Enterprise Application Integration –the Case Study for a Financial Holding Company
全文
(2) In order to find out those 20% clients in FHC, we have to integrate customers information from various systems in heterogeneous platforms, calculate customers’ contribution within the FHC subsidiary information.. Keyword:Financial Holding Company、Data Integration、Enterprise Data Warehouse. 1. 1.1.. INTRODUCTION. We focus on the data integration within legacy systems of the enterprise. Data from the various systems are aggregated into a data warehouse (DW). Using the concept and technique of data warehousing to build a cross-platform, cross-business Enterprise Data Warehouse (EDW) of FHC, we can provide a single view of customers’ personal and financial information for executive to make decision correctly and efficiently.. Background. Due to the deregulation, internationalization and conglomeration of financial institutions in recent years, conglomerated merger and acquisition becomes the worldwide tendency. In order to prompt changes of financial environment, to increase the synergy of financial institutions, to consolidate the supervision of cross-financial industry, to promote the sound development of financial markets, and to protect the public interest The government has passed the ‘Financial Holding Company Act’ to help financial institutions to improve financial institutions competitiveness in 2001.. With customer information that is complete, up-to-date, and easily available, we can better understand our customers, and the better we understand our customers, the better we can offer them products and services that they want and can afford.. A bank’s subsidiary can cross sell diversified financial products of its members of financial holding company to satisfy customers with one-stop shopping services. At the same time, they can effectively employ resources and information manipulation alternative to reduce cost. The financial holding company is equipped with much more efficiency and advantage of scale economy than the individual financial institutions. 1.2.. 2. RELATED WORKS 2.1 Domain Definition 2.1.1.. According to the Article 4 of the Financial Holding Company Act [1], the meaning of ‘Financial Holding Company’ and other relevant terms are as follow:. Motivation. In order to consolidate the subsidiaries business operations of a Financial Holding Company (FHC), such as banking, securities-trading, insurance, etc., the FHC has faced the challenge of integrating application functionality across multiple platforms and business subsystems while presenting a consistent and transparent interface to customers.. ‘Controlling Interest’ shall mean holding voting-right-shares or capital stock of a Bank, Insurance Company or Securities House more than 25% or otherwise having the direct or indirect ability to designate the majority of the directors of a Bank, Insurance Company or Securities House. ‘Financial Holding Company’ shall mean a company established in accordance with this Act and having a Controlling Interest in a Bank, Insurance Company and/or Securities House. (Figure 2.1). To meet this challenge, the information department of FHC needs to integrate all the data within the enterprise and build an Enterprise Application Integration (EAI) solution for cross-platform integration and for storing cross-application data. An employee of FHC, such as account officer of the bank subsidiary of FHC, may access consolidated customer information as a single view. 1.3.. Financial Holding Company (FHC). ‘Financial Institution’ shall mean any of the following Banks, Insurance Companies and Securities Houses: ‘Banks’. Banks and bills finance companies as defined in the Banking Act and other entities designated by the Ministry of Finance.. Objectives. The 80-20 rule states that for many phenomena 80% of consequences stem from 20% of the causes. That assumption is that most of the results in any situation are determined by a small number of causes. That idea is often applied to data such as sales figures: ‘20% of clients is responsible for 80% of sales volume.’. ‘Insurance Companies’. Insurance enterprises established in accordance with the Insurance Law and organized as companies limited by shares.. 2.
