Mining Frequent Itemsets from Data Streams with a Time-Sensitive Sliding Window
全文
數據

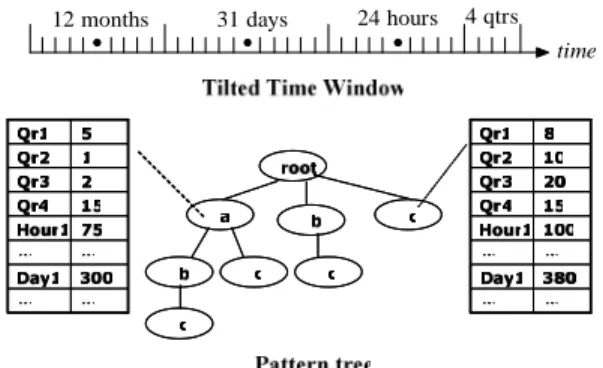


相關文件
Reading Task 6: Genre Structure and Language Features. • Now let’s look at how language features (e.g. sentence patterns) are connected to the structure
This paper presents (i) a review of item selection algorithms from Robbins–Monro to Fred Lord; (ii) the establishment of a large sample foundation for Fred Lord’s maximum
Miroslav Fiedler, Praha, Algebraic connectivity of graphs, Czechoslovak Mathematical Journal 23 (98) 1973,
In the past researches, all kinds of the clustering algorithms are proposed for dealing with high dimensional data in large data sets.. Nevertheless, almost all of
Following the supply by the school of a copy of personal data in compliance with a data access request, the requestor is entitled to ask for correction of the personal data
In the work of Qian and Sejnowski a window of 13 secondary structure predictions is used as input to a fully connected structure-structure network with 40 hidden units.. Thus,
The remaining positions contain //the rest of the original array elements //the rest of the original array elements.
Experiment a little with the Hello program. It will say that it has no clue what you mean by ouch. The exact wording of the error message is dependent on the compiler, but it might