台灣地區金融市場波動性實證研究
全文
(2) 台灣地區金融市場波動性實證研究 Empirical Performance of Financial Market Volatility in Taiwan. 研 究 生:侯姿羽. Student: Tzu-Yu Hou. 指導教授:李昭勝 博士指教授. Advisors: Dr. Jack. C. Lee. 謝國文 博士. Dr. Gwowen Shieh. 國 立 交 通 大 學 管 理 科 學 系 碩 士 論 文. A Thesis Submitted to Department of Management Science National Chiao Tung University in partial Fulfillment of the Requirements for the Degree of Master in Management Science June 2004 Hsinchu, Taiwan, Republic of China. 中 華 民 國 九 十 三 年 六 月.
(3) Empirical Performance of Financial Market Volatility in Taiwan Student: Tzu-Yu Hou. 指教授. Advisors: Dr. Jack. C. Lee Dr. Gwowen Shieh. Department of Management Science National Chiao Tung University. Abstract Forecasting volatility plays an important role in financial market, because volatility predictions are crucial for the successful implementation of risk management. The use of high frequency data approximately renders volatility from a latent to an observable quantity, and opens the new field of visions to forecast future volatilities. Use of realized volatility constructed from high-frequency intraday returns, in contrast, permits the use of traditional time-series methods for modeling and forecasting. Main goals of this thesis are to find general and powerful forecasting procedures for volatilities based on Taiwan high frequency data, to evaluate the predictive potential of volatility forecasts for the true latent volatility, to analyze the impact of more reliable volatility predictions on the quality of three widely used risk measures, and to test for parameter changes in the GARCH(1,1) models. For that purpose, this thesis explores some statistical models for predicting the daily volatility of financial time series.. Keywords: change point, GARCH (1,1) models, realized volatility. i.
(4) 台灣地區金融市場波動性實證研究. 學生:侯姿羽. 指導教授:李昭勝 博士 指導教授:謝國文 博士. 國立交通大學管理科學研究所碩士班. 摘要 預測金融市場的波動性(volatility)非常重要,因為能否準確的 預測波動對於風險管理的成敗扮演著關鍵性的角色。波動性是無法由 金融市場所提供的資訊得知,然而我們可使用頻繁交易的資料來表示 此一無法觀測的值,這也開啟了預測未來波動性的新視野。我們利用 以日內報酬所得到的 realized volatility 當作真實波動以與時間序 列的方法做出的模型做比較。本論文主要的目的是──以台灣的資料 找出能有效預測波動性之模型、計算真實的波動性以用來代表無法直 接觀測到的波動性、以三種不同的方式分析出最可靠的波動預測模 型、並測試出何時 GARCH(1,1)模型需要重新估計參數。為此,本文 利用一些統計中的方法和時間序列模型去預測金融市場的日波動。. ii.
(5) 誌謝. 很高興能有機會到交大來唸書,光陰似箭,轉眼間兩年的碩士班 將告一個段落,在研究生涯將結束的前夕,不能免俗的,要感謝很多 人。 碩士論文能夠順利完成,首先要感謝的是我的指導教授李昭勝博 士,非常感謝老師不厭其煩的悉心指教﹔還有博士班學長牛維方,不 管在論文或未來求職的路上都毫不保留的給我很多意見﹔當然我一 定得感激父母、大舅、三舅以及兩個哥哥(冠羽、翔仁)從小至今的 支持跟鼓勵,少了他們各方面的幫助,就沒有今天的我。 在這兩年中,有研究所同學(佩雯、映均、淑芬、小鳳、柏蓁、 張簡、坤岐、雅雯)以及好友(寶文、雨蓓、玉林、Karen、Tim)、 秋燕、室友怡芬等等的陪伴與幫忙下,讓我的研究生涯更加多采多 姿,你們的關心給我無比的動力,能夠認識你們我真的感到很榮幸。 碩士生活將結束,同時也代表著另一個階段的開始,我會好好努 力,不辜負大家的期望,再次感謝您們!.
(6) Contents Abstract ....................................................................................................... i Abstract in Chinese .................................................................................... ii Contents .................................................................................................... iii List of Tables............................................................................................. iv List of Figures .............................................................................................v 1. Introduction..........................................................................................1 2. Data ......................................................................................................3 2-1 TAIEX..............................................................................................................3 2-2 TXO .................................................................................................................3. 3. Volatility Notations and Illustration of Models ...................................4 3.1 General Autoregressive Conditional Heteroscedastic (GARCH) model.........5 3.2 Realized Volatility ...........................................................................................6 3.3 Change Point Detection with Methods in Quality Control..............................7 3.4 Likelihood Ratio Test (LRT)............................................................................9. 4. Evaluating Volatility Measures ..........................................................11 5. Empirical Analysis.............................................................................13 5-1 Empirical Volatility Measurement.................................................................13 5-2 Some issues of Interest ..................................................................................16. 6. Conclusion .........................................................................................18 Reference ..................................................................................................19 Tables ........................................................................................................22 Figures.......................................................................................................27. iii.
