資訊檢索之學術智慧 - 政大學術集成
全文
(2) 謝. 辭. 『他對我說:我的恩典夠你用的,因為我的能力是在人的軟弱上顯得完全。』[歌 林多後書 12:9] 五年的時間終於可以完成我的學業與夢想,真的很辛苦但也很紮實,首先要 感謝我的兩位指導老師諶家蘭老師以及林我聰老師。諶老師不僅在研究上非常認 真的指導我,幫我訂立目標建構夢想也幫助我逐夢踏實,不僅在做學問上教導我 嚴謹的態度在生命的陪伴上更是給我許多慈愛的引導,我永遠不會忘記老師教導 我生命是一種恩典,我們有可能沒有明天,所以我們要珍惜每一天的生命,努力 的去活並且珍惜時間的去熱愛生命。林老師則是在邏輯的辨證中常常給我很多的 啟發,特別是林老師總是給我正面的能量,讓我常常可以提起精神再出發。兩位 老師在博士生的生涯中都給我很大的幫助。. 政 治 大. 論文口試期間,承蒙口試委員李瑞庭老師、翁頌舜老師以及劉敦仁老師的悉 心指正及建議,使得論文更臻完善與嚴謹。此外,協助本論文訪談部分的陳良弼 老師、沈錳坤老師、楊婉秀老師以及周棟祥學長和王貞淑學姊,在此謹致上最誠 摯的謝意。. 立. ‧ 國. 學. ‧. 求學期間,非常感謝筱芳和明汶兩位求學的好伙伴,我們一起組讀書會,一 起學習,彼此扶持照顧讓我在博士班的學習上不覺孤單。此外還有許多的學長姊 和學弟妹非常感謝各位使得我的博士生生活很精采。. Nat. y. sit. n. al. er. io. 當然我要特別提到木柵靈糧堂的眾弟兄姐妹使我擁有一個龐大的家族彼此 的遮蓋幫補,特別提到我的屬靈父母,主任牧師志強哥和師母佩盈姐在初信的時 候給我許多的造就,區牧靜儀姐和銀珍姊對我的關懷,豐玲姐更是我的伯樂,特 別是在最後面試教職的時候全心全意的給我訓練與幫助,讓我可以應屆取得教 職,我很榮幸成為她的千里馬,更有屬靈同伴文潔、馨誼、昭德、羽仟、慶章、 偉華、念郇、凱忻、思韻、方傑、元懷的陪伴與扶持,當然還有愛我的家人。. Ch. engchi. i Un. v. 但我最想要感謝上帝,因為在我最軟弱無助的時候祂把我找回來,並且肯定 我的價值,在博士生涯中的每個環境造成的困難,每個看似不可能突破的困境, 祂都幫助我教導我引領我,不要看自己過於所當看的,要照著神所分給各人信心 的大小,看得合乎中道[羅馬書 12:3]。在苦難中堅固我的信心,改變我的生命, 我感謝上帝讓我在博士班生涯這個外人看來好像很榮耀的頭銜中了解自己的有 限和軟弱,醫治恢復我並且給我恩典要我知道祂的能力何等完全,這個學位的完 成以及教職順利的錄取都彰顯祂的榮耀,願我一生都在走祂的計畫當中,可以成 為祂聖潔合用的器皿並且榮耀祂的名。 杜逸寧 中華民國九十九年六月. 謹致. 于政大.
(3) Contents Table of Contents ...........................................................................................................ii List of Tables .................................................................................................................. v List of Figures ..............................................................................................................vii Abstract ...................................................................................................................... viii 中文摘要........................................................................................................................ x. 立. 政 治 大. ‧. ‧ 國. 學. n. er. io. sit. y. Nat. al. Ch. engchi. i. i Un. v.
(4) Table of Contents Chapter 1 Introduction ................................................................................................... 1 1.1 Research Background ...................................................................................... 3 1.2 Research Issue .................................................................................................. 5 1.2.1 Research Intelligence between Conferences and Journals.................... 5 1.2.2 Detecting Candidate Emerging Research Topics via the Bayesian Estimation of Author-Publication Correlations............................................ 10 1.2.3 Developing the Emerging Topic Detection Indices ............................ 11 1.3 Thesis Organization ....................................................................................... 12 Chapter 2 Literature Review ........................................................................................ 13 2.1 Topic Detection and Tracking ........................................................................ 13 2.2 Emerging Topic Detection ............................................................................. 15 2.3 Aging Theory ................................................................................................. 16 2.4 Information Retrieval Approach .................................................................... 17 2.5 Summary ........................................................................................................ 20 Chapter 3 The Leading Relationship between Conferences and Journals ................... 22 3.1 Experimental Design ...................................................................................... 22 3.2 Data Selection ................................................................................................ 25 3.2.1 Select the Domain ............................................................................... 25 3.2.2 Use the Keywords to Represent the Domain ...................................... 25 3.2.3 Choose Databases and Search Engines ............................................... 26 3.2.4 Pick the Descriptor of the Paper ......................................................... 28 3.3 Datasets Properties ......................................................................................... 29 3.3.1 Search Conference Papers................................................................... 29 3.3.2 Search Journal Papers ......................................................................... 31 3.4 Application of Information Retrieval............................................................. 32 3.4.1 Identify Each Document ..................................................................... 32 3.4.2 Calculate Frequency of Appearances .................................................. 33 3.4.3 Summarize the Frequency of Conference Papers and Journal Papers 33 3.4.4 Compute Similarity between Conference Papers and Journal Papers 34 3.5 Relationship between Conference Papers and Journal Papers ....................... 35 Chapter 4 The Experimental Results of the Relationship between Conferences and Journals ........................................................................................................................ 37 4.1 Experimental Results ..................................................................................... 37 4.2 Discussion ...................................................................................................... 40 4.3 Summery ........................................................................................................ 43 Chapter 5 The Detection of Impact Research Topics via Bayesian Estimation of Author-Publication Correlations .................................................................................. 45. 立. 政 治 大. ‧. ‧ 國. 學. n. er. io. sit. y. Nat. al. Ch. engchi. ii. i Un. v.
(5) 5.1 The Idea of Detecting Impact Research Topics ............................................. 46 5.2 Measuring an Author’s Impact Power ........................................................... 48 5.2.1 Prior Impact Power of an Author ........................................................ 48 5.2.2 Likelihood Function of the Impact Power of an Author ..................... 49 5.2.3 Posterior Impact Power of an Author.................................................. 50 5.3 Measuring the Impact Power of a Publication ............................................... 51 5.3.1 Prior Impact Power of a Publication ................................................... 52 5.3.2 Likelihood Function of the Impact Power of a Publication ................ 52 5.3.3 Posterior Impact Power of a Publication ............................................ 53 5.4 Measuring the Impact Power of a Paper and a Topic .................................... 54 5.4.1 Impact Power of a Paper ..................................................................... 55 5.4.2 Impact Power of a Topic ..................................................................... 57 Chapter 6 Determination of Impact Research Topics via the Bayesian Estimation of Author-Publication Correlations .................................................................................. 59 6.1 Experiment to Validate an Author’s Impact Power ........................................ 59 6.1.1 Comparing the author’s impact power with previous work................ 59 6.1.2 Comparing the impact power of authors with the expert survey ........ 63 6.2 Experiment to Validate the Impact Power of Publications ............................ 65 6.2.1 Comparing the impact power of publications using the impact factor66 6.2.2 Comparing the impact power of publications using the publication list of authors recommended in previous work .................................................. 70 6.2.3 Comparing the impact power of publications with the experts survey ...................................................................................................................... 73 6.3 How to find impact research topics using the proposed model ..................... 77 Chapter 7 The Indices for Emerging Topic Detection ................................................. 82 7.1 Novelty of Emerging Topics .......................................................................... 82 7.1.1 Term, Candidate Research Topic, Research Topic, Hot Topic and Emerging Topic ............................................................................................ 82 7.1.2 Novelty Index...................................................................................... 83 7.1.3 Published Volume Index ..................................................................... 85 7.1.4 Detection Point.................................................................................... 87 7.2 Information Produced by the Emerging Topic Detection Indices .................. 89 7.2.1 Year of the Detection Point ................................................................. 89 7.2.2 The Detection Point Value .................................................................. 89 7.3 The Properties of Emerging Topic Detection Indices .................................... 90 7.3.1 Novelty Index Properties .................................................................... 90 7.3.2 Published Volume Index Properties .................................................... 91 7.3.3 Detection Point Properties .................................................................. 92. 立. 政 治 大. ‧. ‧ 國. 學. n. er. io. sit. y. Nat. al. Ch. engchi. iii. i Un. v.
