漢語問句偵測之量化研究
全文
(2) 漢語問句偵測之量化研究 A Quantitative Study on Mandarin Question Detection 研 究 生: 葉秉哲. Student:. 指導教授: 袁賢銘 博士. Advisor: Dr. Shyan-Ming Yuan. Ping-Jer Yeh. 國 立 交 通 大 學 電 機 資 訊 學 院 資 訊 科 學 系 博 士 論 文. A Dissertation Submitted to Department of Computer and Information Science College of Electrical Engineering and Computer Science National Chiao Tung University in Partial Fulfillment of the Requirements for the Degree of Doctor of Philosophy in Computer and Information Science July 2004 Hsinchu, Taiwan, Republic of China. 中 華 民 國 九 十 三 年 七 月.
(3)
(4)
(5) 漢 語 問 句 偵 測 之 量 化 研 究 研究生:葉秉哲. 指導教授:袁賢銘 博士. 國立交通大學資訊科學研究所. 摘. 要. 「問句」是日常生活中最為人使用的語言行為之一,在電腦科學裡,舉凡人機對談、 機器間對談、標點處理等次領域中,也都扮演著重要角色。少了「問句偵測與處理」此 一環節,自然語言處理系統就不算完整。 由於語言本質的差異,再加上傳統上研究重心的不同,漢語的問句偵測要比英語更 加困難。有鑑於此,本篇論文鎖定在這個相形之下較為基礎的議題上,並採取量化研究 的角度。由於電子化語料資源的限制,本研究暫時只探討詞彙句法層次。 為了解決此一全新議題,本研究的策略是先追求召回率,再追求精確率。在召回率 方面,我們先以數種統計推論及樣式比對技術進行單變數分析,成功發掘出較傳統語言 學文獻所列更豐富、精確的詞彙特徵。接著我們以白箱式的雙變數分析排除部份誤判情 況,以提升精確率。最後我們以數種黑箱式的語言模型技術進行複變數分析,成功分辨 出更多情況。 在此研究中,我們達到不錯的召回率及精確率,並在漢語問句偵測議題上開拓一條 新的量化研究途徑。.
(6) A Quantitative Study on Mandarin Question Detection Student: Ping-Jer Yeh Advisor: Dr. Shyan-Ming Yuan Department of Computer and Information Science National Chiao Tung University. ABSTRACT Question is one of the most fundamental and frequent speech acts in everyday life. It also plays an important role in sub-areas of computer science such as human-computer and computer-computer communication, and punctuation processing. An NLP application is not complete without proper detection and processing of question. Detection of Mandarin question is more difficult than that of English due to the nature of the language itself and the research focus in the Mandarin linguistics and NLP field. It is therefore the focus of this research to undertake a quantitative study on the more fundamental problem of detecting Mandarin question. Due to limited electronic resource, the study is confined to lexico-syntactic level. To tackle this new topic, our strategy is first trying to maximize recall and then to increase precision. To achieve higher recall, we first undertake univariate analysis on the datasets with a variety of statistical inference and pattern matching techniques. At this stage we successfully discover more comprehensive and precise features at word level than what linguistic literature has mentioned before. Next, to increase precision, we undertake white-box bivariate analysis to filter out some false positives from the previous stage. Finally we undertake black-box multivariate analysis by using several language modeling techniques. In this way we successfully discriminate more cases. We achieve good recall and precision in the preliminary study, and pioneer the quantitative study of Mandarin question.. Keywords: Mandarin question detection, natural language processing (NLP), statistical inference, language models. iv.
(7) ACKNOWLEDGEMENTS. —kNx, ö.ø*Sz– ?êA¥¹H“d, íl|b>áíuBíNû`¤−²= ³]íAâÇp Tê, B.ª?œ}Êû˝Þ®Öjþt«Ô; ³]íXM, B.ø´”Öý−Êø ‘A^Bí˜,œœ7W; ]íNù, yuBÊ(O ˚;víøÑp˚ áá] wŸb >áDÆ`¤¸{YÃ`¤, *dl•zTÇá, øòƒÕ¨tí®_¼¨, Ô{ d!Z û˝¦² xX¿µÞ, ·#8BÝÖíuÇN`, éBœ}¿p¥2/ <‡æ, UHy¶ô 6b>áq¨tãº: >×’lC`¤, Õ¨tãº: 2E ’ô`¤ «×’ Ï]ı`¤ 2Û’ ÏÅP`¤ ‰½’˚d`¤, ì 52#8Bí£‡ ÊÚdj²í¬˙2, BbÕ>áÀ×’ "?ð`¤ ê=]í AÍxkT Ü {˙, Çó7B’mäíÇøm¢, úbç_*(WíÃãb°, 6éBú¥á ‚ÂÖ7ø}¦³ Ê>×xdF Charles Lee `¤í lxkçxeéxkç { Ð,, Bõƒ7* ˙äWBxkçäí\š yb>á>×xdF˜1Ô`¤, ê =]í Æ¶çÈx<çwøx<ç {˙, âdÞ, éB>§ƒDÜ Íi Í.°íˇ; Ê.°*íéÓ-, B|˘%íFäû˝)JMÚA$ ]íù£2 ¥, UBœ}ÊxdFÇ7sŸxe}&{˙, `çóÅ, y¿“Búóɇæí2&h õ AÖç7̤, †‚7›9 û˝Þ®2, Jý7õð#-b, öØ;d}uS m "Ø˙çÅ SÜ çÅ.víÉΣNû, ‹§Bí2¥ DD r: „æ õ Ø:† òäÇAín£}-, Dn.û wp? 1C ÐP-A„®2࣠Ѭˇ, éBÊõðí[ÅÅ íõ.£e-, >á}àÍ$õðvííA ÊBd¨tí¶i9,, ’ÍŸí’‘8üd n15üd Ïüüd, õð ín.ûç! òäÇç! ŠNÖç_A, ÖŸ#8›Œ, éÊÎ×9‰-é)õI ÈíB, )Jß‚¾¬, áá5b ÿ]®, Bf=íÖ¤ (J£ ýA, ••), 5²=dÐáȳTƒ: Êœ. v.
(8) ©wk‡5í¥ˆ, ,u?#BrÖø4,íÅ— . . . . . . áá5Lj7Bíe… wõ, ¥Æu@vuâBVzíÚ à¤ø>, AÞ?? öa1¬ zÑk¡í“J vm: B}Ô+—, ·Íuœ . . . . . . Ô, >á5¥<näJVÊ8>,XMB, ÊÞº,è¥B; éBç}lD§íöÞ, éB^êAÞíÿ¨ ¥³äNkÎè5, BàyÅíu~VÑ f=íf ‚f, ³5b, B.ª?³(è5RË•ƒÛÊ ô>5-uÑ.ê í, cJ¥¹üüíd, .#5b, J£ÊÙ,탂 ...... H“dêA, .ŠO¸©A°ší>¾: V³©F—ì ÒÍí, µAºÊ, ˚ Ê™™T |(, Z)Ú The Mummy(ÿfJ) ³Þ Imhotep íøÆO±«ÈdÑ!!: “Doctoral degree is only the beginning.”. vi.
(9) TABLE OF CONTENTS ABSTRACT . . . . . . . . . . . . . . . . . . . . . . . . . . . . . . . . . . iii ACKNOWLEDGEMENTS . . . . . . . . . . . . . . . . . . . . . . . . .. v. LIST OF TABLES. x. LIST OF FIGURES. . . . . . . . . . . . . . . . . . . . . . . . . . . . . . .. . . . . . . . . . . . . . . . . . . . . . . . . . . . . . xi. LIST OF SYMBOLS AND ABBREVIATIONS . . . . . . . . . . . .. 1. I. INTRODUCTION . . . . . . . . . . . . . . . . . . . . . . . . . . . .. 3. 1.1. Motivation . . . . . . . . . . . . . . . . . . . . . . . . . . . . . . .. 3. 1.2. The Question-Detection Problem . . . . . . . . . . . . . . . . . . .. 4. 1.2.1. Question: A Linguistic View . . . . . . . . . . . . . . . . .. 4. 1.2.2. Human-computer Communication . . . . . . . . . . . . . .. 5. 1.2.3. Computer-computer Communication . . . . . . . . . . . . .. 8. 1.2.4. Punctuation Processing . . . . . . . . . . . . . . . . . . . .. 8. 1.3. Challenge in Mandarin . . . . . . . . . . . . . . . . . . . . . . . .. 9. 1.4. The Scope of This Study . . . . . . . . . . . . . . . . . . . . . . . 12. 1.5. Organization of this Dissertation . . . . . . . . . . . . . . . . . . . 13. II. LINGUISTIC BACKGROUND . . . . . . . . . . . . . . . . . . . . 14 2.1. Question Marks in Chinese Writing System . . . . . . . . . . . . . 14. 2.2. Ways to Express Questions in Mandarin . . . . . . . . . . . . . . . 14. 2.3. Exceptions: Question Words and Referentiality . . . . . . . . . . . 15. 2.4. Exceptions: The Influence of Higher Verbs . . . . . . . . . . . . . 17. III THE BIG PICTURE: RULE SCHEME AND PROCESS . . . . 19 3.1. Overall Strategy . . . . . . . . . . . . . . . . . . . . . . . . . . . . 19. 3.2. Syntax and Semantics of Rules . . . . . . . . . . . . . . . . . . . . 21. 3.3. The Training Process . . . . . . . . . . . . . . . . . . . . . . . . . 22. IV DATASETS: CHOICES AND PREPROCESSING . . . . . . . . 24 4.1. The Corpus. . . . . . . . . . . . . . . . . . . . . . . . . . . . . . . 24. 4.2. The Treebank . . . . . . . . . . . . . . . . . . . . . . . . . . . . . 25. vii.