(7) List of Tables Table 2.1 TAIEX option............................................................................22 Table 5.1 Candidates of change points by method 2 ................................24 Table 5.2 Candidates of change points by method 3 ................................24 Table 5.3 Candidates of change points by method 4 ................................24 Table 5.4 Parameter estimates of GARCH (1,1) model ...........................25 Table 5.5 The calculations of accuracy measures in every model............25 Table 5.6 Some statistics of standardized returns.....................................25 Table 5.7 The Ljung-Box Q-Statistic........................................................26. iv.
(8) List of Figures Figure 2.1 The SARS daily probable cases happened in Taiwan from Feb. 25, 2003 to Sep. 4, 2003 .......................................................27 Figure 2.2 Stock prices .............................................................................28 Figure 3.1 Realized volatility of TAIEX during Jan.2, 2003 to Aug. 29, 2003 with 5-min intraday returns. ........................................29 Figure 5.1 Log returns under 6 sigma criterion ........................................30 Figure 5.2 Log returns from Jan. 2, 2003 to Aug. 29, 2003 .....................31 Figure 5.3.1 QQ-plots of GARCH(1,1) models versus standard normal distribution ............................................................................32 Figure 5.3.2 QQ-plot of realized volatility versus standard normal distribution ............................................................................33. v.
(9) 1. Introduction The explosive growth of applications of econometrics to finance is due primarily to the increased availability of financial data, increased computer power and the greater interest in the performance of financial markets in current economic discussions. Asset returns volatility is a central feature of many financial market problems such as risk management and option pricing. However, it is still an ambiguous term because of different concepts and definitions. Up to now, many literatures have focused on the parametric approach considering volatility as an unobservable variable and using a fully specified functional model for the ex-ante expected volatility. Modeling the volatility was one of the most common topics in the financial literature by using all ARCH types models and stochastic volatility models. With respect to financial econometrics, Bollerslev (2001) declares that the development of ARCH has been one of the two most important developments in this field over the past two decades. However, realized volatility affords the empirical measurement of the latent notional volatility on the discrete time interval [t-h, t]. Similar to the instantaneous volatility measures, realized volatilities may be classified according to whether the estimation of the notional volatility only uses returns observations falling in the interval [t-h, t], which we call “local”, or also incorporates returns outside [t-h, t]. The most obvious local measure for daily volatility is the daily absolute return. More recently a series of papers (Andersen, Bollerslev, Diebold and Labys 2001a and Barndoff-Nielsen and Shepard 2002a, b, c) has formalized and generalized this intuition by applying the quadratic variation theory to the broad class of special (finite mean) semi-martingales. To make clear the purpose and main theme from the very beginning, the main 1.
(10) goal of this thesis is to find general and powerful forecasting models for volatilities based on high frequency data. Particularly, we will use Taiwan high frequency data to fit in-sample volatility, and to find more reliable volatility predictions. The problem of testing for a parameter change has been an important issue in statistics. It started in the quality control context and then moved rapidly to other fields. Finally, we will check whether the results (our expected volatilities) are consistent with the actual volatilities (realized volatility). This will be a powerful method to examine our well-done forecasting models. The change point issue has attracted much attention from lots of researches in time series analysis. In the thesis, we applied some tests to a real data set. Since the data set suffered from a period of SARS, it may detect structural changes owing to changes of events or policy. Ignoring it can lead to a false prediction. The rest of the thesis proceeds as follows: In Chapter 2, we describe data types. Chapter 3 establishes every volatility notation and illustrates some models we used in this thesis. Particularly, there are some special methods to detect structural change. Chapter 4 reports some definitions of evaluating volatility measures and uses these measures to assess performance of each model theoretically. In Chapter 5, we try to summarize empirical analysis, and propose some interesting issues. Chapter 6 concludes the discussion.. 2.
(11) 2. Data The data used in this thesis are indices of the Taiwan Stock Exchange (TAIEX)1 and the Taiwan Stock Exchange option (TXO) obtained for the period from Jan. 2, 2003 to Aug. 29, 2003, and 163 days in the aggregate. We chose this period because it was the period of initial and terminal stages when epidemic SARS spread globally. Figure 2.1 shows the daily probable amount of SARS cases happened in Taiwan from Feb. 25, 2003 to Sep. 4, 2003. According to Figure 2.1, epidemic SARS reached the high summit during the period from the middle of April to the middle of May. It means that high level infection of SARS coronaviruses lasted for more than one month.. 2.1 TAIEX TAIEX is the comprehensive index of all companies entered the market in Taiwan Stock Exchange Corporation, and the method to calculate depends on market value of weighted-average. Taiwan Stock Exchange Corporation provided data banks of the index TAIEX since Aug. 8, 1998. There are three types of indices in calculating methods: price-weighted, value-weighted, and equal-weighted. TAIEX belongs to type two and is common in global financial markets. Value-weighted is calculated by total market value in the current period (outstanding shares* closed stock price)/total market value of base period. Therefore, at the same level of advance-decline, companies with great market value have much more influence than that with small ones. __________________________ 1. At present, the most commonly used index is Taiwan weighted index. It includes more. than six hundreds stocks of listed companies in Taiwan, and it is also the most famous index in Republic of China. The code of Taiwan weighted index in quote systems is TWII.. 3.