(3) ‘Securities Houses’. Securities houses licensed to engage in securities underwriting, trading-on-one-account and brokering or securities finance companies engaging in a securities finance business.[1]. Cross-Selling V.S. Business Effectiveness. Insurance Counter. Front-end Integration Employee sharing Office sharing Facilities sharing. Security Counter. Banking Counter Insurance Subsidiary. Financial Holding Company Organization Chart. Information Sharing Customer Integration Back-end Integration. Security Subsidiary. Banking Subsidiary. Financial Holding Company. Figure 2.2 Cross Selling V.S. Business Effectiveness [3] Bank. Insurance. Security. Bill. Trust. Venture Futures Capital. …. 2.2.. Enterprise application integration (EAI) is the use of software and architectural principles to integrate a set of enterprise computer applications. EAI helps to integrate applications inside an organization, or application of different organizations in a seamless fashion. EAI is related to middleware technologies such as message-oriented middleware, and data representation technologies. The EAI technologies involve using web services as part of service-oriented architecture as a means of integration.. Figure 2.1 Financial Holding Company Organization Chart 2.1.2.. Enterprise Application Integration. Cross-Selling. The essential function of a bank is to provide services related to the storing of deposits and the extending of credit. The evolution of banking dates back to the earliest writing, and continues in the present where a bank is a financial institution that provides banking and other financial services.. Without integration, enterprise computing often takes the form of islands of automation, where the value of individual systems is not maximized because they are working in partial or full isolation.. Currently the term bank is generally understood as an institution that holds a banking license. Banking licenses are granted by financial supervision authorities and provide rights to conduct the most fundamental banking services such as accepting deposits and making loans. There are also financial institutions that provide certain banking services without meeting the legal definition of a bank, a so called non-bank. Banks are a subset of the financial services industry. [7]. With EAI each application only requires one connection, which is to the bus. Attending to EAI involves looking at the system of systems. Such message bus approaches can be extremely scalable, and also highly evolvable. EAI is not just about sharing data between applications. EAI focuses on sharing both business data and business processes [7].. The goal of FHC is to offer customers a one-stop shopping experience, through which they can access all the products and services they want at one time, from wherever they reside, and using a method of their choice. The strategy of pushing new products to current customers based on their past purchases, cross-selling is designed to widen the customer's reliance on the company and decrease the likelihood of the customer switching to a competitor.. 2.3.. Data Warehouse. A data warehouse is a repository of historical data, subject-oriented and organized, summaried, and integrated from various sources so as to be easily accessed and manipulated for decision support [5]. W. H. Inmon, who is known as the ‘father of the data warehouse’, defines the data warehouse as ‘a subject-oriented, integrated, time-variant, nonvolatile collection of data in support of management decisions’ [8].. In order to meet the goal of FHC, combining the data warehousing and analytical capabilities technique is the only solution for that.. Subject-oriented: Focus on natural data groups, not applications boundaries. In the data warehouse, data is not stored by operational 3.
(4) applications, but by business subjects.. Data Warehouse Architecture. Integrated:. Data Acquisition. Data Storage. Data Source. Provide consistent formats and encodings. Data inconsistencies are removed; data from diverse operational applications is integrated.. Information Delivery Application. Warehouse Data. External Sources. Report Operational Database. Time-variant: A data warehouse has to contain historical data, not just current values. Data is organized by time and is stored in diverse time slices.. Extract Transform Load Refresh OLAP Cube. Internal private Data. Data Mining. Data Marts. Nonvolatile: Figure 2.3 Data Warehouse Prototype Data Source Architecture [2]. No updates are allowed, only load and retrieval operations. Usually the data in the data warehouse is not updated or deleted. [6]. In data acquistion area covers the entire of extracting data from the data sources, moving all the extracted data to the staging and preparing the data for loading into the data warehouse repository. The major component ‘Data Source’ means:. DW often hold large amounts of information which are sometimes subdivided into smaller logical units called dependent data marts. Generally Speaking, there are two basic guidelines to build a DW: Integration of data from distributed and differently structured databases, which facilitates a global overview and comprehensive analysis in the data warehouse.. Operational source systems Computing platforms, operating systems, databases, files Departmental data such as files, documents, and spreadsheets. Separation of data used in daily operations from data used in the data warehouse for purposes of reporting, decision support, analysis and controlling. [7]. External data sources Another major component in data acquisition area is ‘Data Staging’, it contains:. DW is a process, not a product. It is a technique to properly assemble and manage data from various sources to answer business questions not previously possible or known. Data from cross-platform and cross-business systems into the data warehouse for further processing. It is common practice to ‘stage’ data prior to merging it into a data warehouse. To ‘stage data’ means to queue it for preprocessing, usually with an ETL tool. The preprocessing program reads the staged data, often a business's primary On-Line Transaction Process(OLTP) databases, performs qualitative preprocessing or filtering, and writes into the data warehouse.. Data mapping between data sources and staging area data structures Data transformations Data cleansing Data integration In data storage area covers the process of loading the data from the staging area into the DW repository. All functions for transforming and integrating the data are completed in the data staging area. The prepared data in the DW is like the finished product that is ready to stack in an industrial warehouse, in this area we need to consider about:. In Figure 2.3, there are three major data warehouse area named data acquisition, data storage and information delivery.. Size of extracted and integrated data DBMS features Growth potential Centralized or distributed ‘Information Delivery’ spans a broad spectrum of different methods of making information available to users. For end-users, the information delivery component is the ‘DW’. They do not come into contact with the other components directly. Most of the information 4.
