3.10). From (3.9), since Hp = [p(0)H p(1)SH · · · p(L)SL H], (3.10) can be written as Rf = p(0)2 HH∗ + p(1)2 SHH∗ ST + · · · + p(L)2 SL HH∗ (ST )L =. L . p(k)2 Sk HH∗ (ST )k .. k=0. (3.11). From [38, p.414], we know that the general matrix equation pj=1 Aj XBj = C can be equiv . p T alently expressed as a matrix-vector equation form, j=1 Bj ⊗ Aj vec(X) = vec(C), 31.
(68) where vec(·) is the vec-function which stacks up columns of a matrix. Hence the matrix equation (3.11) can be written in the following vector form: L L p(k)2 Sk HH∗ (ST )k = p(k)2 Sk ⊗ Sk vec(HH∗ ) = G · vec(HH∗ ). vec(Rf ) = vec k=0. k=0. (3.12) Here G is a block Toeplitz lower-triangular matrix shown as follows: ⎡ p(0)2 IJF 0 ··· 0 ⎢ ⎢ L ⎢ p(1)2 S p(0)2 IJF ··· 0 2 k k ⎢ p(k) S ⊗ S = ⎢ G= .. .. .. .. . ⎢ . . . k=0 ⎣ L p(L − 1)2 S L−1 · · · p(0)2 IJF p(L)2 S. ⎤ ⎥ ⎥ ⎥ ⎥ ∈ RF 2 ×F 2 , (3.13) ⎥ ⎥ ⎦. ∈ RJF ×JF is a block diagonal matrix with S on the diagonal where F = J(L + 1) and S blocks. Since G is square, the solution to (3.12) is vec(HH∗ ) = G−1 vec(Rf ). (3.14). provided p(0) = 0. We use the solution obtained in (3.14) to form a Hermitian matrix Q = HH∗ . Since rank(H) = K by assumption (B3), rank(Q) = K. Since Q is Hermitian and positive semidefinite, Q has K positive eigenvalues, say, λ1 , · · · , λK . We can expand Q as. K Q= ( λj dj )( λj dj )∗ , j=1. where dj is a unit norm eigenvector of Q associated with λj > 0. We can thus choose the channel impulse response matrix to be = [ λ1 d1 λ2 d2 · · · λK dK ] ∈ CJ(L+1)×K . H We note that H can only be identified up to a unitary matrix ambiguity U ∈ CK×K , = HU, since H H ∗ = HH∗ = Q. The ambiguity matrix U is intrinsic to blind i.e., H identification of multiple input systems using only second-order statistics technique [20, 21]. The ambiguity can be resolved using a short pilot block sequence [27]. ¯ (i), to identify Remark: In this section, we only use xf (i), the first L + 1 block rows of x the channel product matrix Q, since the lower-triangular structure of the sparse matrix G makes it easy to compute vec(HH∗ ), which can be seen in Section 3.2.5. If we use more 32.
(69) ¯ (i) for computing a larger covariance matrix and identify Q, the than L + 1 block rows of x accuracy of estimation improves while the computations required roughly increases with ¯ (i). the square of number of rows of x. 3.2.2. Channel Order Overestimation. So far we have assumed that the channel order L is known. If only an upper bound ˆ ≥ L is available, then following the same process given in Section 3.2.1, we obtain L. Lˆ ˆ −1 T ∗ vec(H 0 · · 0 ]T ∈ CJ(L+1)×K . ov Hov ) = [ k=0 Sk ⊗ Sk ] vec(Rf ) where Hov = [H · ˆ L−L blocks. Then we can also obtain Q =. Hov H∗ov .. ˆ − L) block columns and Note that the last (L. block rows of Q are zero. Hence again, rank(Q) = K and Q has K positive eigenvalues. ˆ ˆ = [dT 0 · · · 0]T ∈ CJ(L+1) Each of the associated eigenvectors has the form d where. d ∈ CJ(L+1) . Thus, we can identify the channel impulse response matrix, up to a unitary matrix ambiguity, from the K eigenvectors associated with the K positive eigenvalues of Q. In the noise free case, we can, in theory, also determine the actual channel order.. 3.2.3. More Transmitters Than Receivers. In the above discussions, we assume that there are more receivers than transmitters, i.e., J ≥ K. If there are more transmitters, i.e., K > J, then either J(L + 1) ≥ K or K > J(L + 1). If J(L + 1) ≥ K, then H is a tall matrix and assumption (B3) is generically satisfied. Hence the proposed method still applies. If K > J(L + 1), then rank(H) < K and assumption (B3) does not hold. Hence the proposed method is applicable to the more transmitters case, provided the additional condition J(L + 1) ≥ K is satisfied. In addition, we note that in the proposed method, the channel condition is assumption (B3), i.e., the channel impulse response matrix H is full column rank. Hence the channel needs not be irreducible or column reduced.. 33.