(12) The plot of stock prices from Feb. 25, 2003 to Sep. 4, 2003 is given in Figure 2.2.. 2.2 TXO. Option of Taiwan Stock Exchange Capitalization Weighted Stock Index (TXO) is a financial derivative started on Dec. 24, 2001.This option is European style, thus there is no possibility of early exercise. Trading hours are 08:45AM - 1:45 PM Taiwan time Monday through Friday of the regular Taiwan Stock Exchange business days. This option product belonged to the new financial derivative, so people were not familiar with it at beginning. However, many stock trading companies tried to promote TXO. Therefore, it is quite popular today. We can see the detailed description in Table 2.1.. 4.
(13) 3. Volatility Notations and Illustration of Models A special feature of stock volatility is the fact that it is not directly observable. For example, consider the daily logarithmic rate of returns of Taiwan Stock Exchange Capitalization Weighted Stock Index (TAIEX). The daily volatility is not directly observable from the returns because there is only one observation in a trading day. If intraday data of the stock, such as 5-minute returns, are available, then one can estimate the daily volatility by realized volatility. The accuracy of such an estimate deserves a careful study. Throughout this thesis, we try to do research in volatility. We will first start with theoretical illustration of GARCH model that has been popular in financial time series analysis.. 3.1 General Autoregressive Conditional Heteroscedastic (GARCH) Model. We assume there is an asset guaranteeing an instantaneously risk-free rate of interest. We have the following characterization of the logarithmic asset price, pt , where 0 ≤ t ≤ T . The continuously compounded return over the time interval [t − h, t ] is rt ,h = pt − pt − h ,. 0≤ h≤t ≤T. (3.1). or is directly written as rt ,h = log(. pt ). pt − h. (3.2). The discrete-time models, at a minimum, assume that the correct specification of the h-step ahead conditional mean and variances are known up to a low-dimensional parameter vector.. 5.
(14) The general autoregressive conditional heteroscedastic model, in brief, GARCH(m,s) model (Bollerslev, 1986), can be expressed as follows, m. s. σ 2t ,h = α 0 + ∑ α j [rt − j⋅h,h − µt − j⋅h ,h ]2 + ∑ β iσ 2t −i⋅h,h , j =1. i =1. ≡ α 0 + α ( L, h)[rt , h − µt , h ] + β i ( L, h)σ 2. where α 0 > 0 , α i ≥ 0, for i > 0 , β 0 ≥ 0 ,. ∑. max( m , s ) i =1. 2 t ,h. (3.3). ,. (α i + β i ) < 1 , and L stands for lag. operator. For example, L(rt ) = rt −1 .. In most applications, only lower order GARCH models are used frequently, such as GARCH(1,1), GARCH(2,1), and GARCH(1,2) models. We will use the GARCH(1,1) process of Duan(1995) for daily returns in this thesis. The GARCH(1,1) model lists as follows, 1 rt = r + λ ht − ht + at , at Ft −1 ~ N (0, ht ), 2 2 ht = σ t = α 0 + α1at2−1 + β1σ t2−1 ,. (3.4). where α 0 > 0, α1 ≥ 0, β1 ≥ 0 . We use the maximum likelihood (ML) method to estimate the parameters α 0 ,α1 , β1 , λ , where λ is the constant unit risk premium. (Under conditional lognormality, one plus the conditionally expected rate of return equals exp(r + λ ht ) ).. 3.2 Realized Volatility. Andersen et al.(2001a, b) propose the sum of squared returns daily realized volatility estimator which sums the squares of intraday returns. Let rt ,i , where 0 ≤ i ≤ n , represent a set of n+1 intraday returns for day t, and when i = 1 represents the five minutes commencing at the open, and concluding with 6.
(15) the five minutes at the end when i = n . The realized volatility for trading day t, from the close on day t-1 to the close on day t, is defined by n. σˆ 2t = ∑ rt,2i ,. (3.5). i =0. where 0 ≤ i ≤ n . The realized volatility is simply the second sample moment of the log return process over a fixed interval. We use a sampling frequency of 5-minute returns, which is high enough such that our daily realized volatilities are largely free of measurement error. We show in Figure 3.1.. 3.3 Change Point Detection with Methods in Quality Control. In 2003, the most influential event globally is SARS, especially in Asia. The peak period of the high infectious disease is from April to June. Consequently, we must pay attention to the event. However, if we ignore structural change on purpose, in other words, do not readjust the estimation of all parameters in time series models, it is not a reasonable forecasting at all. For this reason, we hope that methods in quality control can help detect some change points. We can then cut a complete period into several subperiods in accordance with these change points. In order to ensure the candidates of change points to be accepted statistically, we will utilize the likelihood ratio test for this purpose in the next section. Once the change points are identified, we estimate parameters and calculate volatility for each subperiod.. Quality control plays an important role in industries of manufacturing. Quality control is a process that measures output relative to a standard, and take action when. 7.