(5) on a specific, predefined need for a certain grouping and configuration of select data. Since data mart configuration emphasizes easy access to related information, the star schema is a fairly popular design choices, it enables a relational database to emulate the analytical functionality of a multidimensional database.[6]. access in a DW is through online queries, interactive analysis or producing regular and ad hoc report. 2.4.. Extract, Transform and Load (ETL). Data Warehouse acquisition processes that involves extracting data outside sources with cross-platfrom and cross-business, transforming (or transporting) data to fit business needs, and loading data from source systems into the data warehouse. Extraction, transformation and loading are the activities that required to populate data warehouses and On-Line Analytical Processing (OLAP) applications with clean, consistent, integrated and probably summarized data. [6]. 2.6.. Star Schema. The star schema is a fact table in the middle connected to a set of dimension tables. It is the simplest data warehouse schema where a single ‘fact table‘containing a compound primary key, with one segment for each ‘dimension’ and with additional columns of additive, numeric facts. The star schema makes multi-dimensional database functionality possible using a traditional relational database.. Extract The first phase of an ETL process is to extract the data from the source systems. Most data warehousing projects consolidate data from different source systems. Each system may use a different data format. Common data source formats are relational databases or flat files, but other source formats exist. Extraction converts the data into records and columns.. Fact tables in star schema are mostly in third normal form, but dimensional tables in de-normalized second normal form. Normalizing dimensional tables will look like snowflakes and the complex queries and business users cannot easily understand the meaning of data. Though query performance may be improved by DBMS technology and hardware, highly normalized tables make reporting difficult and applications complex.. Transform The transform phase applies a series of rules or functions to the extracted data to derive the data to be loaded. Some data sources will require very little manipulation of data. However, in other cases any combination of the following transformations types may be required: Select only certain columns to load or reject columns not to load Translate coded values. Derive a new calculated value. Join together data from multiple sources. Summarize multiple rows of Generate a Surrogate_key value. data.. Figure 2.4 Star Schema [4]. Transpose / cross tabulates, turn multiple columns into mutiple rows.. 3. REQUIREMENT DEFINTION. Load 3.1.. The load phase loads the data into the data warehouse. Some data warehouses only overwrite old information with new data. More complex systems can maintain a history and audit the track of all changes to the data. 2.5.. The Process of Developing a Data Warehouse. The process of developing a data warehouse shown in Figure 3.1 is similar in many respects to any other development project. Therefore, the process follows a similar path.. Data Mart. A data mart is a specialized version of a data warehouse. Data marts contain a snapshot of operational data that helps business people to strategize based on analyses of past trends and experiences. The key difference with data warehouse is that the creation of a data mart is 5.
