以台灣指數期貨市場為例-研究各類投資人從眾行為與回饋交易行為及交易量大小之資訊效果 - 政大學術集成
全文
(2) 誌謝 論文完成的這一刻,心中充滿無比的歡心與感謝但也夾帶淡淡的離愁, 這部作品記錄著我碩士生涯研究的歷程,卻也代表學生時代將要畫下圓滿的 句點。首先我要感謝我的家人,這半年因有眾多外務而無法回家,但你們總 是默默的鼓勵與支持,讓我有動力完成論文。另外我要感謝我的指導教授 周冠男所長,在老師的循循善誘下,讓我了解到做學問的真諦!也要感謝幫我 論文口試的劉祥熹教授、徐之強教授及何耕宇教授給予我論文的指教與建 議。. 政 治 大. 再來我要謝謝與我同研究室的育賢、欣頤及家齊,很高興能與你們互相. 立. 學習,也常常彼此督促對方的進度,才讓我的論文進度得以如此順利!也要謝. ‧ 國. 學. 謝我的好友淑妘、高瑋、紹奎、昌歷、宗霈、豐羿及乃辰的鼓勵及陪伴,讓 我碩士研究生涯如此充實與歡樂!. ‧. 另外我要謝謝鈞元、柏森、劍洲給予我統計軟體上的協助!感謝楚彬學長、. y. Nat. sit. 雅晴學姊及雅凱學姊,謝謝你們撥空時間指導我,給予我論文寶貴的建議。. n. al. er. io. 謝謝我在交大及嘉中的好友俐婷、曉蓉、易群、威廷、富民、怡汝及. i n U. v. 瓊嬅學妹,時時不忘寄卡片或捎來簡訊問候我的近況及關心我的論文進度。 謝謝你們!. Ch. engchi. 最後感謝所有曾經幫過我的人,感謝您們,因為有您們才讓我論文如此 順利地完成,再次謝謝大家。. 黃彥銘. 謹致. 政治大學財務管理研究所 中華民國一百零二年六月.
(3) Abstract The purpose of this study is to investigate herding and feedback trading by different trader types and the effect of their trade size on Taiwan Capitalization weighted stock index futures contracts on the Taiwan Futures Exchange. The empirical results demonstrate that there are different trading patterns between large and small trade size for individual investors as well as institutional investors.. 政 治 大. For the entire period, individual investors are positive feedback traders, and. 立. institutional investors are negative feedback traders. We divide individual and. ‧ 國. 學. institutional investors into large and small groups. We find that large investors’. ‧. trading behavior is independent and different from trading behavior of average. Nat. io. sit. y. investors who belong to their trader type. This may result from the fact that large. er. investors are the informed. Compared to large investors, small investors are. al. n. v i n C hengage in feedback less-informed traders, and they e n g c h i U trading to get information. As for herding, we observe that small institutional investors have the most significant herding behavior among all types of investors because of competition among them and reception of the similar information. We also find that investors react asymmetrically to periods of contraction and expansion. Small investors would intensify their herding and feedback trading during a period of expansion. Keywords: herding, feedback trading, trader types, trade size, business cycle I.
(4) Table of Contents 1.. Introduction .......................................................................................................... 1. 2.. Literature Review and Hypotheses .................................................................... 4 2.1 Herding and Feedback Trading ...................................................................... 4 2.2 Herding and Feedback Trading by Individual Investors ................................ 7 2.3 Herding and Feedback Trading by Institutional Investors ............................. 9. 政 治 大 Hypothesis.................................................................................................... 14 立. 2.4 Herding and Feedback Trading in different periods .................................... 12. 學. 3.. ‧ 國. 2.5. Data and Methodology ...................................................................................... 14 3.1 Data Description .......................................................................................... 15. ‧. y. sit. Empirical Results ............................................................................................... 21. io. n. al. er. 4.. Nat. 3.2 Methodology ................................................................................................ 17. i n U. v. 4.1 Herding and feedback trading by individual investors and the effect of. Ch. engchi. trade sizes ..................................................................................................... 21 4.2 Herding and feedback trading by institutional investors and the effect of trade sizes ..................................................................................................... 23 4.3 Herding and feedback trading in different periods ...................................... 25 4.4 Herding and feedback trading by different institutional investors ............... 27 5.. Conclusions ......................................................................................................... 29. References ................................................................................................................... 43 II.