(10) V. 4.3. Machine-Readable Dictionaries . . . . . . . . . . . . . . . . . . . . 29. 4.4. Other Non-Electronic Resources . . . . . . . . . . . . . . . . . . . 31. UNIVARIATE ANALYSIS . . . . . . . . . . . . . . . . . . . . . . . 32 5.1. 5.2. 5.3. Finding Question-Related Words . . . . . . . . . . . . . . . . . . . 32 5.1.1. Procedure . . . . . . . . . . . . . . . . . . . . . . . . . . . 32. 5.1.2. Coverage Test in Terms of Recall . . . . . . . . . . . . . . . 37. 5.1.3. Coverage Test by Dictionaries . . . . . . . . . . . . . . . . 40. QRW Classification and Exploration . . . . . . . . . . . . . . . . . 42 5.2.1. Particles and Interjections . . . . . . . . . . . . . . . . . . 43. 5.2.2. Inconsistent Segmentation of A-not-A Questions . . . . . . 45. 5.2.3. A-not-A Questions and Simplified Forms . . . . . . . . . . 47. 5.2.4. WH Questions . . . . . . . . . . . . . . . . . . . . . . . . . 47. 5.2.5. Lexical Semantics of h´e . . . . . . . . . . . . . . . . . . . . 48. 5.2.6. Lexical Semantics of Honorifics . . . . . . . . . . . . . . . . 50. 5.2.7. Evaluative Adverbs and Rhetorical Questions . . . . . . . . 52. 5.2.8. Person . . . . . . . . . . . . . . . . . . . . . . . . . . . . . 54. 5.2.9. Roles . . . . . . . . . . . . . . . . . . . . . . . . . . . . . . 56. Putting Them Together . . . . . . . . . . . . . . . . . . . . . . . . 61. VI BIVARIATE AND MULTIVARIATE ANALYSIS . . . . . . . . 62 6.1. Bivariate Analysis by Exception Rules . . . . . . . . . . . . . . . . 62. 6.2. Multivariate Analysis by Language Models . . . . . . . . . . . . . 63 6.2.1. Particles and Interjections . . . . . . . . . . . . . . . . . . 67. 6.2.2. A-not-A Questions and Simplified Forms . . . . . . . . . . 68. 6.2.3. WH Questions . . . . . . . . . . . . . . . . . . . . . . . . . 70. 6.2.4. Evaluative Adverbs and Rhetorical Questions . . . . . . . . 70. VII CONCLUDING REMARKS . . . . . . . . . . . . . . . . . . . . . . 73 7.1. Discussion . . . . . . . . . . . . . . . . . . . . . . . . . . . . . . . 73 7.1.1. False Negatives . . . . . . . . . . . . . . . . . . . . . . . . 73. 7.1.2. False Positives . . . . . . . . . . . . . . . . . . . . . . . . . 73. 7.1.3. Clause or Sentence Boundary . . . . . . . . . . . . . . . . . 74. viii.
(11) 7.2. Summary . . . . . . . . . . . . . . . . . . . . . . . . . . . . . . . . 74. APPENDIX A. — LIST OF QUESTION-RELATED WORDS . . 76. REFERENCES . . . . . . . . . . . . . . . . . . . . . . . . . . . . . . . . . 85. ix.
(12) LIST OF TABLES Table 1. A gentle overview of English question sentence patterns . . . . . 10. Table 2. Register distribution of question clauses in the Sinica corpus 3.0 . 25. Table 3. 2 × 2 contingency table for finding question-related words (QRWs) 34. Table 4. Top 40 question-related words (QRWs) found by statistical inference procedures . . . . . . . . . . . . . . . . . . . . . . . . . . . . 37. Table 5. Using the words extracted from the ABC Chinese-English Dictionary to validate QRWs . . . . . . . . . . . . . . . . . . . . . . 41. Table 6. Sentence-final particles and interjections co-occurring with questions in the Sinica corpus . . . . . . . . . . . . . . . . . . . . . . 44. Table 7. Honorifics found to be relevant to questions as a result of mining MOE’s Mandarin Dictionary Revised . . . . . . . . . . . . . . . . 52. Table 8. Keywords for rhetorical questions as a result of mining MOE’s Mandarin Dictionary Revised . . . . . . . . . . . . . . . . . . . . 55. Table 9. Top 10 question-related words in terms of recalls . . . . . . . . . 55. Table 10. Relation between person and degree of relevance to questions in the Sinica corpus . . . . . . . . . . . . . . . . . . . . . . . . . . . 56. Table 11. Distribution of 2nd person pronouns and their respective semantic roles in the Sinica treebank . . . . . . . . . . . . . . . . . . . . . 58. Table 12. Different configurations used in our language modeling experiments 67. Table 13. List of top 300 question-related words (QRWs) . . . . . . . . . . 77. x.
(13) LIST OF FIGURES Figure 1. The big picture of overall training and detection structure . . . . 20. Figure 2. Grammar of question-detection rules at univariate and bivariate level . . . . . . . . . . . . . . . . . . . . . . . . . . . . . . . . . . 21. Figure 3. Overall training process of question detection at univariate and bivariate stages . . . . . . . . . . . . . . . . . . . . . . . . . . . . 22. Figure 4. Algorithm for finding the punctuation of a tree from the Sinica treebank by tracing its origin back to the Sinica corpus . . . . . . 28. Figure 5. Algorithm for finding question-related words . . . . . . . . . . . . 34. Figure 6. Comparison between LLR and Pearson’s χ2 tests on QRWs . . . 36. Figure 7. Algorithm for calculating cumulative recall and precision of QRWs 38. Figure 8. Cumulative recall and precision of question-related words . . . . . 38. Figure 9. QRW ranking in terms of LLR vs. in terms of frequency . . . . . 39. Figure 10 Minitab χ2 output for comparing the 5 roles of 2nd person pronoun “5” for Table 11 . . . . . . . . . . . . . . . . . . . . . . . . 59 Figure 11 Minitab ANOVA output for comparing the 5 roles of all 2nd person pronouns for Table 11 . . . . . . . . . . . . . . . . . . . . . . 60 Figure 12 Boxplot of Q/(Q+¬Q) ratio for different roles played by 4 second person pronouns . . . . . . . . . . . . . . . . . . . . . . . . . . . 61 Figure 13 The results applying rules of compound relatives and higher verbs 63 Figure 14 Prepare training and test sets by simple random sampling . . . . 64 Figure 15 Using language models to discriminate questions . . . . . . . . . 66 Figure 16 The result of using language models to discriminate the case of sentence-final particles . . . . . . . . . . . . . . . . . . . . . . . . 68 Figure 17 The result of using language models to discriminate the case of A-not-A questions . . . . . . . . . . . . . . . . . . . . . . . . . . 69 Figure 18 The result of using language models to discriminate the case of WH questions . . . . . . . . . . . . . . . . . . . . . . . . . . . . . 71 Figure 19 The result of using language models to discriminate the case of evaluative adverbs and rhetorical questions . . . . . . . . . . . . . 72. xi.
(14) LIST OF SYMBOLS AND ABBREVIATIONS Symbols. A, B, C, . . .. ordered arrays or associative arrays. A[i]. element of ordered array indexed by scalar number i. A[w]. element of associative array indexed (mapped) by textual word w. a, b, c, . . .. scalar numbers. F. ANOVA F statistic. Fa,b. F statistic with df. a in the denominator and b in the numerator. f, g, h. functions. P. probability function. p. probability value. r, s, t, . . .. character strings. S, W, . . .. unordered sets (i.e., bags) of possibly duplicated elements. |S|. scalar cardinality of S. w. word. z. alphabet or symbol. 1.
(15) Abbreviations. ANOVA. analysis of variance. BOW. Bilingual Ontological Wordnet project. CKIP. Chinese Knowledge Information Processing Group. df. or d.f.. degree of freedom. LLR. log-likelihood ratio. LM. language model. MOE. Ministry of Education, Taiwan. MRD. machine-readable dictionary. NLP. natural language processing. POS. part of speech. QRW. question-related word (invented by the author). regex. regular expression. SRS. simple random sample/sampling. 2.
(16) CHAPTER I. INTRODUCTION This thesis presents a new topic in the field of natural language processing (NLP): Mandarin question detection. Since no such prior research exists to our knowledge, one may ask two “why” questions: 1. Why is it important in general? 2. Why is it special for the Mandarin Chinese language, in particular? To address these questions, this chapter first presents the importance of this topic in a broader linguistic and computer science context, not limited to the Mandarin Chinese language. Afterwards we narrow our discussion down to the sole Mandarin field to see why it is still challenging. Finally we outline the overall research plans and results.. 1.1. Motivation. The whole research originates from a very simple question. In a classical NLP textbook Speech and Language Processing [28, p. 194], there is a paragraph saying that There are tasks such as grammar-checking, spelling error detection, or author-identification, for which the location of the punctuation is important . . . In NLP applications, question-marks are an important cue that someone has asked a question. Punctuation is a useful cue for part-of-speech tagging. However, it treats the punctuation as a given cue. One may then ask: What if the cue is absent at all? Perhaps the tasks mentioned above will be confronted with problems. 3.