(6) 7.4 The Emerging Topic Detection Table ............................................................ 93 Chapter 8 The Research Experiment of the Development of Emerging Topic Detection Indices ......................................................................................................... 95 8.1 Experimental Design ...................................................................................... 95 8.1.1 Choose the Field and Data Resource .................................................. 95 8.1.2 Select the Descriptor ........................................................................... 96 8.1.3 Investigate the Extracted Topics ......................................................... 96 8.2 Experimental Results ..................................................................................... 97 8.3 How to Use the Emerging Topic Detection Table to Predict Whether a Topic Warrant Further Research .................................................................................... 99 8.4 Validate the Accuracy and Effectiveness of the Emerging Topic Detection Indices ................................................................................................................ 101 8.5 Discussion .................................................................................................... 107 8.6 Summary ...................................................................................................... 109 Chapter 9 Conclusions and Future Work ................................................................... 111 9.1 Conclusions .................................................................................................. 111 9.2 Future Work ................................................................................................. 113 References .................................................................................................................. 115 Appendix A Questionnaire .................................................................................... 121. 立. 政 治 大. ‧. ‧ 國. 學. n. er. io. sit. y. Nat. al. Ch. engchi. iv. i Un. v.
(7) List of Tables Table 1-1 The Four Categories of Correlations Between Conference Papers and Journal Papers. ...................................................................................................... 8 Table 3-1 Names of Conferences Responsible for the Publishing of All Conference Papers Collected. ................................................................................................... 30 Table 3-2 Number of Conferences Recorded in the Databases for Conference Papers and the Number of Papers Cited. .......................................................................... 30 Table 3-3 The Data of Journals Collected in Databases and the Number of Papers Cited. ..................................................................................................................... 31 Table 3-4 How Papers Are Recorded Under Features. ............................................... 33 Table 3-5 Features for Each Year (Part of the Experiment). ....................................... 34 Table 4-1 Similarity Between C1991-C2007 and J1990-J2007. ................................ 38 Table 4-2 Similarity in Boolean Expression Between C1991-C2007 and J1990-J2007. ............................................................................................................................... 38 Table 4-3 Similarity Between C1991-C2006 and J1991-J2007. ................................ 39 Table 4-4 Similarity in Boolean Expression Between C1991-C2006 and J1991-J2007. ............................................................................................................................... 39 Table 4-5 Similarity in Boolean Expression Between C1990-C2006 and J1991-J2007. ............................................................................................................................... 40 Table 4-6 The Features of the C1995, C1996, J1997 and J1998. ............................... 41 Table 5-1 Example of Calculating Each Author’s Prior IPA for Topic A. .................. 49 Table 5-2 Example of How to Compute Each Author’s LF (IPA) in Topic A. ........... 49 Table 5-3 Example of How to Compute Each Author’s Posterior (IPA) in Topic A. . 51 Table 5-4 Example of How to Calculate Each Publication’s Prior (IPP) in Topic A. . 52 Table 5-5 Example of How to Compute Each Author’s LF(IPP) for Topic A. ........... 53 Table 5-6 Example of How to Compute Each Publication’s Posterior (IPP) for Topic A. ........................................................................................................................... 54 Table 5-7 Information about Papers Involved in Topic A. .......................................... 55 Table 5-8 Impact Power of Each Paper in Topic A. .................................................... 56 Table 6-1 Top 10 Impact Authors in the Topic of Data Mining. ................................. 62 Table 6-2 Top 10 Impact Authors within the Topic of Data Mining (during 1990-2002). ........................................................................................................... 63 Table 6-3 Grades of Authors Surveyed by Academic Experts. ................................... 64 Table 6-4 Precision and Recall of Author Impact Obtained with Each Model. .......... 64 Table 6-5 Statistics for Papers and Publications. ........................................................ 67 Table 6-6 Top 35 Impact Publications in Topic of Data Mining (during 1990-2002).67 Table 6-7 Top 10 Impact Journals in Topic of Data Mining (during 1990-2002). ...... 69 Table 6-8 Top 10 Impact Conferences in Topic of Data Mining (during 1990-2002) 69. 立. 政 治 大. ‧. ‧ 國. 學. n. er. io. sit. y. Nat. al. Ch. engchi. v. i Un. v.
(8) Table 6-9 Top 10 Impact Publications in Topic of Data Mining (during 1990-2002).70 Table 6-10 Comparison of the Impact Power of Publications to the Lists of Authors Suggested by Three Different Models................................................................... 71 Table 6-11 Information, Recall Rate, and Precision Rate of Each Model. ................. 72 Table 6-12 Precision of Each Model While Considering the Lists of Suggested Publications. .......................................................................................................... 73 Table 6-13 Comparison of Journals Ranks Between the Experts and Our Model...... 74 Table 6-14 Comparison of Conference Ratings Between the Experts and Our Model. ............................................................................................................................... 75 Table 6-15 Comparison of Conferences (Higher Than the Average Score)................ 76 Table 6-16 Impact Power of the Top 10 Papers. ......................................................... 79 Table 6-17 Information on the Papers with the Top 10 Impact Power Rankings. ...... 79 Table 7-1 The Volume of Published Papers on XML in Each Year: An Example. ..... 83 Table 7-2 The NI of XML Example in Each Year....................................................... 85 Table 7-3 The PVI of XML Example in Journals for Each Year ................................ 87 Table 7-4 The Values of Indices for XML Example in Emerging topic Detection. .... 88 Table 7-5 The PVI Table of Different Situations in XML Example. .......................... 91 Table 8-1 The Emerging Topic Detection Experiment. .............................................. 99 Table 8-2 How to Use the Detection Table: An Example of Virtual Environment. .. 100. 立. 政 治 大. ‧. ‧ 國. 學. n. er. io. sit. y. Nat. al. Ch. engchi. vi. i Un. v.