(6) interactions and views is often made more complex by the number of personal relationships and informal channels into and out of the organization.. Data Warehouse Development Life Cycle Define the Project. Manage the Project. Requirements Gathering. Implement the Warehouse. Modeling the Warehouse. Design Validation. Model Validation. This affects customer interactions, and again serves to further increase the number of views a customer holds of the one company. The process chains is not streamlined and efficiency. Customer data is spread over across systems and platforms, containing inconsistent and invalid values. In a mirror image of the process fragmentation, information is held within the organization in many locations and systems and in many cases is duplicated and possibly even conflicting. Inconsistence and duplication of tasks and processes has been increase more and more for organization.. Design the Warehouse. Figure 3.1 Data Warehouse Development Life Cycle [4] 3.2.. The As-Is Situations. As we know, most information systems of subsidiaries had been set up before building an EDW. These OLTP and legacy systems were developed to meet end-users’ requirements in each business unit. Business processes are often deep-rooted, having evolved and formed as the business has grown and developed. However, these processes or systems limit further improvements and need to be replaced or modified to support future development. Unfortunately, major changes in strategy are often expensive and risky and tend to be implemented slowly. Smaller increments of change can often create a much stronger long-term solution.. 3.3.. Requirements. A company's survival depends on its ability to maximize customer value and customer loyalty by effectively marketing additional products and services to customers. To combine the technique of data warehousing and analytical capabilities to help identify new sources of revenue and determine ‘what’s next?’ for specific customers. We must find more effective ways to cross-sell and up-sell to new and existing customers in order to improve campaign response rate, generate more revenue and improve customer loyalty. Despite this need, we still sometime struggle to execute effective cross-selling strategies. Customer data that would help identify good candidates for cross-sell and up-sell is often scattered and containing, inconsistent and sometime invalid throughout the enterprise, making it impossible to get a clear single view of customer preferences and behavior.. These systems have valuable information, but it is difficult and slow to extract meaningful information from them. While these systems can usually support pre-defined operational reports, they usually cannot support an organization's need for historical, consolidated, smart or easy-to-access information. Data is spread over many tables, across many systems and platforms, and often the data is ‘dirty’, containing inconsistent and invalid values, making analysis impossible.. To maximize the value of every customer relationship and improve customer loyalty, we focus on collecting and integrating data from cross-platform and cross-business. It is important that building an EDW to provide a so-called 360-degree view of the customers and tracking, storing, and predicting customer behavior. By the ways, to Access to atomic data, delivered from operational systems in real time, and seamlessly connected to old history.. However, these systems are not suitable to view a combination of claims data in response to ad hoc or business-based questions. Furthermore, they cannot look at claims information in combination with information contained in associated systems outside the claims system, such as policy owner or service provider data.. We could enable account officer to share customer intelligence with intermediaries, helping them to target appropriate customers for additional offers.. Besides, to keep the customer relationship and improve customer loyalty many departments of subsidiaries have to interact with customers but individually, creating many internal and external views of the same customer in different systems. Each view is separately maintained and is not only different, but can often conflict. This highly complex matrix of relationships, 6.
(7) operational systems using replication and other techniques.. 4. SYSTEM DESIGN In this section, we design a prototype system based on the requirements that end-users defined in Requirements Design Section. First, we scratch the data source architecture for data ETL flow and data models of each business area. And then we extract the data from source system, transform data and verify data with business rules end-user defined, and load the data to EDW.. Create intermediary files to store selected data to be merged later Transport extracted files from multiple platforms. Provide automated job control services for creating extract files Reformat input from outside sources.. As we know, for end-users, information delivery is the ‘DW’. Most of the information access in an EDW is through online queries, interactive analysis or producing regular and ad hoc reports. 4.1.. Reformat input from departmental data files, databases, and spreadsheets. Generate common application code for data extraction. Resolve inconsistencies for common data elements from multiple sources.. Data Source Architecture. In this case, we have to collect customer events in each business unit, such as deposit balance or interest in bank, commission in stock-trading, etc., and calculate customer contributions for each product. Because of heterogeneous system platforms and databases even various business attribute, at first we consider to transfer extracted files we need through FTP channel. The information department of each business unit needs to generate those extracted flat files by submit daily, weekly or monthly jobs that DW system needs and put those files on FTP servers. 4.2.. 4.2.2.. Data Transformation. Map input data to data fro data warehouse repository. Clean data, de-duplicate, and merge/purge De-normalize extracted data structures as required by the dimensional model of the data warehouse. Convert data types. Calculate and derive attribute values. Check for referential integrity.. ETL Procedure. Aggregate data as needed.. In staging area, all the extracted data from different legacy systems on multiple platforms or external sources is put together through FTP channel and stand by to load into DW.. Resolve missing values.. To examine each extracted flat file, review the business rules, perform the various data transformation functions, sort data, merge data, resolve inconsistencies and cleanse the data in this phase. After preparing for EDW, data temporarily resides in the staging area repository waiting to be loaded into the DW repository.. Using database utilities to load all extracted data files into EDW or submit programs of embedded SQL commands to refresh the data in EDW.. Consolidate and integrate data. 4.2.3.. 4.3.. Data Extraction. Select data sources and determine the types of filters to be applied to individual sources. Generate. automatic. extract. files. Data Model. As we show in Figure 4.1, some customer data is overlapped in subsidiaries of F.H.C. It is an important issue that how to integrate and keep customer data consistency in EDW. We design a draft of data model based on customer level and drill down to account level in Figure 4.2. Data in the staging area is kept as flat files. The flat files contain the integrated and cleaned data in appropriate formats ready for loading. These files are in the formats that can be loaded by the utility tools of the DW RDBMS. The staging area contains data at the lowest grain because of the business measurements. We need to aggregate data in the staging area from loading. We discuss data extraction, data transformation and data load in this section: 4.2.1.. Data Load. from 7.