(17) In what cases can punctuation be absent? To name but a few. In newsgroup writing, punctuation is often misused or missing, especially among the groups crowded with young people. In speech-to-text software, punctuation is usually absent from the generated text. Among all kinds of punctuation, question marks attract the author’s attention. Therefore, the goal of this study can be paraphrased as a question-detection problem.. 1.2. The Question-Detection Problem. The question-detection problem is, in short, to enable computers to detect the question parts, if any, within a stream of text or utterance. Its importance is twofold: linguistic and computer science perspectives. This section will first discuss the issue from the linguistic point of view, and then, from the computer science point of view, enumerate applications that can benefit from the study of question-detection problem: . Human-computer communication.. . Computer-computer communication.. . Punctuation processing.. 1.2.1. Question: A Linguistic View. From the linguistic science perspective, the study of speech acts has been a hot topic in discourse analysis, and “question” is one of the major illocutionary acts occurred in everyday life. The deeper our understanding of the nature of a variety of question expressions in particular, the better we may form a computational linguistics model for speech acts in general, which in turn improves application of linguistics. What do we mean by the term question, anyway? In Glossary of Linguistic Terms [35], question has two senses:. 4.
(18) 1. An illocutionary act that has a directive illocutionary point of attempting to get the addressee to supply information. 2. A sentence type that has a form (labeled interrogative) typically used to express an illocutionary act. It may be actually so used (as a direct illocution), or used rhetorically. Obviously they reflect two main competitive schools of thought in linguistics: the first addresses the functional facet, while the second addresses the formal facet. From the functional perspective, the following two cases are both questions in spite of totally different surface forms: (1). a. Tell me your age. b. How old are you? As for the formal perspective, there are roughly three types of questions: in-. terrogative, dubitative, and rhetorical questions. For example, (2). a. What is this?. interrogative. b. Can such a diligent student fail the school entrance exams? dubitative c. Don’t you understand me? 1.2.2. rhetorical. Human-computer Communication. As for human-computer communication, a non-toy human-computer dialogue or question answering system needs to distinguish between background information and foreground queries in order to behave more like humans. In such systems, therefore, earlier stages should include at least the question detection module; subsequent processing is fragile without considering it. Now let’s examine the two applications in detail. Question answering (QA) is a fast-growing sub-task of text retrieval. Given a query, it tries to pinpoint the specific answers (noun phrases, sentences, or short passages) rather than just give a pile of relevant documents for you to browse. The QA track of Text REtrieval Conference (TREC) is one of the most famous 5.
(19) example. Since the first QA track initiated in 1999 (TREC-8), the has been a lot of progress in this field (see [50, 51, 52, 53, 54]). Participants in this track are required to give an exact answer in response to a factoid question, a list of exact answers to a list question, and a short passage to a definition question. Look at the following excerpts from TREC QA tracks: (3). a. What is the longest river in the United States?. factoid. b. Name the highest mountain.. factoid. c. What are 5 books written by Mary Higgens Clark?. list. d. List the names of chewing gums.. list. e. Name 22 cities that have a subway system.. list. f. Who is Colin Powell?. definition. g. What are polymers?. definition. As reported, most QA systems first classify an incoming question into various types of query focus (e.g., quantity, name, time, and place) as suggested by its question word (e.g., what and who) or imperative verb (e.g., list and name); the expected answer types can also be predicted accordingly. Next, some systems attempt a full understanding of the text and then use logic proofs or so to verify candidate answers (e.g., [44]); still others just attempt a shallow, data-driven pattern matching against candidate answers (e.g., [33, 48]). There is at least one limitation of these QA systems, however. They assume that a QA system receives and recognizes only canonical query forms beginning with a question word or imperative verb. But in reality, not all questions fall into this category. Take the following real-world query for example.1 Imagine that you are asking a QA system for troubleshooting: (4). I have installed and configured Wine, but Wine cannot find MS Windows on my drive. Where did I go wrong?. 1. This paragraph is excerpted from The Wine FAQ. URL: http://www.winehq.com/site/ docs/wine-faq/index.. 6.
(20) It is hard to imagine that you are allowed to tell the program only the latter half “Where did I go wrong?” without the former “I have . . . on my drive.” Even if the unrealistic assumption was made, no program is smart enough to be able to answer the sole question “Where did I go wrong?”— the query focus is correctly identified as “where” but it is of little use here without preceding sentences. What is worse, the query focus “where” may mislead the program to an irrelevant direction of physical places! As a result, if the QA program fails to distinguish between foreground query and surrounding context, how can it work out a search plan to answer your “where” question?2 Things become even more complicated in dialogue system, in which conversation continues rather than just happens in one round, turn-taking is frequent, and a mixture of various speech acts such as illocutionary and perlocutionary may also be used freely [14]. Since natural conversation switches between both foreground and background expression frequently, it is unrealistic to assume naively that the dialogue system recognizes and accepts only query forms. Take the following excerpt from the novel Harry Potter and the Sorcerer’s Stone for example. One day Harry Potter said to Hagrid: (5). Everyone thinks I’m special, . . . but I don’t know anything about magic at all. How can they expect great things? I’m famous and I can’t even remember what I’m famous for. . . .. Assume for now that Hagrid is a computer. If Hagrid fails to distinguish between the two, it can never understand what Harry means by “great things” and then work out a search plan accordingly to try to comfort Harry by saying “Don’ you worry, Harry. You’ll learn fast enough.” 2. One may think that the QA system has a chance to function well if we force users to rephrase their query as “Where did I go wrong when I’ve installed and configured the Wine but it cannot find MS Windows on my drive?”. It may work, but is neither practical nor user-friendly.. 7.
(21) 1.2.3. Computer-computer Communication. As for computer-computer communication, intelligent agents or software robots may need to travel around the Internet and along the way gather information on behalf of their users. Since XML and semantic webs are still young and there is no universally accepted semantic markup language for unrestricted domains, unstructured documents still dominate the Web. Therefore, a better understanding of speech acts in general and questions in particular may help software analyze unstructured documents and transform them into structured ones. Furthermore, in multi-agent systems agent communication languages are based mostly on speech act theory (e.g., KQML defines a set of performatives for agents to communicate with [2, 29]) and temporal or first-order predicate logic (e.g., KIF [24]). Many information systems for intra- or inter-business process have also been modeled from the language/action perspective (LAP; see [56] for an overview of LAP and [16, 32] for typical applications). The study of question in natural language settings may help to enhance the expressiveness of communication facilities, finer-grained mental states, and belief-desire model of these systems. 1.2.4. Punctuation Processing. As for punctuation processing, any NLP system is not complete without punctuation processing, but punctuation has been neglected in the NLP field. For example, speech-to-text recognition software maps acoustic signals to text, but it seldom places appropriate punctuation marks in the output text. Word processors have built-in or plug-in spelling and grammar checkers, but they seldom try to check punctuation. Some literature did recognize the importance of punctuation more or less, as we have seen in Section 1.1. However, it treats the punctuation as a given cue, and does not discuss what if the cue is absent at all. The reason why punctuation has been neglected is that, it is such a complex. 8.
(22) coding device that challenges computers. It is, as defined in The American Heritage Dictionary [47], “the use of standard marks and signs in writing and printing to separate words into sentences, clauses, and phrases in order to clarify meaning.” Therefore, to assign punctuation correctly involves not only syntactic but also semantic and pragmatic levels of processing. Take the following English sentences for example, (6). a. Is this yours? b. What is it? c. I beg your pardon? d. This is yours? I don’t think so.. To punctuate them correctly with question marks, one has to judge whether they are questions. Sentence (6a) is obviously a question because of its verb BE-initial syntactic pattern; the same for Sentence (6b) because of its WH word-initial followed by a verb BE syntactic pattern. Sentence (6c), which begins without a verb BE, an auxiliary verb, or a WH word, is regarded as a question only if the lexical meaning of the word “pardon” is taken into account. Furthermore, Sentence (6d) is regarded as a question only if the pragmatic context is taken into account. Therefore, to be perfect, it is very complicated in general.. 1.3. Challenge in Mandarin. It is even more challenging for the Mandarin Chinese language because there is no syntactically decisive and reliable marker and word order in Mandarin question sentences [8], let along decisive and reliable semantic and pragmatic clues. Therefore, mainstream approaches to detecting question sentences developed on the basis of English (and possibly Indo-European languages as well) are not readily applicable here. Now consider the English language at the syntactic level only. Questions in English have well-understood and consistent patterns, which can be easily found in books or articles on English grammar, e.g., [58]. Patterns in Table 1 are easily 9.