(9) List of Figures Fig. 3-1 The Research Experiment Process. ............................................................... 24 Fig. 3-2 The Relationship Between Domain, Sub-Domains and Keywords. ............. 26 Fig. 3-3 The Process of Feature Selection. ................................................................. 32 Fig. 6-3 Process of Detecting Candidate Emerging Topics. ....................................... 78 Fig. 6-4 Process of Validating the Candidate Emerging Topics. ................................. 81 Fig. 7-1 The Detection Point of Emerging Topic Detection Index of XML. .............. 89 Fig. 7-2 The PVI Curves of Different Situations in XML Example........................... 92 Fig. 8-1 The Topic of Image Retrieval Evolution Over Time from 1994 to 2004 (Jo et al., 2007). ............................................................................................................ 102 Fig. 8-2 The Topic of Image Retrieval Evolution Over Time from 1997 to 2008 in This Research. ..................................................................................................... 102 Fig. 8-3 The Detection Point of Image Retrieval from 1997 to 2004 ....................... 103 Fig. 8-4 The Topic Evolution of Sensor Networks Over Time from 1994 to 2004 (Jo et al., 2007).......................................................................................................... 103 Fig. 8-5 The Conference Papers Published Volume of Sensor Networks in ACM Database. ............................................................................................................. 104 Fig. 8-6 The Journal Papers Published Volume of Sensor Networks in ACM Database. ............................................................................................................................. 104 Fig. 8-7 The Detection Point of Sensor Networks in ACM Database. ..................... 104 Fig. 8-8 The Topic Evolution of Semantic Web Over Time from 1994 to 2004 (Jo et al., 2007). ............................................................................................................ 105 Fig. 8-9 The Conference Papers Published Volume of Sensor Networks in ACM Database. ............................................................................................................. 105 Fig. 8-10 The Journal Papers Published Volume of Sensor Networks in ACM Database. ............................................................................................................. 106 Fig. 8-11 The Detection Point of Semantic Web in ACM Database. ........................ 106 Fig. 8-12 The Topic Evolution of Support Vector Over Time from 1994 to 2004 (Jo et al., 2007). ............................................................................................................ 107 Fig. 8-13 The Detection Point of Support Vector in ACM Database ........................ 107. 立. 政 治 大. ‧. ‧ 國. 學. n. er. io. sit. y. Nat. al. Ch. engchi. vii. i Un. v.
(10) Abstract This research presents endeavors that seek to identify the emerging topics for researchers and pinpoint research intelligence via academic papers. It is intended to reveal the connection between topics investigated by conference papers and journal papers which can help the research decrease the plenty of time and effort to detect all the academic papers. In order to detect the emerging research topics the study uses the Bayesian estimation approach to estimate the impact of the authors and publications may have on a topic and to discover candidate emerging topics by the combination of the impact authors and publications. Finally the research also develops the measurement tools which could assess the research potential of these topics to find the emerging topics. This research selected huge of papers in data mining and information retrieval from well-known databases and showed that the topics covered by conference papers in a year often leads to similar topics covered by journal papers in the subsequent year and vice versa. This study also uses some existing algorithms and combination of these algorithms to propose a new detective procedure for the researchers to detect the new trend and get the academic intelligence from conferences and journals. The research uses the Bayesian estimation approach and citation analysis methods to construct the prior distribution and likelihood function of the authors and publications in a topic. Because the topics published by these authors and publications will get more attention and valuable than others. Researchers can assess the potential of these candidate emerging topics. Although the topics we recommend decrease the range of the searching space, these topics may so popular that even all of the impact authors and publications discuss it. The measurement tools or indices are need. But the current methods only focus on the frequency of subjects, and ignore the novelty of subjects which is critical and beyond the frequency study or only focus one of them and without considering the potential of the topics. Some of them only use the curve of published frequency will make the index as a backward one. This research tackles the inadequacy to propose a set of new indices of novelty for emerging topic detection. They are the novelty index (NI) and the published volume index (PVI). These indices are then utilized to determine the detection point (DP) of emerging topics. The detection point (DP) is not the real time which the topic starts to be emerging, but it represents the topic have the highest potential no matter in novelty or hotness for research in its life cycle. Different from the absolute frequent method which can really find the exact emerging period of the topic, the PVI uses the accumulative relative frequency and tries to detect the research potential timing of its life cycle. Following the detection points, the intersection decides the worthiness of a new topic. Readers. 立. 政 治 大. ‧. ‧ 國. 學. n. er. io. sit. y. Nat. al. Ch. engchi. viii. i Un. v.
(11) following the algorithms presented this thesis will be able to decide the novelty and life span of an emerging topic in their field. The novel methods we proposed can improve the limitations of impact factor proposed by ISI. Besides, it uses the impact power of the authors and the publication in a topic to measure the impact power of a paper before it really has been an impact paper can solve the limitations of Google scholar’s approach. We suggest that the topic oriented thinking of our methods can really help the researchers to solve their problems of searching the valuable topics. Keywords: Topic discovery and tracking, data mining, information retrieval, Bayesian estimation, academic intelligence, novelty index, published volume index, citation analysis.. 立. 政 治 大. ‧. ‧ 國. 學. n. er. io. sit. y. Nat. al. Ch. engchi. ix. i Un. v.
(12) 中文摘要 偵測新興議題對於研究者而言是一個相當重要的問題,研究者如何在有限 的時間和資源下探討同一領域內的新興議題將比解決已經成熟的議題帶來較大 的貢獻和影響力。本研究將致力於協助研究者偵測新興且具有未來潛力的研究議 題,並且從學術論文中探究對於研究者在做研究中有幫助的學術智慧。在搜尋可 能具有研究潛力的議題時,我們假設具有研究潛力的議題將會由同一領域中較具 有影響力的作者和刊物發表出,因此本研究使用貝式估計的方法去推估同一領域 中相關的研究者和學術刊物對於該領域的影響力,進而藉由這些資訊可以找出未 來具有潛力的新興候選議題。此外就我們所知的議題偵測文獻中對於認定一個議 題是否已經趨於成熟或者是否新穎且具有研究的潛力仍然缺乏有效及普遍使用 的衡量工具,因此本研究試圖去發展有效的衡量工具以評估議題就本身的發展生 命週期是否仍然具有繼續投入的學術價值。. 政 治 大. 本研究從許多重要的資料庫中挑選了和資料探勘和資訊檢索相關的論文並 且驗證這些在會議論文中所涵蓋的議題將會領導後續幾年期刊論文相似的議 題。此外本研究也使用了一些已經存在的演算法並且結合這些演算法發展一個檢 測的流程幫助研究者去偵測學術論文中的領導趨勢並發掘學術智慧。本研究使用 貝式估計的方法試圖從已經發表的資訊和被引用的資訊來建構估計作者和刊物 的影響力的事前機率與概似函數,並且計算出同一領域重要的作者和刊物的影響 力,當這些作者和刊物的論文發表時將會相對的具有被觀察的價值,進而檢定這 些新興候選議題是否會成為新興議題。而找出的重要研究議題雖然已經縮小探索 的範圍,但是仍然有可能是發展成熟的議題使得具有影響力的作者和刊物都必須 討論,因此需要評估議題未來潛力的指標或工具。然而目前文獻中對於評估議題 成熟的方法僅著重在議題的出現頻率而忽視了議題的新穎度也是重要的指標,另 一方面也有只為了找出新議題並沒有顧及這個議題是否具有未來的潛力。更重要 的是單一的使用出現頻率的曲線只能在議題已經成熟之後才能確定這是一個重 要的議題,使得這種方法成為落後的指標。. 立. ‧. ‧ 國. 學. n. er. io. sit. y. Nat. al. Ch. engchi. i Un. v. 本研究試圖提出解決這些困境的指標進而發展成衡量新興議題潛力的方 法。這些指標包含了新穎度指標、發表量指標和偵測點指標,藉由這些指標和曲 線可以在新興議題的偵測中提供更多前導性的資訊幫助研究者去建構各自領域 中新興議題的偵測標準。偵測點所代表的意義並非這個議題開始新興的正確日 期,它代表了這個議題在自己發展的生命週期上最具有研究的潛力和價值的時間 點,因此偵測點會根據後來的蓬勃發展而在時間上產生遞延的結果,這表示我們 的指標可以偵測出議題生命力的延續。相對於傳統的次數分配曲線可以看出議題 的崛起和衰退,本研究的發表量指標更能以生命週期的概念去看出議題在各個時 間點的發展潛力。本研究希望從這些過程中所發現的學術智慧可以幫助研究者建 構各自領域的議題偵測標準,節省大量人力與時間於探究新興議題。本研究所提. x.