(8) 4.4.1. Staging Area. Data Model. In data staging area we need to consider as follow:. Customer data Bank Bill. Provide backup and recovery for staging area repositories. Security. Sort and merge files. Create and populate database.. Insurance. Trust. Preserve audit trail to relate each data item in the data warehouse to input source. Resolve and create primary and foreign keys for load tables. Figure 4.1 Customer Data Overlap in Subsidiary of F.H.C. Generate surrogate key for each target table. Consolidate datasets and create flat files for loading through DBMS utilities. Extract load files prepare for loading data to DW repository.. Data Model customer contribution. customer campaign info. 4.4.2. Data Storage. customer account daily balance. account monthly balance. account. In data storage area, we stress on:. account contribution. Loading data for full refreshes of DW tables Perform incremental prescribed intervals. account detail. Provide automated job control services for loading the DW. Set up ‘corntab’ parameter or running Shell program as daemon program background.. Infrastructure and System Architecture. As show in Figure 4.3, we set up a FHC EDW with IBM DB2 on IBM e-Series Server. We build the temporary relational tables in staging area on the same physical machine.. Provide backup and recovery for the data warehouse database to backup device. Ex: tapes or Storage Area Network. Monitor and fine turn EDW database 4.5.. Software Architecture. Application Operational Data. AIX 5L DB2 Warehouse Manager DB2 Connect EE DB2 OLAP Server Brio On Demand Server for Unix IBM HTTP Server. Intranet. Information Delivery. Most of the information access in an EDW is through online queries, interactive analysis or producing regular and ad hoc report. The success of EDW rides on the strengths of the information delivery tools. There are four ways for information delivery:. Window Client Application / Internet Explorer. Analytical Data. regular. Optimize the loading process. Figure 4.2 Data Model of FHC. EDW DB2. at. Support loading into multiple tables at the detailed and summarized levels. trans log. 4.4.. loads. Application:. Report. A decision support application in relation to the data warehouse is any downstream system that gets its data feed from the data warehouse. In this case, we build an application to integrate customer assets and liabilities in FHC as a 360-degree view.. OLAP Cube. Data Mining. Figure 4.3 Software Architecture. Report: We push all the information to end-user by report, not pulled by users as in case of queries. All the report is pre-defined by IT 8.
(9) c. Receives input files and convert into one consistent data format. members. User subscribes the report by e-mail, so we will establish a proper distribution system to handle this.. d. Calculate and derive attribute values. And check for referential integrity.. OLAP Cube:. e. The extracted file is at the lowest grain because of the business measurements. We aggregate data summary data as needed before loading.. To set up a multi-demission data mart for OLAP cube. Monthly, we generate aggregated data from EDW and load into the analytical data mart. And then run jobs to generate OLAP Cubes for end-users.. f. Resolve missing values.. Data Mining:. g. Generate a surrogate key by database utility or user-defined function to identify customer as an unique key in database. It is also known as knowledge-discovery in databases, is the practice of automatically searching large stores of data for patterns. To segment cusomter or predict customer’s behavior is the most popular subject of data mining.. 5.3 Data Load After extraction and transformation, the files are in the formats that can be loaded by the utility tools of the DW RDBMS. 5.3.1 Initial Load. 5. CONSTRUCTION. Load mode:. In this Section, we extract, transform and load data that end-user required into the FHC EDW and develop a prototype system to present a 360-degree view of customer.. For the first run of the DW, we choose load mode for most DW tables. Append mode. 5.1 Data Extraction. Then all further runs will apply the incoming data using the append mode.. Here all the extracted data from different OLTP or legacy systems on multiple platforms or external sources is put together on FTP server. The subsidiaries generate flat files as a routine job, through FTP channel put those files on FTP server.. 5.3.2 Incremental Loads Ongoing changes to the OLTP system always tied to specific times, irrespective of whether or not they are base on explicit timestamps in the OLTP systems. We need to preserve the periodic nature of the changes in DW.. a. OLTP and legacy system data ongoing change in customer-level, account-level and transaction-level.. Destructive Merge. b. Internal data: self-design worksheet by PC each business unit or not computerized worksheet in private. In destructive mode, we apply the incoming data to the target data. If the primary key of an incoming record matches with the key of an existing record, update the matching target record. If the incoming record is a new record without a match with any existing record, insert the coming record to the target table.. c. Archived data: OLTP or legacy system historic data on off-line storage device. d. External data: bureau information form external organization. By running shell program as a daemon program, we can automatically to get flat files from each subsidiaries FTP server and put to the certain staging directory on staging area server.. Constructive Merge a.. If the primary key of an incoming record matches with the key of an existing record, leave the existing record, add the incoming record, and mark the added record as superceding the old record. Till now, we do not use this kind merge in our system.. b.. If the time stamp is part of the primary key or if the time stamp is included in the comparison between the incoming and existing record, the constructive merge would be used to preserve the periodic. 5.2 Data Transformation In this stage, we need to cleanse and transform each extracted files into one consistency format as follow: a. Cleanse data Review the business rules, verify fields each extracted files, removing errors. b. Merge and sort input files 9.