(23) Table 1: A gentle overview of English question patterns, summarized from [58]. For brevity, “AUX” means auxiliary, “SUB” means subject, and “WH” means wh words such as who, why, how, and what. Note that some oral or idiomatic expressions such as “so what?” and “say what?” are not included here Question Type Pattern Example Inverted sentence yes-no question. AUX-initial + SUB. non-subject-extracted wh-moved . . .. WH + AUX + SUB. Will John buy a backpack?. NP complement. What was Beth asked by Diaia?. object of P. Which girl did Beth talk to?. PP. To which girl did Beth talk?. S complement. What does Clove want?. ADJ complement. How did he feel?. do-support. DO-initial + SUB. Did John buy a backpack?. subject wh-question. declarative order. Who wrote the paper?. non-subject-extracted wh-moved question. see above. Extraction. detected by computers; full-fledged parsers are even unnecessary for this sole task. Take a commonly-available full-fledged link grammar parser from Carnegie Mellon University for example.3 When a sentence is recognized as question, the leftmost link will be labeled with a Wq, Ws, Wj, or Q link type. Therefore, question detection in English is easy. Things are not quite the same in Mandarin, though. What do we mean in the beginning of this section by the statement that there is no syntactically decisive and reliable marker and word order in Mandarin question sentences? As for the word order, take sentence (7) for example, (7). a. ¥. u. This is. Bó. ?. what. 3. Software, documentation, and related information of the link grammar parser can be accessed at http://www.link.cs.cmu.edu/link/. You can also experiment with the parser on-line.. 10.
(24) What is this? b. Bó. n. v`. y. ?. What time. cÞ. ?. can again meet. What time can me meet again? c. 5. Ê. Bó. Ï. ‰a. ?. You be going eat what thing What are you eating? The word order of “Bó” (sh´enme; what) appears freely in sentences (7), unlike English. Things become even more complicated that “Bó” alone is not a reliable and decisive marker for question. For example, (8). a. 5. Bó. ‰a. · ;. Ï. . You what thing all want eat You want to eat everything. b. B I. V. . õ. come buy CL. Bó. ß . what. Let me buy something. To our knowledge, no prior research in Mandarin has focused on exactly the same problem. From the linguistic perspective, traditionally Mandarin linguists discuss question sentences mostly at syntactic level and identify general typology of question expressions (see Section 2.2 for details). Recently researchers have tried to model the Mandarin questions using symbolic approaches such as propositional logic and lambda calculus (see [42] for a brief review). The big picture is very likely to be correct. Not in a corpus-oriented approach, however, they fail to identify more comprehensive and precise features, and lack stronger quantitative evidence. On the other hand, researchers from NLP and text retrieval fields also have tried to model the Mandarin question-answering problem as semantic frames and ontology [43]. But they all base on an ideal assumption that users issue no sentence other than well-formed questions. Mixed-type cases such as Sentence (4) are 11.
(25) beyond their scope of discussion. It is therefore the focus of this research to undertake a quantitative study on the more fundamental problem of detecting Mandarin question.. 1.4. The Scope of This Study. The goal of this study is to enable computers to detect the question parts, if any, within a stream of Mandarin text or utterance. Now we would like to define the scope of this study clearly. Textual, not prosodic. A declarative sentence may be used to express a question by rising intonation. This study considers only textual rather than prosodic issues. Lexico-syntactic, not semantic and pragmatic. The author takes the formal position instead of functional as mentioned in Section 1.2.1 for several reasons. Quantitative studies at semantic and pragmatic levels require many machine-readable resources. Since there is no adequate Mandarin corpus with functional annotation, a quantitavie study in this direction is difficult. As for the formal perspective, quality corpora have punctuation attatched to all sentences types. Among them, interrogative, dubitative, and rhetorical questions are labeled with question marks. Such corpora are readily applicable for this quantitative study. Therefore, at this stage only lexico-syntactic issues are considered in order to narrow down the scope of discussion. Written, not spoken. Spoken utterrance has unique characteristics not equally prominent in written text. To name but a few: conversational filters, ellipsis, and interrupts. They all require additional treatement among utterrance. The datasets in use for this study include some transcribed spoken utterrance, but the research focus is still on written text. That said, the author thinks that parts of the overall methodology remains roughly the same even for spoken utterrance. Detection, not generation. This study aims to detect Mandarin questions in contemporary use, not to detect superficial cases, nor to generate grammatical. 12.
(26) utterrance. Therefore, construction of a well-formed descriptive grammar is out of the scope of this study. That said, some research results here may provide a basis for a more thorough grammar for Mandarin questions.. 1.5. Organization of this Dissertation. The goal of this study is to detect Mandarin question sentences. To put it more concretely, our task, in respect of training and validation, is to label un-punctuated input text with appropriate question marks. This dissertation is organized as follows. Chapter 2 reviews linguistic literature on Mandarin questions. Chapter 3 outlines our overall strategy, rule scheme, and training procedures. Chapter 4 discusses the datasets used in this study, why, and what kinds of pre-processing should be done on them. Chapter 5 describes our feature-selection stage at univariate level and findings, and in the meantime re-examines literature from a different angle: statistical point of view. Chapter 6 describes bivariate and multivariate stages. Chapter 7 discusses our findings and concludes our main contributions.. 13.
(27) CHAPTER II. LINGUISTIC BACKGROUND 2.1. Question Marks in Chinese Writing System. Modern Mandarin punctuation system, inspired by the western culture, was stabilized and formalized in the 20th century [57]. Since then, prescriptive guidelines have been announced by authorities in major Mandarin-speaking regions, including Taiwan and mainland China [39, 45]. In general, question marks are used at the end of three kinds of question sentences: interrogative, dubitative, and rhetorical questions. For example,1 a. ¥uBó?. (9). interrogative. What is this? b. µóàŠíçÞ, }5.,ç?. dubitative. Can such a diligent student fail the school entrance exams? c. Ø−5´.7jB?. rhetorical. Don’t you understand me? However, these vague statements touch only superficial mood issues. For rigorous research, we need more information on their linguistic structures.. 2.2. Ways to Express Questions in Mandarin. There are, in general, two ways to express questions in Mandarin: prosodic and grammatical devices. It is not necessary for the scope and purpose of this paper to enter into a detailed discussion of the former issue. Therefore we only summarize 1. When Chinese people write or publish text, they do not separate characters with spaces, i.e., words are written down consecutively without delimiters as shown in sentences (9). But in the linguistics literature, Chinese words are usually delimited by spaces for the sake of the research community’s culture.. 14.
(28) intonation patterns from relevant literature. In an interrogative sentence, the focal words are usually stressed and the whole sentence usually ends with a rising intonation. In a dubitative sentence, the focal words are often lengthened, possibly with a high pitch. In a rhetorical question, it is usually spoken with a sustained or falling intonation. Interested readers may consult more literature on this topic, such as (Zhang [62]; Fan [19]). As for grammatical devices, various classification schemes have been proposed in literature. Some are compiled for educational purpose (Zhang [62]; Liu et al. [34]; Chu [11]), and others are for linguistic research purpose (Li and Thompson [30]; Lyu [37]; Fan [19]; Zhang [61]; Chu and Chi [12]). Some are classified mainly on the basis of morpho-syntactic forms (Li and Thompson [30]; Fan [19]; Chu [11]), some semantic types (Lyu [37]; Liu et al. [34]; Zhang [61]; Chu and Chi [12]), and others pragmatic functions (Zhang [62]).2 While the big picture is now widely accepted, there is still considerable disagreement about details.. 2.3. Exceptions: Question Words and Referentiality. Since no syntactically reliable marker exists in Mandarin question sentences, as mentioned in Section 1.3, exceptions are inevitable. In most of the exceptional cases, the WH words are used as indefinitives or compound relatives (Chu [11]; Chu and Chi [12]). There are roughly 5 cases as pointed out by (Chu [11]; Chu and Chi [12]). The case for indefinitives, as shown in Sentence (10), can be (and possibly can only be) identified from the context since there seems no obvious syntactic pattern. On the other hand, the case for compound relatives can be identified from syntactic patterns, as shown in Sentences (11)–(12). (10). a. B. b. _. A. 6. 2. The literature review is not meant to be definite. Some of them use hybrid criteria for classifying question sentences since there is no strict dividing line between morpho-syntactic, semantic, and pragmatic issues. Most of them also discuss more than one level of linguistic issues. Here we only point out the most prominent point of view.. 15.
(29) Wˇo. y`ao. jˇı-ge. r´en. b¯ angm´ ang. I. need how many people help. I need some people to help me. V. . Wˇo. l´ai. mˇai diˇ an. I. come buy CL. b. B. õ. Bó. ß. sh´enme ba what. Let me buy something.. (11). a. Bó. 9. Sh´enme sh`ı What. b. d?. y` ao. zu` o. things need do. What things do we need to do? Bó. b. ³ M´eiyˇou. 9. sh´enme sh`ı. Not exist what. b. d. y` ao. zu` o. things need do. Nothing needs to be done. c. Bó. 9. Sh´enme sh`ı What. ·. b. d. d¯ ou. y` ao. zu` o. things all. need do. Everything needs to be done. d. Bó. 9. Sh´enme sh`ı What. 6. b. d. yˇe. y` ao. zu` o. things also/even need do. Everything needs to be done.. (12). a. Õ Sh´ei. l. ƒ?. xi¯an. d`ao. Who first. arrive. Who arrived first? 16.