(13) 出的新方法不僅可以解決影響因子這個指標的缺點,此外還可以使用作者和刊物 的影響力去針對一個尚未累積任何索引次數的論文進行潛力偵測,解決 Google 學術搜尋目前總是在論文已經被很多檢索之後才能確定論文重要性的缺點,學者 總是希望能夠領先發現重要的議題或論文。然而,我們以議題為導向的檢索方法 相信可以更確實的滿足研究者在搜尋議題或論文上的需求。 關鍵字:議題的發現與追蹤、資料探勘、資訊檢索、學術智慧、貝氏估計、新穎 度指標、發表量指標、引文分析。. 立. 政 治 大. ‧. ‧ 國. 學. n. er. io. sit. y. Nat. al. Ch. engchi. xi. i Un. v.
(14) Chapter 1 Introduction The growing pervasiveness of information technologies and Internet indicates that research is increasingly focusing on discovering academic connections between investigations. Thelwall (2005) concluded that scientific web intelligence encompasses all technologies that obtain academic intelligence from the webs. In other words, the relationships among the papers can be discovered from the hyperlinks in the webs. This conclusion naturally follows from the basic principle of citation analysis that an article cited many times is more likely to have scientific value than an uncited article. Therefore, hyperlinks and citations form endorsements that increase the value of web pages and papers more valuable. These instruments will help researchers extract research intelligence behind the papers. The research tries to find out the research intelligence involving information retrieval, especially the emerging topic of detection problem which is an important issue of information retrieval. Unlike the past works, the study also develops measurement tools which are indices that would determine whether the proposed topic is emerging or not. The study also investigates the relationships between conference papers and journal papers to find out the leading trend of the academic papers. If we are able to discern the leading trend behind academic papers, such a discovery will relieve researchers of significant effort and time in detecting emerging topics in the huge database. And then the publications and authors that possess the potential of the leading tread will be detected to find out the proposed topics. The proposed topics will be measured by the emerging topic indices to examine if the topic has excellent prospects or has already taken place. Finally, the study will help the researchers to decrease the survey domain but can find the potential emerging topic in their domain and save the time and resources by the academic intelligence we propose.. 立. 政 治 大. ‧. ‧ 國. 學. n. er. io. sit. y. Nat. al. Ch. engchi. i Un. v. The research includes three major parts of the research intelligence involving information retrieval. The first part is to investigate the relationships between academic papers which are the journal papers and conference papers. The connection between the academic productions inspires this research to explore the connections between papers. The methodologies of information retrieval can be exploited to discover the relationships between the published papers throughout the topics of papers in the database. The connections between conference papers and journal papers can help researchers to discover the academic intelligence, and help them to the deeper research works. The second part of the research is to detect the impact research topics of a 1.
(15) discipline based on the impact authors and publications may have in the same field. This discipline is called detecting emergent research topics via the Bayesian estimation of author-publication correlations. This part takes the academic position of the same field authors and publications into account. The topic with the powerful author and popular publications in the field will generate more attention than the other topics, that is to say, the proposed topic may become the hot and important one. The study will examine the proposed topic using the emerging topic detection indices which will introduce in the third part to detect its potential to be prominent. Finally the research will validate the precision and recall rate of proposed topics between conference papers and journal papers. The third part is to track the evolution of a discipline which is called the developent of the emerging topic detection index. Tracking the evolution of a discipline is important to researchers and scholars (Lee et al., 1997). Many researchers accumulate their knowledge by experience or use state-of-the-art techniques to investigate trends and identify new research topics. However, before a new research topic was identified, years passed before researchers identified the topic. Relatively, it is always that when a topic is in great demand; in other words, over hot and it started to attract the attention of many researchers. It is always a backward index. As the number of papers discussing the same topic increases, their influence decreases. Consequently, this part of study develops a novel topic-detection index that uses automatic approaches to help researchers detect topics and make proper decisions before a topic becomes popular.. 立. 政 治 大. ‧. ‧ 國. 學. er. io. sit. y. Nat. al. v. n. This study explores the connections between the academic publications. The methodologies of information retrieval and data mining can be exploited to discover the relationships between published papers among all topics. By discovering the connections between conference papers and journal papers, researchers can improve the effectiveness of their research by identifying academic intelligence.. Ch. engchi. i Un. This study discusses how conference papers and journal papers are related. The topics of conference papers are identified to determine whether they represent new trend discussed in journal papers. An automatic examination procedure based on information retrieval and data mining is also proposed to minimize the time and human resources required to predict further research developments. This study develops a new procedure and collects a dataset to verify those problems. Analytical results demonstrate that the conference papers submitted to journal papers are similar each year. Conference papers certainly affect the journal papers published over three years. About 87.23% of data points from papers published in 1991–2007 support our assumption. The research is intended to help researchers identify new trend in their 2.
(16) research fields, and focus on the urgent topics. This is particularly valuable for new researchers in their field, or those who wish to perform cross-domain studies. The research will help researchers to build their own domain emerging topic detecting indices, construct the procedure to examine leading trend in their field and find out the authors and publications with the impact to discover emerging topics in reduced but nonetheless sufficient databases. The goal of this research is fourfold: First, the research investigates if the conference papers’ themes lead the journal papers’ and examines how the new research themes can be identified from the conference papers. Second, the work uses the Bayesian estimation to connect the powerful authors and publications in some field and find out the impact research topics which we also call the candidate emerging topics. Third, the research tries to propose the emerging topic indices for researchers to assess the potential of the research topics. Finally, the study combines the results of the leading trend of academic papers, the candidate emerging topics by impact authors and publications, and the emerging topics indices to help researchers identify the research intelligence in topic detection problems.. 立. 政 治 大. ‧ 國. 學. 1.1 Research Background. ‧. n. al. er. io. sit. y. Nat. Citation analysis was initially proposed by sociologist Robert Merton, and stated that citations form cognitive links between cited and citing papers, (Thelwall, 2005). In other words, authors judge two papers to have important connections with each other. The principle of citation analysis has since been extended to many applications. Tho et al. (2007) combined ontology, data mining and information retrieval to search for professional techniques in the same filed from citations. Felix et al. (2005) adopted information visualization approaches to discover hidden connection between papers in different fields based on citation analysis, thus improving the depth of research.. Ch. engchi. i Un. v. Conversely, the citation analysis could extend to web applications with hyperlinks. Researchers can analyze hyperlinks to discover modern web intelligence. Thelwall (2004) discussed whether that universities who submit more papers to publications also publish more papers on the web. Such information is useful for determining whether citation analysis and hyperlink analysis are valuable in searching out academic intelligence in references to the papers or links between web pages, respectively. This academic intelligence establishes the criteria for measuring academic value. Additionally, these criteria also push the government, universities and researchers to measure the academic value of their work. However, whether papers create academic intelligence through their relationships 3.