(16) output does not meet the standard. A control chart is essentially a picture of a sampling distribution. That is, it consists of a series of sample values or “statistics” which, if they were gathered together instead of being plotted in sequence, would form a distribution. A natural pattern has three characteristics simultaneously. They can be summarized as follows: 1. Most of the points are near the solid centerline. 2. A few of the points spread out and approach the control limits. 3. None of the points (or at least only a very rare and occasional point) exceeds the control limits. If any characteristic stated above is missing, the pattern will look unnatural. A control chart may indicate out of statistical control either when one or more points fall beyond the control limits or when the plotted points exhibit some nonrandom patterns of behavior. The Western Electric Handbook (1956) suggests several decision rules for detecting nonrandom points on control charts. It shows that the unnatural consecutive pattern is out of control if there is any one listed below: 1. One point plots outside the 3 sigma control limits; 2. Two out of three consecutive points plot between a distance of 2 sigma and 3 sigma; 3. Ten out of eleven consecutive points plot on one side of the centerline; 4. A run2 of five consecutive points plots on one side of the centerline; or 5. Five consecutive points are in a rising or falling trend.. __________________________ 2. A run is a sequence of observations with a certain characteristic. 8.
(17) Under models with Markov properties, each innovation of a financial time series can be treated as independent. Therefore, it is natural to assume that quality control may be a good tool to find nonrandom and unnatural points. We can use it to find candidates of change points, which will be the subject of the next section.. 3.4 Likelihood Ratio Test (LRT). After using several methods of quality control, we can find some candidates of change points. If the Xth day is a candidate, the interval between the first day and the (X-1)st day is period one and interval between the Xth day and the nth day is period two. Consider the following testing hypothesis problem. H0:period one and period two have the same data structure, H1:period one and period two have different data structures.. For a GARCH(1,1) model, the likelihood function (L) is given by T. L=∏ t =1. −( r − µ ) 2 1 exp[ t 2 t ] . 2σ t 2πσ t. (3.6). Reject H0 if. λ = −2 log[. L( period1 + period 2) 2 ] > χ 4,1 − 0.05 = 9.488 , L( period1) L( period 2). (3.7). 2 where χ 4,1 − 0.05 is the upper 100(1 − 0.05)% point of the chi-square distribution with. 4 degrees of freedom3. __________________________ 3. The degree of freedom is the amount of difference between parameters under H0 and. H1. For instance, there are 4 parameters under H0 and 8 parameters under H1 in the GARCH(1,1) model. Thus, the degree of freedom here equals to 4. 9.
(18) When it comes to the end of hypothesis testing, we can conclude whether the candidates are change points or not from the LRT. Once the change points are established, we estimate the parameters of the GARCH(1,1) model in each subperiod.. 10.
(19) 4. Evaluating Volatility Measures It is almost impossible to describe an evaluation criterion that is commonly acceptable. However, given our objective of measuring the general degree of predictability of the volatility theoretically and practically, we will avoid using very sophisticated evaluation criteria. Hence, we will stay with the statistical tradition of reporting summary statistics based directly on the differences between forecasts and realizations.. Standard accuracy measures. Three criteria are used here to evaluate the accuracy of the forecasts: root mean squared error (RMSE), mean absolute error (MAE) and logarithmic error (LE). Mean squared error provides a quadratic loss function which disproportionately weights large forecast errors more heavily relative to mean absolute error, and hence the latter (MAE) may be particularly useful in forecasting situations when large forecast errors are disproportionately more serious than small errors. Finally, logarithmic error was employed in Pagan and Schwert (1990) and Andersen et al. (1999). Root mean squared error: RMSE = [. 1 1 2 2 ˆ σ − σ ( ) ] ∑ GARCH RV . n. (4.1). Mean absolute error: MAE =. 1 ∑ σˆ GARCH − σ RV . n. (4.2). Logarithmic error: LE =. σ 1 [log( RV )]2 . ∑ n σˆ GARCH 11. (4.3).
(20) In measures of (4.1), (4.2), and (4.3), σˆ GARCH are the volatilities estimated by the GARCH(1,1) model, and σ RV are realized volatilities. With the accuracy measures of RMSE, MAE, and LE, it is easier to judge which model is more consistent with “true” volatility. The next chapter will provide results of empirical analysis for each criterion under the GARCH model.. 12.
(21) 5. Empirical Analysis Estimation of the parameters and volatilities in a GARCH(1,1) model could be based on data from the entire period. However, if we do not consider some extraordinary events occurring during the period while estimating the parameters, the estimates so obtained may not be meaningful. Hence, we want to detect some change points and find several such events according to historical incidents. Consequently, we will divide 163 days into several subperiods. We then estimate the parameters of the GARCH(1,1) model for each subperiod. At the same time, we hope that volatilities estimated with change points can have better performance than those ignoring such changes. That is, volatilities with change points will yield smaller values of accuracy measures.. 5.1 Empirical Volatility Measurement By using methods in the Western Electric Handbook (1956) (see methods 1 to 5 in section 3.3), we find some candidates of change points. Since there is no point exceeding the 3-sigma limit from Figure 5.1, we say that the whole pattern is random by method 1 of Western Electric rules. In Table 5.1, there are two candidates of change points by using method 2 but the LRT values are less than 9.488. That means the candidates of change points can not change data structure. By method 3, as indicated in Table 5.2, there is only one candidate with the LRT value less than the critical value. Absence of change points detected by method 5 (Five consecutive points are in a rising or falling trend) produces a natural pattern. It is much similar to the result of method 1 above. We can observe from Table 5.3 that when period 1 (Jan. 2, 2003 to May 14, 2003). 13.