(70) 3.2.4. Optimal Design of the Precoding Sequence. When the noise is present, the covariance matrix Rf contains the contribution of noise. Thus (3.10) becomes Rf = E[xf (i)xf (i)∗ ] = Hp H∗p + σw2 IF ,. (3.15). In this case, (3.12) becomes vec(Rf ) = G · vec(HH∗) + σw2 vec(IF ).. (3.16). From (3.14), the approximate solution of vec(HH∗ ) is ∗ ) = G−1 vec(Rf ). vec(HH. (3.17). It follows from (3.17) and (3.16) that ∗ ) = vec(HH∗) + σ 2 G−1 · vec(IF ) = vec(HH∗) + σ 2 z. vec(HH w w . (3.18). z. The vector z = [z1 z2 · · · zF 2 ]T in (3.18) is the solution of Gz = vec(IF ). Since the matrix G is completely determined by the precoding sequence p(n), we seek to choose p(n) so that z22 is minimized. To this end, we need to analyze the relations between z and p(n). By expanding the matrix equation Gz = vec(IF ), we find that ⎧ ⎪ ⎪ p(0)2 zi = 1 i = 1 + k(F + 1), k = 0, 1, · · · , J − 1 ⎪ ⎪ ⎪. 1 ⎪ ⎪ ⎪ p(n)2 zi+(1−n)J(F +1) = 1 i = 1 + k(F + 1), k = 0, 1, · · · , J − 1 ⎪ ⎨ n=0. 2 2 i = 1 + k(F + 1), k = 0, 1, · · · , J − 1 n=0 p(n) zi+(2−n)J(F +1) = 1 ⎪ ⎪ ⎪ .. .. .. ⎪ ⎪ . . . ⎪ ⎪ ⎪. ⎪ ⎩ L p(n)2 z i = 1 + k(F + 1), k = 0, 1, · · · , J − 1 i+(L−n)J(F +1) = 1 n=0. (3.19). and zj = 0 for all other indices j. We write (3.19) as the following matrix equation. ⎡ ⎤⎡ ⎤ ⎡ ⎤ 0 ··· 0 g m 1 ⎢ 0 ⎥⎢ 0 ⎥ ⎢ ⎥ ⎢ ⎥⎢ ⎥ ⎢ ⎥ ⎢ g1 g0 · · · 0 ⎥ ⎢ m1 ⎥ ⎢ 1 ⎥ ⎢ ⎥⎢ ⎥ ⎢ ⎥ (3.20) ⎢ .. .. ⎥ ⎢ .. ⎥ = ⎢ .. ⎥ .. .. . ⎢ . ⎥ ⎢ . . . ⎥ ⎢ . ⎥ ⎣ ⎦⎣ ⎦ ⎣ ⎦ gL gL−1 · · · g0 mL 1 . m. Gs. 34. y.
數據


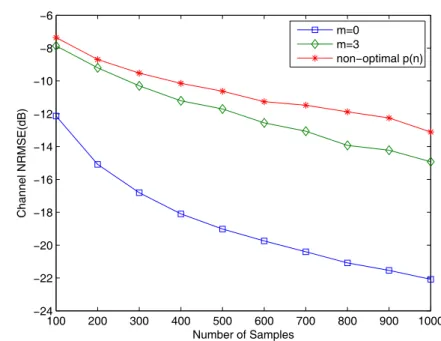
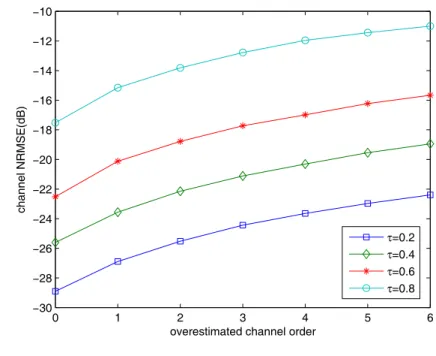
相關文件
In this paper, we propose a practical numerical method based on the LSM and the truncated SVD to reconstruct the support of the inhomogeneity in the acoustic equation with
In this paper, we have shown that how to construct complementarity functions for the circular cone complementarity problem, and have proposed four classes of merit func- tions for
For the proposed algorithm, we establish a global convergence estimate in terms of the objective value, and moreover present a dual application to the standard SCLP, which leads to
For the proposed algorithm, we establish its convergence properties, and also present a dual application to the SCLP, leading to an exponential multiplier method which is shown
In this work, for a locally optimal solution to the NLSDP (2), we prove that under Robinson’s constraint qualification, the nonsingularity of Clarke’s Jacobian of the FB system
This kind of algorithm has also been a powerful tool for solving many other optimization problems, including symmetric cone complementarity problems [15, 16, 20–22], symmetric
The evidence presented so far suggests that it is a mistake to believe that middle- aged workers are disadvantaged in the labor market: they have a lower than average unemployment
In this thesis, we have proposed a new and simple feedforward sampling time offset (STO) estimation scheme for an OFDM-based IEEE 802.11a WLAN that uses an interpolator to recover