(17) is an interesting issue. This study explores the relationships between conference papers and journal papers. The value of topics of conference papers in predicting those of journal papers is investigated. An automatic detective procedure based on information retrieval is also proposed. Such research requires long academic experience, and taking time to study papers, in order to gain sufficient enough ability to predict the publishing trends in the same field. Unfortunately, insufficient time and people are available to perform this task. The procedure proposed by the research could help researchers avoid the complicated TDT (Topic Detection and Tracking) approaches, and to obtain the keywords of the trend of journal papers. These make the researches’ discussion fit in with academic trends. TDT is an important filed which discusses the tracking evolution of a topic. It originated in 1996 when the DARPA (Defense Advanced Research Projects Agency) A pilot study in 1997 (Allan et al., 1998) laid down the essential ground work, generating a small corpus and establishing a durable system. TDT research flourished during 1998 and 1999 (Lee, Lee & Jang, 2007). But it is still stayed in the target of the news story.. 立. 政 治 大. ‧ 國. 學. ‧. Lee et al., (1997) started to discuss the evolution of the topics which discipline is management of information systems (MIS). They found journals and magazines focusing on different themes with the former focusing on conceptual and abstract models while the latter devoting attention to specific applications. Significantly, academic themes show more variance over time. Their work started to discuss about the trend of MIS topic but lacked the TDT techniques to help they deal with the time-consuming work.. er. io. sit. y. Nat. al. n. iv n C h etonaddress Swan and Allen (2000) started g c h itheUissue of how to automatically. overview timelines of a set of news stories. They used the X 2 -method to identify at each time a burst of feature terms that more frequently appear than at other times. Kleinberg (2002) proposed a method for analyzing document streams. Morinaga & Yamanishi (2004) improved the Kleinberg’s approach.. There are more relative works produced. We could roughly separate those methods into three groups: (1) text mining and data mining approaches such as (Hatzivassiloglou et al., 2000), (Franz & McCarley, 2001), (Kollios et al.,2003), (Clifton, et al., 2004), (Kuramochi & Karypis 2004), (Ozmutlu 2006), (Aurora et al., 2007) and (Chou & Chen, 2008), (2) Time-line burst detection of feature terms and the measurement such as (Manmatha et al., 2002), (Yang et at., 2005), (Wang et al., 2007), (Chen et al., 2007) and (3) Combined content analysis or link analysis such as (Stokes & Carthy, 2001), (Yang et al., 2002), (Wu, et al., 2004), (Ozmutlu & Cavdur 4.
(18) 2005), (Jin et al., 2007), (Steyvers et al., 2007), (Jo et al., 2007), (Nallapati et al., 2008), (Ontrup et al.,2008) and (Zhang et al., 2008). The developed emerging topic detection indices work is classified into the category of the time-line burst detection of feature terms and the measurement which major work is tracking the topic whether it emerges or not whereas others put more effort on topic detection while it burst to be a new one. Besides, while the degree of impact authors and publications may have are factored in, the academic position of each topic is also taken into account in the research. The study suggests that detecting results will hence be more precise. (Steyvers, et al., 2004) started to discuss the relationships between the research topics and the authors. They used the Markov chain Monte Carlo algorithm and applied their methodology to a large corpus of 160,000 abstracts and 85,000 authors from the database and learned a model with 300 topics. But they just found out the connections between the topics and the authors. (Jo, et al., 2007) extended the research topics via the correlation between citation graphs and texts. The significant contribution of their work is the separation of the topical words and common words even though they all have high frequencies. In other words, high frequency of a term by itself cannot be seen as the deciding factor for the determination of topics. More tacit knowledge of the topics and citations should be considered. Although Chen’s study (Chen, et al., 2007) still concentrated on the new topics but the pervasiveness and topicality concepts of each topic based on the spread degree of the channel had begun to be considered. We extend those concepts and complement the lacks of their works to propose the emerging research topics detecting approaches.. 立. 政 治 大. ‧. ‧ 國. 學. n. er. io. sit. y. Nat. al. 1.2 Research Issue. Ch. engchi. i Un. v. The research issues could be illustrated in three major parts: (1) identifying research intelligence between conferences and journals, (2) detecting candidate emerging research topics via the Bayesian estimation of author-publication correlations, (3) developing the emerging topic detection indices. 1.2.1 Research Intelligence between Conferences and Journals Academic papers are generally categorized as conference papers and journal papers. These two classifications have features, based on their diverse objectives and functions. The aim of this study of first part is to determine whether the conference papers are helpful for predicting the topics of journal papers. The research also intends to help researchers identify the transition of new trend in their fields. The topics of the papers are utilized as the index to identify their contents. The topics of 5.
(19) conference papers are then adopted to predict those of journal papers. The experimental results demonstrate that the conference papers are useful for predicting the topics of journal papers. The properties of conference papers and journal papers are discussed below. Conferences are forums for scholars of specific fields of studies. A conference paper focuses on the core subject of conference. Many scholars publish their latest research in conference papers, which can then be discussed among renowned scholars in their particular field of study. Research topics acquired through this channel are then employed as the basis for papers later submitted to journals. Given that conferences are held regularly, the research topics discussed in conference papers are subject to current times, making them volatile, but typically novel. Since conferences are reconvened regularly, conference papers are reviewed much more rapidly than journal papers. Therefore, the research topics of a particular field of study are likely to be published and deliberated within the year, making the topics in conference papers volatile and novel.. 立. 政 治 大. ‧ 國. 學. ‧. The acceptance of conference papers are accepted generally depending on the novelty of the topic, rather than the application of strict research methods. Since conference papers are published regularly, the number of articles collected over the period normally exceeds the number published in journals. Furthermore, the routine convention of conferences means that a certain number of conference papers are guaranteed to be published and accepted. The short span of time between publications means that there is little time to practice meticulous research methods. Therefore, the assessment of papers addresses the value of the topic itself more than the precision of research details during an assessment.. n. er. io. sit. y. Nat. al. Ch. engchi. i Un. v. Journal papers are papers published in academic journals. In general, journal papers are subject to a far more meticulous set of assessing standards than conference papers. Journal papers do not have the pressure of regularly publishing deadlines based on routine conference dates, although all academic journals have copy deadlines for editions. Additionally, journal papers have differences in nuance from the regular reconvention and publication of conference papers. Journal papers are academic papers that undergo strict assessment procedures. Many journal papers subject to extensive systems will undertake various procedures. Committee members in charge of assessing journal papers also input suggestions. Researchers must continually edit their work until it coincides with the suggestions of the committee members. Additionally, since the number of publications included in a single edition of an academic journal is less than the number of conferences held in a 6.
(20) year, academic journals can be regarded as the second stage of screening for conference papers. Journal papers lack the volatility and novelty observed in conference papers. Although academic journals are released on set dates, journal papers are submitted according to the researchers’ schedule. The committee members only begin their assessment and review after the researcher has submitted the paper. The editor-in-chief first makes a preliminary assessment. The paper is then reviewed by an expert in the area of study, otherwise known as the advisory editor, for a second stage of assessment. Additionally, the time taken to review conference and journal papers varies. Resubmitting papers after editing is also time-consuming. Therefore, researchers are clearly likely to take longer to publish a journal paper, even though academic journals are released according to set dates. The amount of time invested in this lengthy process usually adds up to over a year, sometimes as much as two to three years. Research topics for journal papers are thus less novel or time-concurrent than conference papers.. 立. 政 治 大. ‧. ‧ 國. 學. Conference papers and journal papers are correlated. Many researchers first publish their research topics as a conference paper. The authors then edit the papers to the journal format and submit it after receiving remarks from fellow scholars in the same area of studies. The journal paper goes through a series of assessment procedures, and is further edited in accordance with committee members’ suggestions. Finally, the paper is accepted and published in an academic journal, helping fellow scholars to generate further knowledge in the future. It is a simple fact that researchers normally publish an early version of their research first in a conference proceeding and then a more complete version later in a journal. This pattern indicates that conference papers represent the beginning of any research process if this pattern was to be followed. However, whether the inspiration for research is inspired by the conference paper, with its capacity for creative topics, or by the journal paper, which is abided by strict and precise research methods, is an interesting question. The two types of paper seem to correlate. This relationship can be further broken down into four categories: Conference papers published in advance create a new trend, affecting conference papers that follow. Conference papers published in advance create a new trend, affecting journal papers that follow. Journal papers published in advance create a new trend, affecting conference papers that follow. Journal papers published in advance create a new trend, affecting journal. n. er. io. sit. y. Nat. al. Ch. engchi. 7. i Un. v.