(10) To integrate data from distributed, heterogeneous databases and platforms.. nature of the changes. 5.4 Information Delivery. To make a global comprehensive analysis warehouse.. We construct a prototype customer financial management system as information delivery tool for account officers in FHC. We can get customer assets and liabilities as a clear and single view.. overview in the. and data. Base on the subsidiaries domain knowledge to define the formulas for customers’ contribution in each subsidiary, calculate and integrate these information to rank all the customers’ contribution. Using information delivery tools (e.g. ad-hoc query or report) to find out those ‘20% of clients are responsible for 80% of sales revenue’.. As the authority concern, we grant the suitable privilege to login user. If the end-user is an employee of FHC and has been authorized to login the system, he or she can access all the customer data in FHC EDW. If the users are account officers of subsidiary, they can only access the customer data of their company, no other subsidiaries information with this customer, even all this customer data are stored in FHC EDW. The functions of this prototype system we design in this study case are:. Instead of using data on sources system, using data on enterprise data warehouse for reporting, decision-making support and analysis can decrease the overhead on OLTP systems.. Login function using subsidiary ID and employee number.. In this thesis, we build a data warehouse of Financial Holding Company with star schema model. In order to integrate data and to improve the efficiency of data analysis and reports we consolidate the enterprise transactional and operational information across various business units and platforms.. Key in customer number to inquiry a customer name and cross-selling information. Inquiry customer personal information. Inquiry customer contribution information in FHC and subsidiaries.. We extract data from subsidiaries, transform data, varify the consistency of data and loading data to FHC data warehouse. Then we construct prototype application as an information delivery tool to present customer information with a single view for the FHC staff to make decision more correctly and efficiently.. Inquiry all bank account information of the customer. Inquiry bank NTD deposits account of the customer. Inquiry bank trust fund account of the customer.. 6.2 System Restriction Considerate the information security, customers personal privacy and not violating the ‘Financial Holding Company Act’ and ‘Act of Computer Processing of Personal Data And its Protection’, customer data is totally independent in each subsidiary of FHC. Therefore, customer information sharing in FHC DW will base on the customer's agreement. If a customer disagrees to offer any personal / financial information for cross-selling, we cannot use his or her data for any cross-selling marketing activities. [1]. Inquiry bank NTD loan account of the customer. Inquiry bank loan account of the customer. Inquiry bank card account of the customer. Inquiry bank card account of the customer. Inquiry bill account information of the customer. Inquiry insurance account information of the customer. 6.3 Future Works. Inquiry security account information of the customer. The new Basel Accord (Basel II) is being implemented in 2006. Therefore, it is significant to integrate data within subsidiaries. Building a data warehouse of financial holding company from the heterogeneous platforms and databases for cross-business is really a big challenge.. 6. CONCLUSION AND FUTURE WORKS. In the study we focused on customer data. For the future works, we should pay more attention to credit and risk information for risk assessment. To combine and integrate operational data and risk data horizontally and. 6.1 Conclusion Generally speaking, we build up an enterprise data warehouse for the purpose below:. 10.