(22) and period 2 (May 15, 2003 to Aug. 29, 2003) had been decided, the value of the likelihood ratio test is bigger than 9.488.. λ = −2 log[. L( period1 + period 2) 2 ] > χ 4,1 − 0.05 = 9.488 L( period1) L( period 2). According the LRT, the only change point occurred on May 14, 2003. The LRT statistic yields a value bigger than the critical value of 9.488. However, other candidate points are not change points according to the LRT. Our finding coincides with the news announcement on May 14, 2003 that the epidemic SARS had reached the countryside of Mainland China.4 Hence, we can separate 163 days into two subperiods. From Figure 5.2, it is clear and observable that the 87 th point is a possible change point of events. Before the 87 th day, the returns had more substantial variations than the remainder. The epidemic SARS has since subsided and consequently the stock market became more stable. After making sure of the change point, we can rewrite separate formulae for separate subperiods as follows: 1 rt = 0.014 − 1.79319 ht − ht + at , 2 ht = σ t2 = 0.0003956 + 0.0044637at2−1 + 0.726661σ t2−1 ,. (5.1). for the GARCH(1,1) model of period 1, and 1 rt = 0.014 − 1.50269 ht − ht + at , 2 ht = σ t2 = 0.00015201 + 0.0173133at2−1 + 0.13366σ t2−1 ,. (5.2). for the GARCH(1,1) model of period 2. These formulae indicate that the parameters change significantly. Meanwhile, if we ignore the change and fit the GARCH(1,1) __________________________ 4. Data source: http://sars.health.gov.tw/article.asp?channelid=H&serial=189&click=. 14.
(23) model for the entire period, we have 1 rt = 0.014 − 1.57103 ht − ht + at , 2 ht = σ t2 = 0.0006657 + 0.0043164at2−1 + 0.480879σ t2−1 ,. (5.3). which is quite different from either (5.1) or (5.2). The estimates of parameters for different models are shown in Table 5.4. For an GARCH model, the standardized shocks at =. at σˆ t. are iid and N(0,1). And the Ljung-Box (1978) Q-statistic is expressed as below ρˆl2 Q(m) = T (T + 2)∑ , l =1 T − l m. where ρˆl is the lag-l sample autocorrelation of at or at 2 .Table 5.7 shows the value of the Ljung-Box Q-statistic for the squares of the standardized shocks. It is also noted that the Q-statistic has also been computed for the standardized shocks themselves and the adequacy of the models has been established as well. We can check this table and find the fitted models are all adequate. Table 5.5 reports the in-sample forecasting accuracy criteria RMSE, MAE, and LE, respectively. It compares the performance of the fitted models. The RMSE criterion is not very robust, and in practice easily influenced by a few large values. The MAE criterion is less susceptible to these values. Under the accuracy measures for the GARCH(1,1) model, we find higher values for RMSE, MAE and LE when no change point was detected. For example, RMSE for the GARCH(1,1) model with change point is 0.0190417 versus 0.0234972 without change point. Thus, detecting structural change is meaningful in fitting the GARCH(1,1) models to financial data.. 15.
(24) 5.2 Some Issues of Interest. There are some interesting issues derived from the empirical results. We will illustrate them as follows. Besides estimating volatility, GARCH types of models have some interesting and useful properties. If we standardized the return, zt =. rt , σˆ t. (5.4). then zt will be distributed as N(0,1) when the normality assumption is reasonable for rt . We can verify this by calculating some statistics, such as mean, standard deviation, skewness and excess kurtosis, as given in Table 5.6. Skewness characterizes the degree of asymmetry of a distribution around its mean. Positive (Negative) skewness indicates a distribution with an asymmetric tail extending towards more positive (negative) values. The skewness of normal distribution is zero. A significant degree of asymmetry (skewness regardless of sign) is bigger than 2 standard errors of skewness (ses). The ses can be estimated by using the following formula (Tabachnick & Fidell, 1996): ses =. 6 , N. where N is the number of observations. The excess kurtosis of a standard normal random variable is zero. A distribution with positive excess kurtosis is said to have heavy tails. It implies that the distribution has more mass on the tails than a normal distribution. A significant degree of mass tail (kurtosis regardless of sign) is bigger than 2 standard errors of kurtosis (sek). The sek can be estimated roughly using the following formula (Tabachnick & Fidell, 1996): sek = where N is the number of observations. 16. 24 , N.