(21) papers that follow. Different disciplines may include varying correlations between conference papers and journal papers. Observation results of this study demonstrate that the above four types of correlation between conference papers and journal papers may follow different principles, depending on alternate disciplines and fields of studies, since each field of study has a different research culture. For instance, scholars in accounting generally take prominent journal papers as the most important basis of references. In contrast, conference papers are usually publications of research results and topics that lack maturity. Therefore, the mainstream research discussions in the discipline of accounting rely heavily on significant journal papers, because documents built on meticulousness and precision are more likely than those based on innovative research topics to dominate the mainstream in this particular discipline.. 立. 政 治 大. ‧. ‧ 國. 學. The opposite is the case in computer science (computer science, computer science and information engineering, management of information systems), where the innovation in a topic is the most important factor in the acceptance of conference papers. Researchers in this particular area are fairly interested in new topics, since fresh issues symbolize the uncovering of new territory. Innovative research topics suggest many problems have yet to be discovered or solved, and such problems often generate heated deliberations in computer science.. sit. y. Nat. n. al. er. io. The research question is to discuss whether conference papers represent the new trends among academic papers. Table 1-1 show the four descriptors of correlations between conference and journal papers are mentioned above.. Ch. engchi. i Un. v. Table 1-1 The Four Categories of Correlations Between Conference Papers and Journal Papers.. Leading Following. Conference papers Journal papers. Conference papers. Journal papers. C→C. J→C. C→J ˇ. J→J. There are indeed some leading categories between the conference papers and journal papers. The conference papers lead the conference papers which we called C→C. The conference papers lead the journal papers which we called C→J. The journal papers lead the conference papers which we called J→C. The journal papers lead the journal papers which we called J→J. 8.
(22) The researchers most care about is which one could stand in the leading time and the leading trend position could be established firmly. The lack of C→C is researchers almost think the journal papers are more validating than conferences. The J→ C have the time lag since the turn around time of journal papers always longer than conference papers, which we call time lag. Also the J→J have the same problem with time lag. Based on the property of conferences and journals, the C→J without the time lag problems and the trend of journal could convince the scholars to establish the research position. If we could make sure the relation of C→J, it could help the researchers to detect the new trend in conference papers and verify in the journal papers. The conference papers could be the leading indicator. This study focuses on identifying academic papers that seed trends for a particular domain of study by exploring the relationship between conference papers and journal papers. A conference paper appears to mark the beginning of a research process. Therefore, we believe that conference papers represent trends in the overall focus of academic papers.. 立. 政 治 大. ‧. ‧ 國. 學. Searching for research topics for the new trend is a significant issue. Searching and discovering research topics for the new trend is vital to many researchers. Each researcher must cite references of papers as the basic assumption in citation analysis. Hence, some interplay exists between conference and journal papers. Clearly, the issues that are most widely discussed are the ones that have the most impact in a field. The researchers attempt to solve the most urgent and popular issues first. The contribution and impact of this like of issues is bigger. In contrast to non-urgent issues, papers that explore current issues have high acceptance rates. The experiment data are presented by a matrix, with the columns representing features and rows representing papers. This matrix can describe the features of each paper with features, and therefore can be used to calculate the number of papers with each feature. Finally, the Cosine Similarity between annual conference papers and journal papers can be obtained using a unit of measurement in year.. n. er. io. sit. y. Nat. al. Ch. engchi. i Un. v. The topics for the conference papers in the sequential year are investigated to identify any similarities. The research findings strongly support the assumption: 87.23% of the data nodes in 1990–2007 demonstrate that the topic for one year influences topics in future years. These findings indicate that researchers can recognize new trends in research topics in conference papers. Furthermore, massive data can be efficiently processed automatically by computing the similarities between conference papers and journal papers, pinpointing the keywords and topics that would most often appear in future journals.. 9.
(23) The goal of this part is fourfold: First, the research investigates if the conference papers’ themes lead the journal papers’. Second, the research examines how the new research themes can be identified from the conference papers. Third, the research looks at a specific area such as information retrieval and data mining as an illustration. Fourth, the research studies any inconsistencies of the correlation between the conference papers and the journal papers. 1.2.2 Detecting Candidate Emerging Research Topics via the Bayesian Estimation of Author-Publication Correlations. Recently, topic detection has become a very active area of research due to its utility for information navigation, trend analysis, and high level description of data. The past approaches only considered the topic-author relation. The improved method could help researchers distinguish between the topical words and common words. But they had not taken the academic positions into account. This research tries to find out if an author or a publication published or has been cited by lots of papers would be a powerful one to direct future discussions of researchers.. 立. 政 治 大. ‧ 國. 學. ‧. This study aims to help researchers find out the impact an author may have by examining the frequency of published papers and the number of cited papers by the author on the same topic. It is suggested that the more impact an author may have, the more attention and more studies will be generated following the new work of this author. Similarly, the study also investigates the impact a publication may have. The current study of the relative work is to measure the impact factor of a journal, but since a publication will encompass lots of topics, some of them may be weighted heavier in specific fields. If only the impact factor of the journal is used to measure the influence of a publication, it would be unsuitable if only some topics of the publication were mentioned. For example: the MIS (management of information system) researchers may list the top 5 publications in the discipline. But if researchers of MIS discipline want to investigate the field of information retrieval they would not be able to find the top 5 information retrieval publications within the MIS discipline. The computer science discipline will be the more suitable domain for such an investigation even though information retrieval could be the sub field under both MIS and computer science. While impact could be computed in the unit of a topic, it would be more suitable if researchers resorted to searching engines of research paper databases as well. In addition, currently there is still no measure mechanism to gauge the impact conferences may garner. In the study, the impact each conference may have been based on specific topics.. n. er. io. sit. y. Nat. al. Ch. engchi. i Un. v. The emerging topic should be new and important. We can understand that an 10.
(24) important topic should get high grades from authors with significant impact and publications. On the other hand, if we consider only the impact of the author-publication correlation in a topic but do not consider the novelty of the topics, and then this approach may find some topics that are so popular that impact authors or publications can not ignore it anymore and must discuss about it. We only can confirm the impact of the topics we propose but not the emerging potential and future development of the topics. Consequently, we need some tools or indices to help us to assess called emerging topic detection indices. 1.2.3 Developing the Emerging Topic Detection Indices Researchers typically hope their work will contribute to an emerging research topic. Therefore, researchers generally want to identify important and novel research topics, and conduct studies before the topic becomes hot and mature. This study develops novel indices for identifying emerging topics to help researchers to determine whether a topic has potential as a hot topic. This study uses the following case as an example. If there are ten papers discussing a topic, then the impact of a new paper on the same topic can be calculated as 1/11=0.0909. Comparatively, when 1000 papers have discussed a topic, the impact of an addition paper can calculated as 1/1001=0.000999.. 立. 政 治 大. ‧. ‧ 國. 學. n. al. er. io. sit. y. Nat. The concept of novelty was applied in (Zheng et al., 2002) and aging theory was developed by applying a study in TDT (Chen et al., 2003). Those were used when constructing an index for detecting emerging topics. Chen et al. (Chen et al., 2007) used aging theory and term frequency to solve the problem of topic detection, and proved that aging theory was the best solution. Although they claimed that their work can detect a topic while it is emerging, it cannot detect a topic before it emerges.. Ch. engchi. i Un. v. Based on the proposed indices that determine whether a conference or journal paper is a leading trend in research topics, and how long will the leading trend get ahead to the following trend. Those help researchers make decisions about a topic before undertaking research. Conversely, not all new topics are valuable as not all topics become a focus of considerable research. Thus, this study develops a detection table to solve this problem. The study uses novelty, ageing theory, the curve of accumulative relative frequency to develop an appropriate set of index for detecting emerging topics. To our best knowledge this is the first study only for detecting emerging topics. Inductive learning and deductive prediction methods of machine learning help in constructing predictable indices that are feasible. Whether a topic will become is based on discussions in papers during the same period. That is, the volume published 11.