(11) vertically will help risk management departments calculate risk factors, such as own capital, credit risks and assets operational risk, etc. Furthermore, calculate the impact of The New Basel Capital Accord on bank’s capital adequacy ratio for risk assessment of Financial Holding Company.. [4] Chuck Ballard, Dirk Herreman, Don Schau, Rhonda Bell, Eunsaeng Kim, Ann Valencic, Data Modeling Techniques for Data Warehousing, IBM Redbooks February 1998 [5] Efraim Turban, Ephraim Mclean, James Wetherbe, Information Technology for Management Transforming Organizations in the Digital Economy 4th Edition., John Wiley & Sons, Inc ,2004, P500-505. BIBLIOGRAPHY [1] 行政院金融監督管理委員會 銀行局, 「金融控股公司法相關法令彙編」,九 十三年十一月版 http://www.boma.gov.tw/. [6] Paulraj Ponniah, Data Warehousing Fundamentals, A Comprehensive Guide for IT Professionals, John Wiley & Sons, Inc., 2001. [2] 陳煇煌,大同大學, 「資料倉儲-Chapter 4 Planning and Project Management」 ,上課 講義,2002. [7] The Wikipedi Fundation, The free encyclopedia, http://en.wikipedia.org/wiki/Main_Page.. [3] 彭金隆博士,政治大學金融學系「金融. [8] W.H. Inmon, Building the Data Warehousing, 3rd Edition, John Wiley & Sons, Inc., 2002. 控股公司的組織與策略:我國金融控股 公司之特性」課程教材 http://www.banking.nccu.edu.tw/data/cour se/bank%20holding/金控公司之特性 (II).ppt. 11.
(12)
數據
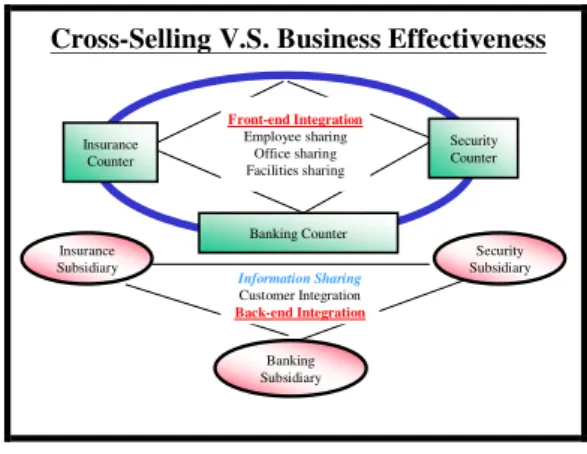
![Figure 2.3 Data Warehouse Prototype Data Source Architecture [2]](https://thumb-ap.123doks.com/thumbv2/9libinfo/8915003.261113/4.892.460.753.99.325/figure-data-warehouse-prototype-data-source-architecture.webp)
![Figure 2.4 Star Schema [4]](https://thumb-ap.123doks.com/thumbv2/9libinfo/8915003.261113/5.892.464.755.624.817/figure-star-schema.webp)
![Figure 3.1 Data Warehouse Development Life Cycle [4]](https://thumb-ap.123doks.com/thumbv2/9libinfo/8915003.261113/6.892.134.438.103.320/figure-data-warehouse-development-life-cycle.webp)
相關文件
Create and present information and ideas for the purpose of sharing and exchanging by using information from different sources, in view of the needs of the audience.
Time constrain - separation from the presentation Focus on students’ application and integration of their knowledge. (Set of questions for written report is used to subsidize
prevent cruelty and alleviate suffering, and through education to cultivate a deep respect for life in the community so that all living creatures may live.. together
Discovering the City by Mining Diverse and Multimodal Data Streams – IBM Grand Challenge: New York City 360. § Exploring and Integrating Multiple Contents and Sources for
The remaining positions contain //the rest of the original array elements //the rest of the original array elements.
The presentation or rebranding by a company of an established product in a new form, a new package or under a new label into a market not previously explored by that company..
– It allowed a commercial bank, investment bank, and insurance company to merge and form a financial holding company.. – To serve all their customers’ financial needs, bank
The Performance Evaluation for Horizontal, Vertical and Hybrid Schema in Database Systems.. -A Case Study of Wireless Broadband