(25) From Table 5.6, it is clear that the GARCH(1,1) models have heavy tails due to positive excess kurtosis. Hence, the GARCH(1,1) models are not reasonable for modeling volatilities. Since no proper detection of change point is done, the GARCH(1,1) model without change points can be affected by the oscillation of prices coming from some unusual events. In order to get more insight, we combine Table 5.6, Figure 5.3.1 and Figure 5.3.2. Figure 5.3.1 and Figure 5.3.2 are the quantile-quantile (q-q) plots. The q-q plot is a graphical technique for determining if the data have come from a certain distribution. Realized volatility may be fitted with standard normal distribution as shown in Figure 5.3.2. However, it will provide only daily reference and will have no capability of prediction. Meanwhile, it seems that the GARCH(1,1) models are fitted somewhat poorly with the standard normal distribution as shown in Figure 5.3.1. From Table 5.6, we see that the GARCH(1,1) models with or without change points have positive skewness. That means that standardized returns under the GARCH(1,1) models are slightly skewed to the right. The values of Jaque-Bera (JB) test5 in Table 5.6 show that the GARCH(1,1) model with change points is fitted better with standard normal distribution than the GARCH(1,1) model without change points. Furthermore, the volatilities under the GARCH(1,1) models are overvalued because their variances of standardized returns are less than 1, as shown in Table 5.6. In short, the GARCH(1,1) models with change points will be a useful model for our purpose, even though it is not perfect. __________________________ 5. Jarque and Bera (1980,1987) showed the Jaque-Bera (JB) test of normality:. S2 K2 ~ χ22 JB = n + 6 24 , where S = skewness coefficient and K =excess kurtosis coefficient. For a normally distributed variable, S = 0 and K = 0. Therefore, the JB test of normality is a test of the joint hypothesis that S and K are 0 and 0, respectively. 17.
(26) 6 Conclusion Throughout the thesis, the period of the data is from Jan. 2, 2003 to Aug. 29, 2003. It is the period of initial and terminal stages when epidemic SARS spread globally. During this period, many events related to SARS deserve some discussions. In chapter 5, the LRT was applied to the TAIEX data and detected several change points. We deeply hope that ignoring the change points will lead to a wrong conclusion. As a matter of fact, it is important to detect change points. Overall, we believe our test constitutes a functional tool for testing for a parameter change in the GARCH(1,1) models. We anticipate that methods in the Western Electric Handbook that combined with the LRT can be extended to other types of time series models. Finally, empirical results are consistent with our expectation. We should attach importance to structural change in time series models which will lead to better prediction. However, overvalued-volatility is a defect of GARCH models. Perhaps, realized volatility mixed with GARCH model can be a useful model. We leave the task of extension to other types of GARCH models for future research.. 18.
(27) References Andersen, T. G., Bollerslev, T., Diebold, F. and Labys, P. (2001a), "The distribution of exchange rate volatility, " Journal of the American Statistical Association 96, 42-55.. Andersen, T. G., Bollerslev, T., Diebold, F. and Ebens, H. (2001b), "The distribution of realized stock return volatility, " Journal of Financial Economics 61, 43-76.. Andersen, T.G., Bollerslev, T. and Diebold, F. (2002), "Parametric and Nonparametric Volatility Measurement," in L.P. Hansen and Y. Ait-Sahalia (eds.), Handbook of Financial Econometrics. Amsterdam: North-Holland, forthcoming.. Andersen, T. G., Bollerslev, T. and Lange, S. (1999), “Forecasting Financial Market Volatility: Sample Frequency vis-a-vis Forecast Horizon,” Journal of Empirical Finance 6, 457-477.. “Statistical Quality Control Handbook,” Western Electric Company, Inc. Mack Printing Co. Easton. Pa., 1956.. Bollerslev, T. (1986), “Generalized autoregressive condtional heteroskedasticity,” Journal of Econometrics 31, 307–327.. Bollerslev, T. (2001), "Financial econometrics: past developments and future challenges", Journal of Econometrics 100, 41-51.. 19.
(28) Barndoff-Nielsen, O. E. and Shepard, N. (2002a), “Econometric analysis of realized volatility and its use in estimating stochastic volatility models,” Journal of the Royal Statistical Society 64, 253-280.. Barndoff-Nielsen, O. E. and Shepard, N. (2002b), “Estimating quadratic variation using realized variance,” Journal of Applied Econometrics 17, 457-477.. Barndoff-Nielsen, O. E. and Shepard, N.(2002c), “How accurate is the asymptotic approximation to the distribution of realized volatility?” in D. W. F. Andrews, J. L. Powell, P. A. Ruud and J. H. Stock (eds), Identification and Inference for Econometric Models, forthcoming.. Duan, J. C. (1995), “The GARCH option pricing model,” Journal of Mathematical Finance 6, 13-32. Fleming, J. (1998), “The quality of market volatility forecasts implied by S&P 100 index option prices,” Journal of Empirical Finance 5, 317-345.. Jarque, C. M. and Bera, A. K. (1980), “ Efficient tests for normality, homoscedasticity and serial independence of regression residuals,” Economics Letters, 6, 255-259.. Jarque, C. M. and Bera, A. K. (1987), “A test for normality of observations and regression residuals,” International Statistical Review, 55, 163-172.. Ljung, G., and Box, G. E. P. (1978), “On a measure of lack of fit in time series models,” Biometrika, 66, 67-72. 20.
(29) Pagan, A. R. and Schwert, G. W.(1990), “Alternative models for conditional stock volatility,” Journal of Econometrics 45, 267-290.. Tabachnick, B. G. and Fidell, L. S. (1996). Using multivariate statistics (3rd ed.). New York: Harper Collins.. 21.