(25) studies on a topic helps determine whether a topic is important. Additionally, whether a topic has emerging potential is based on its novelty. Generally, as the number of times a topic increases, its potential value increases. Hence, a valuable topic attracts the attention of many researchers. Notably, novel topics have considerable content not yet discussed. No matter in empirical or theoretical studies, the novel topics have not completely developed. Applications in the real world are viewed as large space for topic expansion. Consequently, the novelty of and the volume of papers published on a topic are important indices for determining whether a topic has potential to become a hot and emerging topic. The novelty index (NI) and published volume index (PVI) are developed to identify the detection point (DP) of a topic. For the same topic, the period before emerging has the higher NI than the period after emerging. Conversely, the subsequent period has the higher PVI than the previous period. Hence, when a topic has a high published volume, it was an emerging topic. The curve of PVI increases the percentages of publication volume in the previous period and the DP forms in the former period. It represents a topic at an early age as an emerging topic. If delays in published volume are significant, the rise in the curve would be delayed until a subsequent period. Regardless of the time the PVI start increasing, it can be viewed as representation of the topic. Based on the DP of a topic at conferences and in journals, it can help in determining whether conferences or journals are the leading trend of the topic.. 立. 政 治 大. ‧. ‧ 國. 學. n. al. er. io. sit. y. Nat. 1.3 Thesis Organization. Ch. i Un. v. The remaining parts of this research are described as follows. Chapter 2 investigates the related work. Chapter 3 describes the research model of the leading relationship between conferences and journals. Chapter 4 illustrates the experimental results of the relationships between conferences and journals. Chapter 5 proposes the new approach to find out the proposed impact research topics via the Bayesian estimation combined with the impact power authors and publications may have. Chapter 6 shows the experiment and survey results of the Bayesian estimation model. Chapter 7 develops the indices for emerging topic detection. Chapter 8 conducts the research experiment of developing topic detection indices to validate the accuracy and effectiveness of the measurement tool. Chapter 9 gives the conclusion, limitation and future work.. engchi. 12.
(26) Chapter 2 Literature Review The growing development of information technologies and the Internet means that many studies now focus on discovering academic connections among researches. Thelwall (2005) concluded that scientific web intelligence encompasses all of the technologies which extract academic intelligence from the webs, and discussed methods applied in generating such intelligence. Citation analysis was first proposed by Robert Merton, and states that citations represent a cognitive connection between cited and citing papers (Thelwall, 2005). Many investigations have since extended citation analysis to various applications. Those studies apply citation analysis methods to help the researchers to obtain academic intelligence from papers. This approach could help the researchers to deal with the deep research. Conversely, citation analysis could extend to the web applications with hyperlinks. Thelwall et al. (2003) and Thelwall (2004) concluded that links among web pages in universities can measure academic intelligence. Hyperlink analysis is widely performed by text mining or information retrieval approaches to analyze the framework of web pages, (Yang & Lee, 2004), (Yang & Lee, 2005).. 政 治 大. 立. ‧. ‧ 國. 學. 2.1 Topic Detection and Tracking. n. al. er. io. sit. y. Nat. The availability of large linked document collections, such as the web, and specialized literature archives presents new opportunities for mining knowledge about community activities behind document collections. Topic discovery is an example of such knowledge mining that has recently attracted considerable research interest. Topics can be considered semantic units that function as the basic building blocks in knowledge discovery. Once discovered, topics can be utilized in various ways, including information navigation, trend analysis, and high-level data description (Jo et al., 2007). Topic trend analysis has the following three steps: (1) topic structure identification to identify the main topic types and their importance; (2) topic emergence detection to detect the emergence of a new topic and determine how it grows; and, (3) topic characterization to identify the characteristics of each main topic (Morinaga & Yamanishi, 2004).. Ch. engchi. i Un. v. The dissemination and exchange of documents have become commonplace with the recent growth of the Internet, thus raising the significance of content analysis techniques. Topic analysis of, say, e-mails and news articles is an important research task (Cui & Kitagawa, 2005). Many researchers have focused on techniques for topic discovery, topic tracking, topic-based text segmentation and related issues. In addition to TDT, Malone et al. utilized data mining to analyze trends (Malone et al., 2006). 13.
(27) Aurora et al. developed a topic discovery system that reveals the implicit knowledge in news streams (Aurora et al., 2007). Notably, TDT is an information retrieval technology recently developed. It was developed in 1996 when the DARPA was searching for a technique without human intervention to detect topic structures in news streams. A pilot study in 1997 (Allen et al., 1998) laid down the essential groundwork, generating a small corpus and establishing a durable system. In 1998, TDT research flourished (Lee, Lee & Jang, 2007). Makkonen et al. described the techniques applied in TDT cover a large portion of the prevailing techniques in formation extraction, retrieval and filtering, text clustering and text categorization and natural language processing. Notably, TDT uses many common techniques such as in formation extraction, retrieval and filtering, text clustering and text categorization and natural language processing. A TDT system is implemented on-line does not have knowledge of unseen documents, which makes a case for clustering. Some investigations have utilized retrospective topic detection and tracking when a system shows all data simultaneously; however, these studies focus mainly on on-line environments (Makkonen et al., 2004).. 立. 政 治 大. ‧. ‧ 國. 學. n. al. er. io. sit. y. Nat. Allan, Papka and Lavrenko described problems related to new event detection and event tracking within a stream of broadcasted news stories (Allan, Papka, & Lavrenko, 1998). They focused on an on-line setting, i.e., one in which the system makes decisions about one story before analyzing subsequent stories. They used a single-pass clustering algorithm and novel threshold model with event attributes as a major component. Their tracking approach is similar to typical information filtering methods. They determined the value of unique terms that had unusual occurrence characteristics, and applied on-line adaptive filtering to identify the evolution of events in the news. New event detection and event tracking are TDT initiatives.. Ch. engchi. i Un. v. Subsequent studies have improved TDT techniques. For instance, Walls et al. (Walls et al., 1999) developed a system for TDT detection tasks for unsupervised groups of stories in the news and on web pages based on topics. Their system used an incremental k-means algorithm to cluster stories. A probabilistic document similarity metric and conventional vector space model was adopted to compare stories (Salton et al., 1975). Schultz and Liberman proposed approaches for detecting and tracking which is based on the well-known idf-weighted cosine coefficient similarity metric, and achieved excellent tracking results using a very simple term-selection method that did not involve word stemming or score normalization (Schultz & Liberman, 1999) 14.