(30) b. Õ Sh´ei. l. ƒ,. Õ. l. d. xi¯an. d`ao. sh´ei. xi¯ an. zu` o. arrive. who. first. do. Who first. Let those who arrive first do it first. c. Õ Sh´ei. l. ƒ,. ÿ. (Õ). l. d. xi¯an. d`ao. j`ıu. (sh´ei). xi¯ an. zu` o. arrive. then. who. first. do. Who first. Let those who arrive first do it first.. 2.4. Exceptions: The Influence of Higher Verbs. In his articles [7, 8] Cheng investigated an interesting issue: higher verbs in a complex sentence may influence the decision whether the proceeding question form is interrogative or not. For example, (13). u. Bó. ‰a. Zh`e. sh`ı. sh´enme. d¯ ongx¯ı. This. is. what. thing. a. ¥. ?. What is this? V. |Œ. ¥. u. Bó. ‰a. Wˇo. l´ai. di`aoch´ a. zh`e. sh`ı. sh´enme. d¯ ongx¯ı. I. come. investigate. this. is. what. thing. b. B. . Let me investigate what it is. The verb “|Œ” (di`aoch´a ; investigate) in sentence (13b) will turn the question form in sentence (13a) into a non-question. He concluded in [7] that inquisitive and cognitive verbs will turn a embedded question form into non-interrogative because the focus is shifted from the question form to the higher verbs; while other types of verbs may or may not have the same effect. However, in his subsequent article [8] cognitive verbs were classified into the “may or may not” case without further explanation.. 17.
(31) There are still open issues regarding the influence of higher verbs. To name but a few: Is the classification scheme of verb types exhaustive, complete, and accurate? How to explain the exceptions to these higher-verb rules? Is there another theory to explain the phenomena better? We will re-examine parts of this topic in Section 6.1.. 18.
(32) CHAPTER III. THE BIG PICTURE: RULE SCHEME AND PROCESS In this chapter we focus on devising an overall scheme of rules and models to detect Mandarin questions. Based on this scheme, subsequent chapters will then focus on mining features relevant to questions with a variety of technologies.. 3.1. Overall Strategy. To approach this task, the overall strategy adopted in this study is first trying to maximize recall and then to increase precision. In many applications recall and precision are two competitive goals. One target at one time makes the whole analysis process more focused, streamlined, and easier for performance tuning. Another advantage of this recall-first-precision-next route is that, as we progress, we may gain more insight into some facets of question, which may not be discussed in linguistic literature from the same angle or for the same coverage. If we perform a black-box machine learning procedure from the very beginning, we may miss this opportunity. Black-box procedures may also fail to integrate knowledge from a variety of heterogeneous datasets into a seamless model. With these ideas in mind, we outline the big picture of overall training and detection structure in Figure 1. Next we will describe the overall analysis and detection process. Levels of Analysis. Three levels of factors are considered in this study. Univariate analysis deals with single word feature, e.g., “Bó” (sh´enme; what) and “àS” (r´ uh´e ; how). Bivariate analysis deals with the patterns involving two words, e.g., the compound relative “Bó” + “·” case. Multivariate analysis deals with the syntactic patterns involving three or more words. 19.
(33) mining text. univariate rules passed through. filtered out linguistic datasets. bivariate rules passed through. filtered out. multivariate models filtered out detected as question. Figure 1: The big picture of overall training and detection structure Analysis Process. To achieve higher recall, we not only review linguistic literature but also re-examine relevant issues from a new quantitative and corpus point of view, in the hope that more comprehensive and precise features than before will be discovered. Therefore, we prefer the univariate analysis to be a white box rather than a black box. The next goal is to increase precision without hurting recall too much. As for bivariate analysis, there is still room for white box analysis. As for multivariate analysis, however, white box analysis is difficult since there are still many open issues in linguistics, let alone in NLP field. Therefore, multivariate analysis is done in a black-box approach using probability models. Detection Process. A sentence input is first analyzed by the univariate module. Since the goal of univariate module is to maximize recall, there may be many false positives. Therefore, both true and false positives will be sent to and re-analyzed by bivariate and then multivariate modules, during which more and more false positives will be filtered out so as to increase precision.. 20.
(34) <RuleSet >. ::= <Rule > ::= <PositiveAtom > ::= <ExclusiveAtom > ::= <PositivePosition > ::= | | | <ExclusivePosition > ::= | | <Regex > ::=. <Rule >+ +. ∗. . disjunction of rules . conjunction of atoms. <PositiveAtom > <NegativeAtom > ‘P’ <PositivePosition > <Regex > ‘N’ <ExclusivePosition > <Regex > ‘[’ . head ‘]’ . tail ‘x’ . don’t care ‘%’ . middle ‘<’ . before ‘>’ . after ‘x’ . don’t care <any legal Perl 5.8 regular expressions >. Figure 2: Grammar of question-detection rules at univariate and bivariate level. The quantifier symbol ‘∗’ attached to nonterminals means “zero or more,” and the symbol ‘+’ means “one or more”. 3.2. Syntax and Semantics of Rules. At lexico-syntactic level, Mandarin questions have a number of characteristics, according to what we have discussed in Section 1.3: . No reliable and decisive marker.. . No reliable and decisive word order.. In addition, previous studies on Mandarin questions are seldom in a corpusoriented approach. Therefore, they fail to identify more comprehensive and precise features. To perform univariate and bivariate analysis, we first define a specification for rule set as a basis for analysis. The syntax of detection rules is listed in Figure 2. The whole rule set <RuleSet > is a disjunction of a series of single rules. Since there are many exceptions in determining questions, each <Rule > is composed of a set of positive patterns <PositiveAtom > and exclusive patterns <ExclusiveAtom > if necessary. The test for a sentence by the rule is passed only when it matches every <PositiveAtom > and mismatches every <ExclusiveAtom >. A positive pattern may appear only in a specific place of the clause, while a exclusive pattern may. 21.
(35) 1 2 3 4 5 6 7 8. Construct plain rules using QRWs found in Chapter 5 while the result does not converge do Train the rules using the training set if the number of false negatives is not acceptable then Investigate if there is any missing feature if the number of false positives is not acceptable then Investigate if the rules are too general Merge similar rule patterns. Figure 3: Overall training process of question detection at univariate and bivariate stages only precede or procede it. Therefore, <PositiveAtom > and <ExclusiveAtom > have a <xxxPosition > field to specify this characteristic. To handle irregular morphological patterns, we devise the patterns around regular expressions. The advantage of regular expressions is that they make rules more concise and flexible. The disadvantages are that they may over-generalize the patterns and then decrease recall or precision. As for the syntax of regular expressions (or regex for short), we adopt the Perl 5.8 flavor [21] for its expressiveness and popularity. It is also considered the de facto standard in the industry that industrial-strength regex APIs or packages for other programming languages usually claim to be “Perl compatible” to one extent or another instead of compatible with POSIX’s flavor.. 3.3. The Training Process. In the first two stages (univariate and bivariate analysis), overall training process is iterative, as shown in Figure 3. Steps 5 and 7 are not entirely automatic since for now there remains many sophisticated facets to analyze further. For example, we discover in step 5 many subtle patterns not stated explicitly in linguistic literature before, such as the flexibility in the WH words and the lexeme “S” (h´e ; what). Due to the lack of quality machine-readable dictionaries, these patterns are hardly recognized correctly by machines. As for the multivariate analysis, we use probability model techniques to try to. 22.
(36) discriminate questions. We will discuss the details in Section 6.2.. 23.
(37) CHAPTER IV. DATASETS: CHOICES AND PREPROCESSING Since it is the first time to examine this topic in a quantitative approach, at this stage we intend to acquire as accurate knowledge about Mandarin question as possible. Therefore care must be taken to insure the quality of datasets. In this chapter we discuss the reason why these datasets are chosen as our starting point, the mismatch between these datasets and our research needs, and what have to be done in order to bridge the gap.. 4.1. The Corpus. The corpus used in this study is Academia Sinica balanced corpus of modern Chinese (or the Sinica corpus for short) developed by the Chinese Knowledge Information Processing Group (CKIP).1 It comprises about 5 million words, tagged with part-of-speech (POS) information and segmented according to the draft standard in Taiwan. Further details of the corpus can be found in [10]. Clauses are the basic analysis unit used in this study. The corpus divides every complex sentence into clauses that end with commas, periods, colons, semicolons, ellipses, exclamation, or question marks. There are 20,228 question clauses (2.70%) out of total 749,984 clauses. The register distribution of question clauses is listed in Table 2. If we look at the 4th column (qi /ai ), we may find that questions are more frequent in the oral forms than written, which is quite consistent n P with our intuition. If we look at the 5th column (qi / qi ), however, the corpus i=1 1. The latest public version of the Sinica corpus is 3.0, released on October 1997. Since then, there has been minor fixes on inconsistent formats, tagging, and data cleaning (e.g., about half of the file “t820902” was duplicated in the first release of version 3.0; this mistake was corrected in later revisions). The revision used in this study is dated April 19th , 2001.. 24.