(30) Tables Table 2.1 TAIEX option Item. Description. Underlying Index. Taiwan Stock Exchange Capitalization Weighted Stock Index (TAIEX). Ticker Symbol. TXO. Exercise Style. European. Multiplier. NT$ 50 (per index point). Expiration Months. Spot month, the next two calendar months followed by two additional months from the March quarterly Cycle (March, June, September, and December) 100 index points in spot month, the next two calendar months. Strike Price Interval. 200 index points in the additional two months from the March quarterly Cycle When listing series of new expiration months, one series with at-the-money strike price is listed based on the previous day's closing price of the underlying index rounded down to the nearest multiples of 100. 1. For the spot month and the next two calendar months: Three other series each with in-the-money and out-of-the-money strike prices with price interval of 100 points are listed.. Strike (Exercise) Price. 2. For the next two quarter-months: Two other series each with in-the-money and out-of-the-money strike prices with price interval of 200 points are listed. Up to the 5th business days before expiration, 1. For the spot month, and the next two calendar months: additional series are added when the underlying trades through the third highest or lowest strike prices available, to maintain at least 3 in- and 3 out-of-the-money strike prices 2. For the next two quarter-months: additional series are 22.
(31) added when the underlying trades through the second highest or lowest strike price available, to maintain at least 2 in-and 2 out-of-the-money strike prices < 10 points: 0.1 point (NT$5) >=10 points,<50 points: 0.5 point (NT$ 25) Premium Quotation. >=50 points, <500 points: 1 point (NT$ 50) >=500 points, <1,000 points: 5 point (NT$ 250) >=1,000 points: 10 point (NT$ 500). Daily Price Limit. +/- 7% of previous day's closing price of the underlying index Individuals: 8,000contracts on either side of the market. Institutional Investors: 16,000 contracts on either side of the market.. Position Limit. Institutional investors may apply for an exemption from the above limit on trading accounts for hedging purpose. Exemptions are allowed for Future Proprietary Firms.. Trading Hours. 08:45AM - 1:45 PM Taiwan time, Monday through Friday of the regular Taiwan Stock Exchange business days. Last Trading Day. The third Wednesday of the delivery month. Expiration Date. The first business day following the last trading day. The final settlement price for each contract is computed from the first fifteen-minute volume-weighted average of each component stock's prices in that index on the final settlement Final Settlement Price day. For those component stocks that are not traded during the beginning fifteen-minute interval on the final settlement day, their last closing prices would be applied instead. Settlement. Cash settlement. An option that is in-the-money and has not been liquidated or exercised on the expiration day shall, in the absence of contrary instructions delivered to the Exchange by the Clearing Member representing the option buyer, be exercised automatically. Data source: http://www.taifex.com.tw/. 23.
(32) Table 5.1 Candidates of change points by method 2 (Two out of three consecutive points plot between a distance of 2 sigma and 3 sigma) Candidates. Date. LRT value. Testing. Change point= 72. Apr.. 22, 2003. LRT=4.51952<9.488. Accept H0. Change point= 73. Apr.. 23, 2003. LRT=5.19142<9.488. Accept H0. Table 5.2 Candidates of change points by method 3 (Ten out of eleven consecutive points plot on one side of the centerline) Candidates Change point= 101. Date Jun.. 3,. LRT value 2003. LRT=7.5551<9.488. Testing Accept H0. Table 5.3 Candidates of change points by method 4 (A run of five consecutive points of returns plot on one side of the centerline) Candidates. Date. LRT value. Testing. Change point= 36. Mar.. 3,. 2003. LRT=3.31969<9.488. Accept H0. Change point= 37. Mar.. 4,. 2003. LRT=3.21291<9.488. Accept H0. Change point= 87. May. 14, 2003. LRT=10.75551>9.488. Reject H0. Change point=106. Jun.. 11, 2003. LRT=5.44082<9.488. Accept H0. Change point=107. Jun.. 12, 2003. LRT=5.24297<9.488. Accept H0. Change point=119. Jun.. 30, 2003. LRT=2.92873<9.488. Accept H0. Change point=120. Jul.. 1,. LRT=2.83138<9.488. Accept H0. 2003. 24.
(33) Table 5.4 Parameter estimates of GARCH (1,1) model Parameters. Alpha0. Alpha1. Beta1. 0. 00039566 0. 00446372 0. 72666151 (0.0000432084) (0.002081046) (0.0004901274) 0. 00015201 1. 01731336 2. 13366277 GARCH(1,1) model of period 2 (0.0000383383) (0.0029162786) (0.0688668976) 1. 00431641 2. 48087884 GARCH(1,1) model of period 1+2 0. 00066570 (0.0016216986) (0.0208480670) (1.1261979466) GARCH(1,1) model of period 1. The numbers in parentheses are the standard errors of the parameters.. Table 5.5 The calculations of accuracy measures in every model Volatility of GARCH(1,1) model Volatility of GARCH(1,1) model with change point. without change point. RMSE. 0.01904166569. 0.02349722250. MAE. 0.01433519575. 0.02296283234. LE. 0.81310630801. 1.254419791781. Table 5.6 Some statistics of standardized returns GARCH(1,1) with. GARCH(1,1) RV. c. p.*. without c. p.. Mean. 0.130611159. 0.05201497. 0.041427136. Standard deviation. 0.745337197. 0.450746075. 1.180303863. Skewness. 0.662977222. 0.454234493. -0.318000015. Excess kurtosis. 1.399504106. 2.020160196. 0.542824948. JB test. 25.24304206. 33.32238285. 4.748427444. * c.p. means change point. 25.