(28) However, their detection task results were poor, probably due to the poor performance of the clustering algorithm rather than that of the underlying similarity metric. Some investigations have found that while existing learning techniques must be adapted or improved to manage difficult situations in which each event has very few positive training instances, most training documents are unlabelled, and most events have short durations. Yang et al. combined several supervised text categorization methods, namely, several new variants of the k-Nearest Neighbor (KNN) algorithm and the Rocchio approach, to track events (Yang et al., 2000). Their approach, based on a traditional parameter optimization solution, significantly decreased variance in the performance of their event-tracking system for different data collections. Kleinberg proposed a method for analyzing document streams (Kleinberg, 2002). Although the main objective was to detect bursts of topics, the method can be adopted for topic activation analysis. However, Kleinberg’s method only considers document arrival rates, and disregards document relevance. Furthermore, the Kleinberg method is a “batch-oriented” approach. Cui and Kitagawa presented a solution to these problems (Cui & Kitagawa, 2005). Although many studies have improved TDT techniques, these techniques are generally applied to time-sensitive documents (e.g., real-time news and e-mails) and have not been widely applied to identify new topics in academic papers.. 立. 政 治 大. ‧. ‧ 國. 學. y. Nat. sit. 2.2 Emerging Topic Detection. n. al. er. io. An emerging trend is a topic area that is growing in interest and utility over time. For instance, Extensible Markup Language (XML) emerged as a trend in the mid-1990s. Knowledge of emerging trends is particularly important to individuals and companies that monitor developments in a particular field or industry. For example, a market analyst specializing in the biotech industry may want to review technical and news-related literature for recent trends that will impact biotech companies. Manual review of all available data is simply not feasible. Human experts who must identify emerging trends must rely on automated systems as the amount of information available in digital resources is considerable (Berry, 2004).. Ch. engchi. i Un. v. Zhang et al. extended an adaptive information filtering system to make decisions regarding the novelty and redundancy of documents (Zhang et al., 2002). They argued that relevance and redundancy should be modeled explicitly and separately. A set of five redundancy measures was developed and evaluated in experiments with and without redundancy thresholds. Experimental results demonstrated that the cosine similarity metric and a redundancy measure based on a mixture of language models effectively identified redundant documents. Their research focused on the novelty and 15.
(29) redundancy of documents, but did not address research topics. Yang et al. proposed a novel two-stage approach that used (1) a supervised learning algorithm to classify on-line document streams into pre-defined broad topic categories and (2) performed topic-conditioned novelty detection for documents in each topic. They also exploited named entities for event-level novelty detection. Their study used the unit of a topic for novelty detection, but did not discuss when a topic is emerging (Yang et al., 2002). Jo et al. generated unique approach that used correlation between the distribution of a term representing a topic and the link distribution in a citation graph in which nodes are limited to documents containing the term. This tight coupling between a term and graph analysis differed from other approaches such as those using language models. They applied a topic score to each item using the likelihood ratio of binary hypotheses based on a probabilistic description of graph connectivity. Their approach was based on the assumption that if a term is relevant to a topic, documents containing that term have a stronger connection than randomly selected documents. They applied the algorithm to detect a topic represented by a set of terms based on the assumption that if the co-occurrence of terms represents a new topic, the citation pattern should exhibit a synergy. They tested the algorithm on two electronic literature collections, arXiv and Citeseer. Their evaluation results showed that their approach was effective and revealed some novel aspects of topic detection. However, the curve which they only used term frequency to develop was still a backward index for detecting (Jo et al., 2007).. 立. 政 治 大. ‧. ‧ 國. 學. n. al. er. io. sit. y. Nat. 2.3 Aging Theory. Ch. engchi. i Un. v. Capturing variations in a distribution of key terms on a time line is critical when extracting hot topics. Therefore, tracking terms to determine their lifecycle stage is essential. Previous studies have recognized that topics in a continuous document stream can be identified via a simultaneous temporal burst of related documents. There was a research applied Aging Theory to model the life span of a news event, and suggested that a news event can be considered a life form that goes through a lifecycle of birth, growth, decay, and death, reflecting its popularity over time. They utilized the concept of energy to track event lifecycles. The level of energy indicates the stage of a news event in its life span. The energy of an event increases when the event becomes popular and decreases as its popularity wanes. Hence, aging theory is suitable for tracking variations in term frequency, which we consider critical to successful hot topic extraction (Chen et al., 2007).. 16.
數據

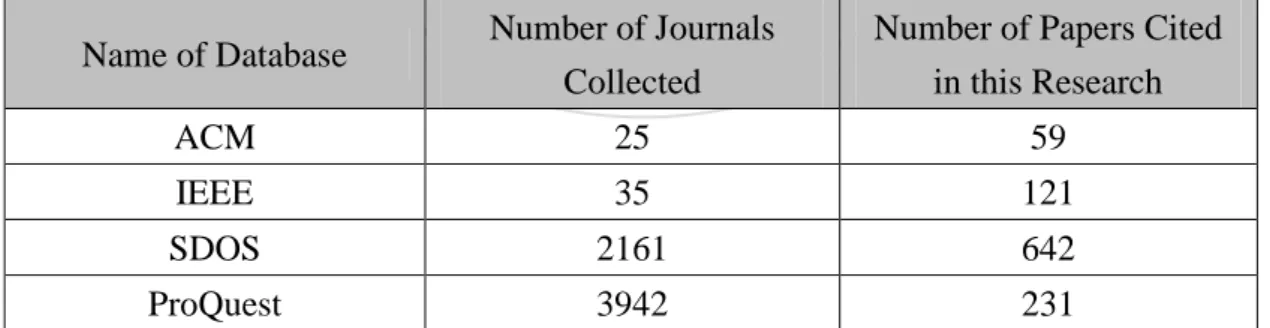
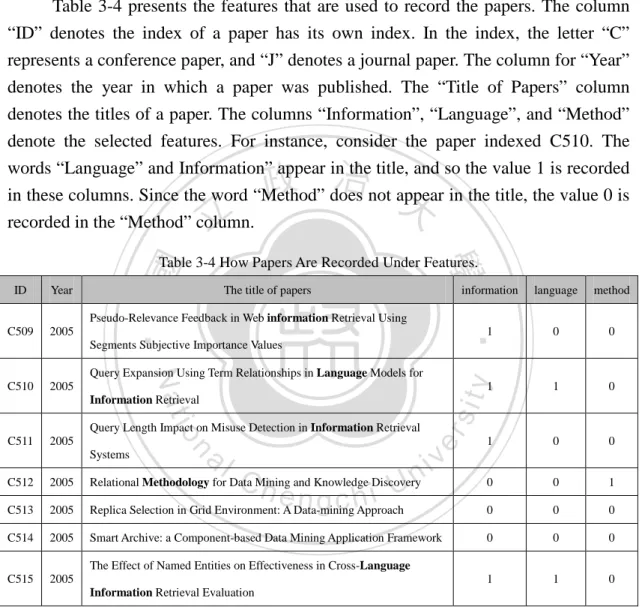

相關文件
You are given the wavelength and total energy of a light pulse and asked to find the number of photons it
substance) is matter that has distinct properties and a composition that does not vary from sample
Promote project learning, mathematical modeling, and problem-based learning to strengthen the ability to integrate and apply knowledge and skills, and make. calculated
- Informants: Principal, Vice-principals, curriculum leaders, English teachers, content subject teachers, students, parents.. - 12 cases could be categorised into 3 types, based
Wang, Solving pseudomonotone variational inequalities and pseudocon- vex optimization problems using the projection neural network, IEEE Transactions on Neural Networks 17
volume suppressed mass: (TeV) 2 /M P ∼ 10 −4 eV → mm range can be experimentally tested for any number of extra dimensions - Light U(1) gauge bosons: no derivative couplings. =>
Define instead the imaginary.. potential, magnetic field, lattice…) Dirac-BdG Hamiltonian:. with small, and matrix
incapable to extract any quantities from QCD, nor to tackle the most interesting physics, namely, the spontaneously chiral symmetry breaking and the color confinement..