(38) Table 2: Register distribution of question clauses in the Sinica corpus 3.0 n P Clause qi /ai qi / qi i=1. Register. Question: qi. Written Written to be read Written to be spoken Spoken Spoken to be written Unknown Total:. All: ai. (%). (%). 13,821 645,767 257 10,315 1,168 12,736 4,915 76,470 55 2,944 12 1,752. 2.14 2.49 9.17 6.43 1.87 0.68. 68.33 1.27 5.77 24.30 0.27 0.06. 20,228. 2.70. 100.00. 749,984. is biased severely toward the written forms. Therefore, our results may have the same bias, too. The choice of Sinica corpus, however, restricts us from fuller investigation. The most serious problem is that it is not a treebank. Since there is no hierarchical information available, we cannot handle properly question clauses embedded in complex or compound sentences. For convenience, we use the format “(file name : serial number of the clause)” to indicate where the quotation comes from. For example, “(ev7 : 121)” indicates that we quote the clause numbered 121 from the file “ev7” in the corpus.. 4.2. The Treebank. To investigate some issues in more detail (e.g., “person,” see Section 5.2.8), we refer to the CKIP Chinese treebank (or the Sinica treebank for short) as a source of syntactic and semantic information.2 The treebank, based on a subset of the Sinica corpus as raw material, is bracketed with syntactic hierarchies and annotated with semantic roles according to the information-based case grammar (ICG) developed by the same CKIP team. It currently comprises about 54,902 trees (as claimed on their Web site) and 290,144 words. Further details of the treebank can be found in [4, 5]. 2. The latest public version of the Sinica treebank is 2.1. It can be accessed on-line at http: //treebank.sinica.edu.tw/.. 25.
(39) It is a pity that the Sinica treebank removes punctuation marks altogether, including intra-clause punctuation (e.g., quotation marks and parentheses) and inter-clause punctuation (e.g., periods and commas), eliminating important clues for our research. There is no relevant annotation for us to infer from, either. As a result, we sometimes need to trace these trees back to their origins in the Sinica corpus. Take the following tree numbered 47397 for example, (14). S(theme:NP(predication:S *í(head:S(agent:NP(Head:Nhaa: 5)| Head:VC2: õ)|Head:DE: í))|evaluation:Dbb: 6|Head:V 11:u| range:NP(quantifier:DM: ¥_|Head:Nab: ~)|particle:Td: ý). Its origin in the Sinica corpus is as follows: (15). 5(Nh). õ(VC). í(DE). 6(D). ¥(Nep). _(Nf). ~(Na). ý(T). ?(QUESTIONCATEGORY). u(SHI). (ev7 : 121). It can be easily seen that two differences exist. The first is that they segment words differently: the treebank treats “¥_” as one word while the corpus two words. The second is that they assign parts of speech differently: The treebank uses a full form (e.g., “Nhaa” for “5” and “Dbb” for “6”) while the corpus a simplified form (e.g., “Nh” and “D”); the treebank tags the word “u” as “V 11” while the corpus tags it as a special “SHI” symbol. In case there may be still other differences, the backtracking procedure is performed solely at a level of Chinese characters rather than words as shown in Figure 4. Note that the regular expression pattern in line 9 is crafted this way in order to handle more complicated formats like the following tree numbered 914: (16). S(evaluation:Dbb: ˝§| <font color="#FF0000">agent:NP(Head:Nhaa: 5)</font>| epistemics:Dbaa: u|reason:Dj: 5ó| Head:VD1: }º|particle:Ta: í). 26.
(40) Let’s take a closer at this regular expression pattern. The last symbol “$” indicates that the whole pattern is to be matched at the end of the string u. The quantifier meta-character “*” means zero or more occurrences, “+” means one or more, and “?” means zero or one. A pair of parentheses “(” and “)” groups a series of characters and is also ready for field extraction. A pattern of the form “[ z1 z2 . . . zn ]” matches any single character in z1 , z2 , . . . , zn . On the contrary, a pattern of the form “[^ z1 z2 . . . zn ]” matches any single character except for z1 , z2 , . . . , zn . The backslash “\” is an escape character. Therefore, the first parenthesis group “([^:\)<]+)” says that it tries to match (and also extract the content of the underlined part, if successful) a non-empty string composed of any character except for the three symbols :. ). <. The next quantified. parenthesis group “(<[^>]+>)?” says that it tries to match an HTML tag, if any. With this carefully-crafted pattern, complicated trees such as Sentences (14) and (16) can be handled gracefully and neatly. To our surprise, we find in the backtracking process that the textual data of the treebank are not entirely a subset of the Sinica corpus; i.e., some sentences in the treebank are not extracted from the Sinica corpus but elsewhere. Take the tree numbered 39880 for example: (17). VP(Head:VK1: ı|goal:S(agent:NP(Head:Nhaa: g)| deontics:Dbab: ?|manner:Dh: ‚˛|deixis:Dbab: | Head:VC2: õõ)). The sentence cannot be found in the Sinica corpus. In consequence, some trees cannot be backtracked successfully to check if they are question clauses. These trees are excluded from this study for the sake of objectivity. Another treebank, also based on the Sinica corpus as raw material and furthermore annotated with HowNet semantic information, does contain punctuation and provide richer semantic information [22, 23]. It currently comprises 3,178 trees and about 36,000 words. However, the sample size is too small to be useful for this study: only 8 trees are relevant to questions! Therefore we do not use this 27.
(41) Algorithm: Finding the punctuation of a tree from the Sinica treebank by tracing its origin back to the Sinica corpus Input: a tree t in the Sinica treebank format Output: associated punctuation Begin: 1 Scorpus ← all clauses in the Sinica corpus, 2 with part-of-speech tags and delimiters removed 3 . Split the tree t into an array of fragments U 4 . using the vertical bar “|” as spliting points 5 U ← split(t, “|”) 6 . Extract Chinese characters from U to string r 7 for each u ∈ U do 8 . From the last “:” to the end (with optional “)” symbols) in u 9 Match u against the regex pattern “:([^:\)<]+)\)*(<[^>]+>)?$” 10 w ← the first field of match result (underlined part) 11 Append w to the end of r 12 for each s ∈ Scorpus do 13 if r in s do 14 return the last Chinese character (i.e., punctuation) of s 15 return not found Figure 4: Algorithm for finding the punctuation of a tree from the Sinica treebank by tracing its origin back to the Sinica corpus. As for the syntax of regular expressions (or regex for short), we adopt the Perl 5.8 flavor [21] for its expressiveness and popularity. 28.
(42) treebank for now.. 4.3. Machine-Readable Dictionaries. Lexical semantics have influence on the determination of questions, and therefore quality machine-readable dictionaries (MRDs) would be very helpful in mining such information automatically. In addition, quality dictionaries are proved by experts (often trained in a certain degree of corpus-based lexicography); research based on them may be more accurate than solely on corpora. The richer information an MRD has for defining and explaining words, the easier and more accurate our research will be. For instance, if an MRD tells us in plain language that the word “â” (g` uix`ıng; your last name) is “usually used in asking questions,” researchers may then try to write programs accordingly to extract such clues. Furthermore, if the MRD is compiled from a modern linguistic perspective, the word may be annotated with more detailed syntactic or pragmatic information in a consistent format for ease of automated processing. For instance, the WordNet [20] (though it only focuses on the English language) annotates the word “why” with “question word,” thus simplifying automated search for such interrogative expressions. Mandarin dictionaries are seldom compiled with a modern lexicology perspective in mind, let alone Mandarin MRDs. The treatment of morphology and pragmatics is severely neglected [13, pp. 3–6]. We choose the on-line installation of the ABC Chinese-English Dictionary [15], under the umbrella of the Academia Sinica Bilingual Ontological Wordnet project (or the Sinica BOW for short),3 as our primary MRD resource. The dictionary, though claimed to comprise about 60,400 words, makes only 32,691 words publicly accessible on the BOW browsing frontend (the other half may actually be on the BOW server too, but inaccessible through the dynamic pages exposed to Web browsers). The search interface at the frontend is not user-friendly—no wildcard search at all! As a result we have 3. The service can be accessed on-line at http://bow.sinica.edu.tw/.. 29.
(43) to write programs to gather page by page the list of words accessible, and then use this list to perform further search. The dictionary translates Chinese words and idioms into equivalent English words or phrases. Although it is quite simple that no pronunciation, examples, usage notes, etc. is available, it does provide one important clue for this study: question marks. Take the words “Sv” (h´esh´ı) and “SJ” (h´eyˇı) for example, they are translated by the dictionary as “when?” and “how?; why?” respectively (notice the question marks). Given this feature, we can write programs to gather all translation entries containing the question marks as our starting point. In this way, there are totally 37 words found to be related to questions, if part-of-speech is also considered. The disadvantage of this dictionary is that its coverage of words is too small, compared to CKIP’s Chinese Electronic Dictionary (about 80,000 words) or even open lexicons such as libtabe (about 137,000 words; see [26]) and EZ Input big lexicon (about 100,000 words; see [18]).4 The larger a lexicon is, the better chance we may have to extract useful information. The Sinica BOW provides other machine-readable dictionaries as well, but they are too small in size, in under-construction or restricted-use state (e.g., CKIP’s Chinese Electronic Dictionary and Lyu’s Eight Hundred Words of Modern Mandarin) and/or not qualified enough for this kind of linguistic research (e.g., MOE’s Mandarin Dictionary Revised ). Therefore they are excluded from this study. Among them, the MOE’s Mandarin Dictionary Revised is worth a closer look. In fact, the dictionary service at BOW makes use of merely a subset of the original database. The official site for this dictionary [40] provides much larger coverage (about 166,193 words at present), richer information, and better search interface than the subset one installed at BOW. Compiled from a more traditional lexicography perspective, it provides no modern tagging or annotation system and 4. Dr. Tsai compiles a list of lexicons available on the Internet [49]. Most of them are free or licensed as open source software. Not for linguistic purpose, though, they can still give us a rough estimate of appropriate coverage a practical Mandarin lexicon should have.. 30.