(34) Table 5.7 The Ljung-Box Q-Statistic Ljung-Box Q-Statistic. Q(10). 5.5473 (0.85175957) 11.7482 GARCH(1,1) model of period 2 (0.30225856) 6.8301 GARCH(1,1) model of period 1+2 (0.74137724) GARCH(1,1) model of period 1. Q(20) 13.6110 (0.84966122) 19.5059 (0.48919712) 11.6695 (0.92698101). The numbers in parentheses are p value of the test statistic. Thus, these models appear to be adequate.. 26.
(35) Figures. Figure 2.1 The SARS daily probable cases happened in Taiwan from Feb. 25, 2003 to Sep. 4, 2003.. Data source: http://sars.health.gov.tw/. 27.
(36) Figure 2.2 Stock prices. Data source:Taiwan Stock Exchange Capitalization Weighted Stock Index from 2003.1.2 to 2003.8.29, 162 days in the aggregate. It comes from Taiwan Stock Exchange Corporation. Website: http://www.tse.com.tw/. 28.
(37) Figure 3.1 Realized volatility of TAIEX during Jan.2, 2003 to Aug. 29, 2003 with 5-min intraday returns.. 29.
(38) Figure 5.1 Log returns under 6 sigma criterion. 30.
(39) Figure 5.2 Log returns from Jan. 2, 2003 to Aug. 29, 2003. 31.
(40) Figure 5.3.1 QQ-plots of the GARCH(1,1) models versus standard normal distribution (A). (B). 32.
(41) Figure 5.3.2 QQ-plot of realized volatility versus standard normal distribution. 33.
(42)
數據

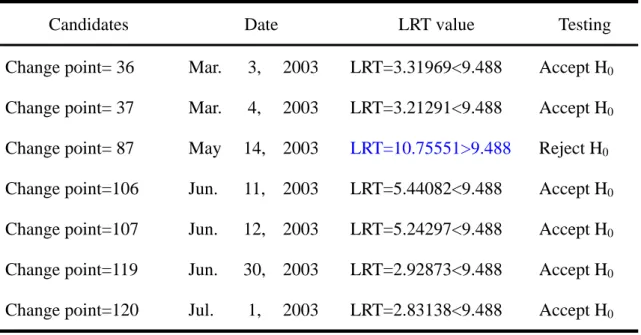

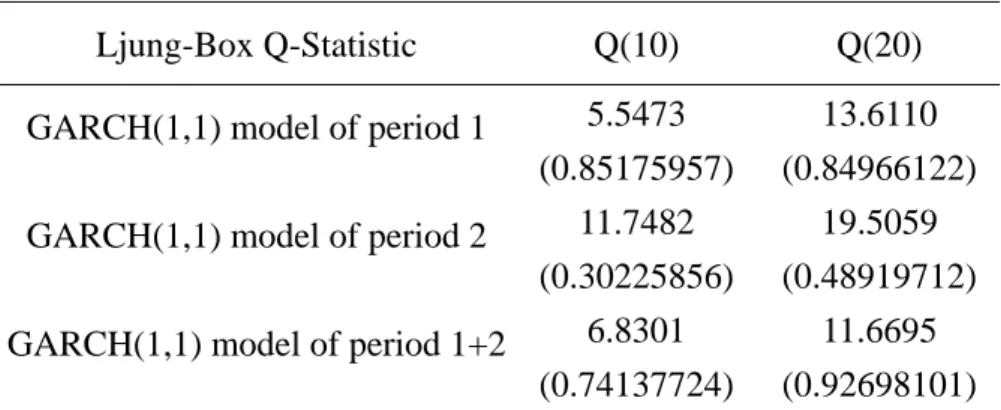
相關文件
For problems 1 to 9 find the general solution and/or the particular solution that satisfy the given initial conditions:. For problems 11 to 14 find the order of the ODE and
For the data sets used in this thesis we find that F-score performs well when the number of features is large, and for small data the two methods using the gradient of the
It is based on the goals of senior secondary education and on other official documents related to the curriculum and assessment reform since 2000, including
This thesis will focus on the research for the affection of trading trend to internationalization, globlization and the Acting role and influence on high tech field, the change
Abstract - The main purpose of this study is applying TRIZ theory to construct the Green Supply Chain management (GSCM) strategies for the international tourist hotel.. Based on
The main purpose of this study is applying TRIZ theory to construct the Green Supply Chain management (GSCM) strategies for the international tourist hotel1. Based on the
There are two main topics in this thesis: personalized mechanisms for exhibitions and interfaces equipped with cyber-physical concept and the services supporting for this
The purpose of this paper is to use data mining method in semiconductor production to explore the relation of engineering data and wafer accept test.. In this paper, we use two