(44) therefore is not easy to analyze automatically. That said, it does contain something useful for this study. It is therefore chosen as the auxiliary MRD in this study.5 In conclusion, the primary MRD in this study is the on-line installation of the ABC Chinese-English Dictionary at Sinica BOW site, and the auxiliary MRD is the official site for MOE’s Mandarin Dictionary Revised.. 4.4. Other Non-Electronic Resources. Sometimes it is inevitable to consult more comprehensive resources other than electronic ones about some linguistic issues. For example, we use the Unabridged Mandarin Dictionary [36], Unabridged Dictionary of Chinese Characters [59], and Eight Hundred Words of Modern Mandarin [38] to explore more similar cases for a certain kind of lexical semantics. Since they are not in electronic forms, exhaustive search is impossible unless plenty of labor is available.. 5. The examples in this dictionary were once considered as another source of corpus for this study. But too many quotations from ancient classics make them inappropriate here.. 31.
(45) CHAPTER V. UNIVARIATE ANALYSIS Our overall machine learning strategy is first trying to increase recall and then precision, as has been stated in Chapter 3. To maximize recall, we need to discover all features that may constitute a question. In Chapter 2 we have reviewed linguistic literature on Mandarin question forms, but the literature does not stand on a corpus and statistical basis. To be useful in statistical NLP methodology, however, a quantitative investigation is necessary. Another reason to conduct a quantitative survey is that the features listed in literature are neither comprehensive nor precise enough for NLP purpose. In this chapter, therefore, we re-examine several issues in quantitative point of view. It should be noted that the main purpose is to pave the way for devising programmable rules and heuristics. The fuller linguistic and qualitative study of them is beyond the scope of this research.. 5.1. Finding Question-Related Words. As a beginning, we will examine what set of words constitutes a question sentence in a somewhat context-free manner. These “question-related words” (hereafter, QRWs) may be content words or particles. We coin the term “QRW” in order to avoid confusion with another term used frequently in linguistic literature: “question words” [30, 11], which should mean the interrogative words or WH-words (e.g., what, which, who). The set of Mandarin interrogative words is therefore a subset of QRWs. 5.1.1. Procedure. To find QRWs in a quantitative approach, the question-delection problem should be modeled first as a statistical form suitable for identifying and ranking univariate features. Since they are categorical variables, we model the problem as a statistical 32.
(46) problem: test-of-independence of two dimensions of factors. One dimension is whether a word wi under consideration is in a sentence sj under consideration, and the other is whether the sentence sj is a question. Modeled in this way, the word wi = “Bó” (sh´enme; what) may have the following four cases: (18). a. wi is in a question sentence ƒ(D) Bó(Nep). n(Da). u(VG). ‘X¹(Na) ?. b. wi is in a non-question sentence Ì(Cbb). êÞ(VJ). 7(Di) Bó(Nep). ×(VH). 9(Na) ,. c. wi is not in a question sentence 5(Nh). ó](VK). ý(T) ?. d. wi is not in a non-question sentence y(D). ×;(VH). 6(D). Ìà(VH) . Based on these four observations, one may undertake statistical inference procedures to test if and to what degree wi is independent of questions. To undertake any statistical inference, one needs to calculate, for each wi candidate in the corpus, the number of occurrence of the four cases in sentence (18), and they can be arranged in a 2 × 2 contingency table (see Table 3) with 4 cells a, b, c, and d. The algorithm in Figure 5 will then generate the four variables, undertake statistical inference, and rank the results. Lines 1–2 initialize the unordered sets SQ and SNQ to store all question and non-question clauses, respectively. Line 3 initializes the unordered set W to hold all QRW candidates. The main loop of the algorithm in lines 4–12 iterates through each word wi ∈ W to compute its statistic. The framework is so general that a variety of statistical procedures can be applied. Here we apply two kinds of test procedures which have solid mathematical foundation in the field of inferential statistics. Regarding the two procedures for asymptotic χ2 distribution, some state that the log-likelihood ratio (LLR for short) is better at sparse data [17] while others state that the Pearson’s chi-square (χ2 ) test is better at smaller n and more sparse tables (for literature review for this 33.
(47) Table 3: 2 × 2 contingency table for finding question-related words (QRWs) Is wi in the clause? Clauses. Yes. No. Ends with ‘?’ Ends without ‘?’. a c. b d. where intermediate values:. wi ∈ {all words in the corpus}. n ma mb mc md and final statistics of wi :. = = = = =. a+b+c+d (a + b)(a + c) (a + b)(b + d) (a + c)(c + d) (b + d)(c + d). LLR statistic = 2 ×. d X. j ln. j=a. n×j mj. n(ad − bc)2 ma md Frequency = a + c Precision = a/(a + c) Recall = a/(a + b). χ2 statistic =. Algorithm: Finding question-related words from the corpus Input: corpus Output: associative array C[w1 , . . . , wn ] mapping from wi to statistic of interest, where n = |W| and i = 1, . . . , n Begin: 1 SQ ← all question clauses in the corpus 2 SNQ ← all non-question clauses in the corpus 3 W ← all unique words in SQ 4 for each wi ∈ W do 5 a, b, c, d ← 0 6 for each s ∈ SQ do 7 if wi in s then ++a 8 else ++b 9 for each t ∈ SNQ do 10 if wi in t then ++c 11 else ++d 12 C[wi ] ← compute statistic of interest for wi via a, b, c, d 13 Sort C in descending order Figure 5: Algorithm for finding question-related words 34.
(48) point, see [1, pp. 24, 395–397]). For completeness and comparison, both are used in this study. It has been reported in [46, 55] that Fisher’s exact test for this task is better at dealing with sparse data than some other ways to approximate theoretical χ2 distribution such as Pearson’s χ2 test or LLR test. However, it requires a lot of computation in hypergeometric space and factorials, especially as large as factorial 749,887: def. Θ = set of configuration from this to the most extreme case X (a + b)! (a + c)! (b + d)! (c + d)! P (wi ) = a! b! c! d! n! Θ X (a + b)! (a + c)! (b + d)! (c + d)! P1 (wi ) = since n! remains constant a! b! c! d! Θ Even with the help of Stirling’s formula: x! ∼ =. √ √. 2π xx+0.5 e−x. for x large. 2π e(x+0.5) ln x−x. it is still too large for a long double floating point to handle. It is therefore not very practical here. For brevity, top 40 results are listed here in Table 4, and more details can be found in Appendix A. There are some disagreements about the rankings and statistics in the two tests. Looking at the comparison chart in Figure 6, however, the overall trend remains the same, and converges when the ranking is greater than about 1000. The correlation coefficient r = +0.9919 in Figure 6b further suggests a very strong association between both ranking schemes. Therefore, we will refer to the ranking in terms of LLR unless mentioned explicitly. At first glance both the statistics for LLR and χ2 in Table 4 seems too large. The reason is that, given a wi , the “No” column in Table 3 may contain something that acts similar to the “Yes” column; such lurking variables have side effects that falsely magnify the statistic. Therefore, a higher χ2 critical value (i.e., a lower Type 35.
(49) 100,000.00 LLR. Statistic in log scale. 10,000.00. Chi-Square. 1,000.00. 100.00. 10.00. 1.00. 0.10. 0.01 0. 200. 400. 600. 800. 1000. 1200. Word ranking in terms of LLR. (a) Ranking versus statistics of LLR and Chi-Square. 1,200 regression line: y = 0.9919 x + 4.894 r2 = 0.9838. Chi-Square ranking. 1,000. 800. 600. 400. 200. 0 0. 200. 400. 600. 800. 1,000. 1,200. LLR ranking. (b) Ranking of LLR versus Chi-Square. Figure 6: Comparison between LLR and Pearson’s χ2 tests on QRWs. In (a) the X-axis is arranged in terms of LLR ranking. The Y-axis shows statistics of LLR and χ2 respectively in logarithmic scale. Here the statistics of Pearson’s χ2 test tend to be larger than that of LLR, but converge in the long run. In (b) both axes are arranged in terms of LLR and χ2 respectively. The dashed regression line and a correlation coefficient r = +0.9919 suggest a very strong association between the two rankings 36.
(50) Table 4: Top 40 question-related words (QRWs) found by statistical inference procedures Ranking LLR. 2. QRW. χ. wi. 1 2 3 4 5 6 7 8 9 10. 1 2 3 4 6 5 13 7 10 8. ý(T) á(T) Bó(Nep) ÑBó(D) 5(Nh) 5ó(D) .(D) Õ(Nh) àS(D) ƒ(D). 11 12 13 14 15 16 17 18 19 20. 14 9 12 33 11 15 16 17 18 19. u´(D) 5óŸ(VH) 5óš(VH) u(SHI) Ø−(D) ¨(Nep) S(Nes) ¨³(Ncd) ³(D) ˝§(D). Statistic. Ranking 2. LLR. 17,956.52 17,798.44 11,223.98 6,163.42 5,464.47 4,776.90 3,320.25 2,685.79 2,548.63 1,998.58. 88,941.79 72,731.19 37,515.11 26,622.68 11,060.08 18,747.55 4,982.97 9,161.25 7,369.35 8,221.60. 1,653.17 1,549.34 1,454.17 1,342.47 1,265.77 1,226.55 1,154.87 1,033.09 973.16 944.68. 4,540.90 7,372.23 5,464.69 1,605.50 5,861.16 4,376.40 4,307.55 4,086.19 3,959.01 3,554.16. LLR. χ. 2. QRW. Statistic LLR. χ2. 5b(Nh) ß(T) í(DE) ÑS(D) ø−(VK) àS(VH) }(D) }.}(D) Öý(Neqa) ¢(D). 915.39 851.01 813.92 807.60 772.87 772.15 741.07 711.35 709.86 702.45. 2,173.73 1,919.64 772.95 3,003.16 1,404.77 2,857.26 1,057.46 2,774.36 1,844.61 1,097.02. ´(D) g(Nh) ´u(Caa) š(Nf) v(D) ú(VH) ](Nh) b(D) 5ó(VH) Ê(P). 691.14 658.02 652.50 613.09 609.59 581.03 580.09 564.10 517.82 482.15. 1,036.92 1,558.61 2,169.88 1,808.57 1,471.49 1,107.65 1,223.44 761.19 2,169.03 402.42. χ. wi. 21 22 23 24 25 26 27 28 29 30. 23 28 59 20 40 21 49 22 29 48. 31 32 33 34 35 36 37 38 39 40. 50 37 24 31 38 47 43 61 25 76. I error probability α) is required to claim that the result is significant. However, the raw statistic is not important at this stage, and we only refer to the statistic in terms of rankings in this section.1 5.1.2. Coverage Test in Terms of Recall. Before going any further, we would like to stop for a while to validate the validity of these QRWs in two ways: one is by quantitative analysis inside the corpus itself, and the other is by the MRD and a little qualitative analysis. First, we would like to verify if these QRWs (especially those with top ranking) really cover most of the question cases in the corpus. To do this, let’s examine them in terms of recall. We use the procedure outlined in Figure 7 to calculate cumulative recall of these QRWs in ascending order of their ranking; the result is shown in Figure 8. 1. Note that in many NLP applications, as Manning and Sch¨ utze [41, p. 166] pointed out, “the level of significance itself is less useful . . . All that is used is the scores and the resulting ranking.”. 37.
(51) Algorithm: Calculating cumulative recall and precision of QRWs Input: corpus Output: cumulative recall and precision for Wi , i = 1, . . . , n def Def: wi = the QRW ranked i-th Wi ≡ {w1 , . . . , wi } Begin: 1 SQ ← all question clauses in the corpus 2 SNQ ← all non-question clauses in the corpus 3 for i = 1, . . . , n do 4 a, b, c, d ← 0 5 for each s ∈ SQ do 6 if s contains any word in Wi then ++a 7 else ++b 8 for each t ∈ SNQ do 9 if t contains any word in Wi then ++c 10 else ++d 11 Compute and print recall and precision for Wi Figure 7: Algorithm for calculating cumulative recall and precision of QRWs. 100% 90% 80%. Cumulative value. 70% 60% recall 50%. precision. 40% 30% 20% 10% 0% 1. 26. 51. 76. 101. 126. 151. 176. 201. 226. 251. 276. QRW ranking in terms of LLR. Figure 8: Cumulative recall and precision of question-related words. 38. 301.
數據
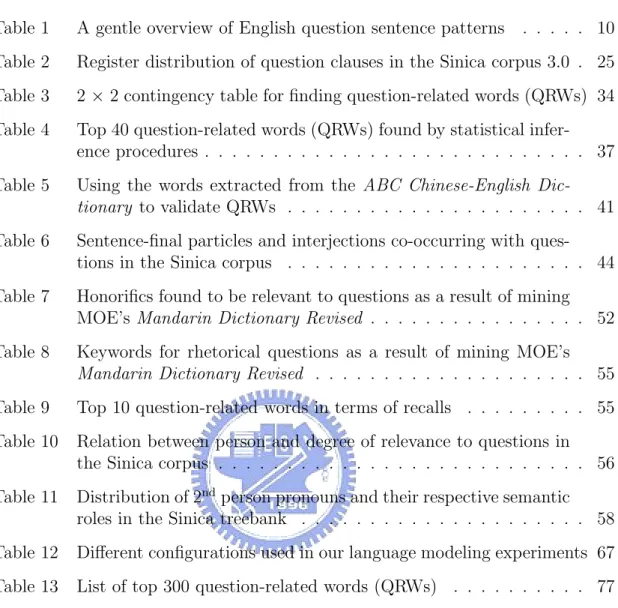
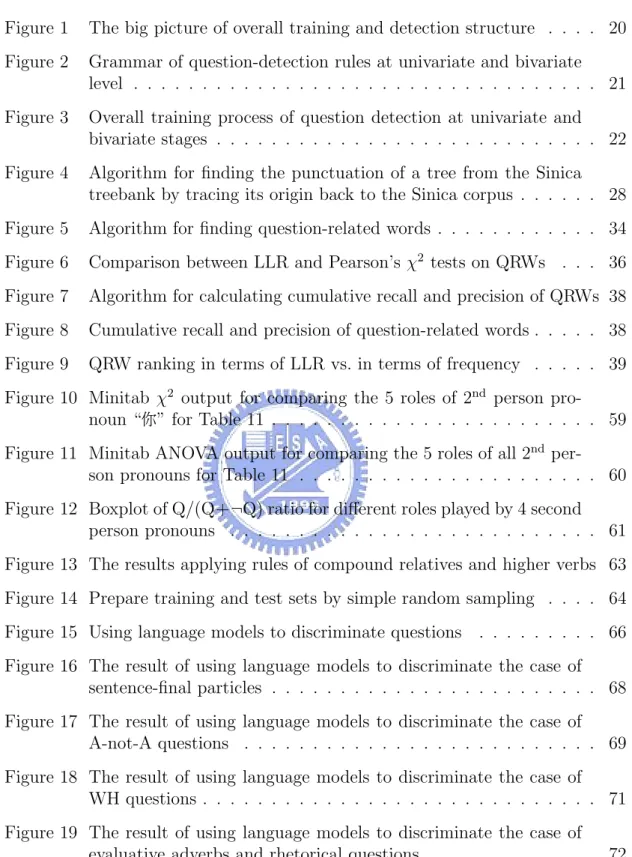
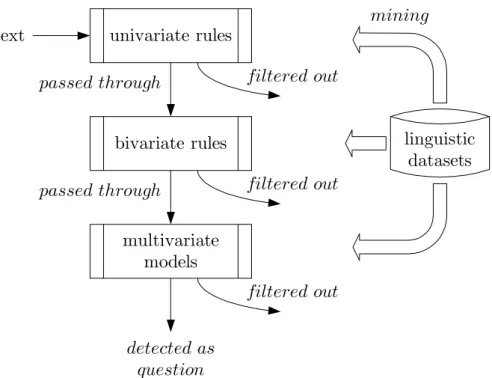
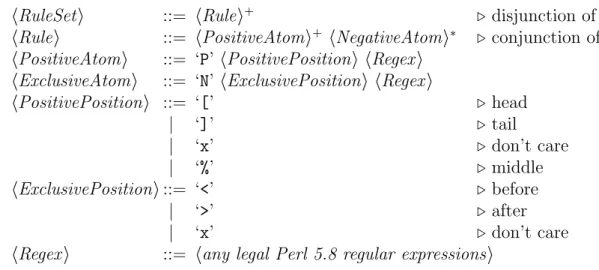
相關文件
Reading Task 6: Genre Structure and Language Features. • Now let’s look at how language features (e.g. sentence patterns) are connected to the structure
Guiding students to analyse the language features and the rhetorical structure of the text in relation to its purpose along the genre egg model for content
Wang, Solving pseudomonotone variational inequalities and pseudocon- vex optimization problems using the projection neural network, IEEE Transactions on Neural Networks 17
volume suppressed mass: (TeV) 2 /M P ∼ 10 −4 eV → mm range can be experimentally tested for any number of extra dimensions - Light U(1) gauge bosons: no derivative couplings. =>
QCD Soft Wall Model for the scalar scalar & & vector vector glueballs glueballs
Define instead the imaginary.. potential, magnetic field, lattice…) Dirac-BdG Hamiltonian:. with small, and matrix
incapable to extract any quantities from QCD, nor to tackle the most interesting physics, namely, the spontaneously chiral symmetry breaking and the color confinement..
• Formation of massive primordial stars as origin of objects in the early universe. • Supernova explosions might be visible to the most