(5) List of tables Table 1 Descriptive statistics per contract by trade type categories ....................... 31 Table 2 Descriptive statistics per contract by trade size categories ........................ 32 Table 3 Descriptive statistics of individual investors.............................................. 33 Table 4 Analysis for herding and feedback trading by individual investors for a full business cycle ........................................................................................... 34. 政 治 大. Table 5 Analysis for herding and feedback trading by individual investors during a. 立. period of contraction ................................................................................. 35. ‧ 國. 學. Table 6 Analysis for herding and feedback trading by individual investors during a. ‧. period of expansion .................................................................................. 36. Nat. er. io. sit. y. Table 7 Descriptive statistics of institutional investors ........................................... 37 Table 8 Analysis for herding and feedback trading by institutional investors for a full. al. n. v i n 38 business cycle ........................................................................................... Ch engchi U. Table 9 Analysis for herding and feedback trading by institutional investors during a period of contraction ................................................................................. 39. Table 10 Analysis for herding and feedback trading by institutional investors during a period of expansion................................................................................ 40 Table 11 Descriptive statistics of all types of institutional investors ...................... 41 Table 12 Analysis for herding and feedback trading by different types of institutional investors .................................................................................................... 42 III.
(6) 1. Introduction Keynes (1936) proposes the Greater Fool Theory to suggest that investors believe they can find that others are willing to pay at a higher price to explain why some investors buy something at an unreasonably high price. As a result of asymmetric information among traders, less-informed traders mimic others’ trading behavior, and it may cause the assets to be overpriced. It leads to economic bubble. 治 政 indirectly. Less-informed traders mimic others’ trading大 behavior or pay more attention 立 ‧ 國. 學. to public information such as market returns to get information. The phenomenon is known as herding and feedback trading.1. ‧. Our study follows a significant literature by Nofsinger and Sias (1999).. sit. y. Nat. io. n. al. er. They suggest that institutional investors engage in positive feedback trading to a. i n U. v. greater extent than individual investors. Institutional investors’ herding has a larger. Ch. engchi. impact on returns than individual investors’ herding. Easley and O’Hara (1987) argue that informed traders prefer to trade larger amounts at any given price. It explains that large trades are commonly more informative. If large and small investors engage in different trading behavior, their trading-related effects cancel out. The contribution of our study is discussion of the relationship between trading behavior and trader types while considering their trade size. So far it seems, most of the previous literature 1. The earliest research of herding traces back to Asch’s conformity experiment in 1951.. 1.
(7) focuses on herding and feedback trading by trader types. There is less empirical literature on examining herding and feedback trading based on investors’ trade size. Our empirical results demonstrate individual investors are positive feedback traders, and institutional investors are negative feedback traders for the entire period. However, there are different trading patterns between large and small trade size for individual investors as well as institutional investors. Large trade size investors are. 政 治 大. the informed, and their trading behavior is independent and different from trading. 立. behavior of average investors who belong to their trader type. Small investors are. ‧ 國. 學. less-informed traders, and they mimic informed traders and pay more attention to. ‧. public information such as market returns to get information. Small individual. Nat. io. sit. y. investors would follow market returns. Small institutional investors have the most. er. significant herding behavior among all types of investors because of competition. n. al. i n C among them and reception of the similar h e ninformation. gchi U. v. The entire sample period can be divided into a period of contraction and a period of expansion. We find that investors react asymmetrically to periods of contraction and expansion. Small investors would intensify their herding and feedback trading during a period of expansion. Finally, we divide institutional investors into three types: domestic institutions, futures proprietary firms, foreign institutions. We discuss whether these three types of 2.
(8) institutional investors engage in herding and feedback trading. We observe that only foreign institutional investors engage in herding. They tend to follow themselves rather than different types of institutional investors. Moreover, we observe that only foreign institutional investors engage in negative feedback trading. Other types of institutional investors are neither positive feedback traders nor negative feedback traders.. 政 治 大. The paper is organized in four sections. Section 2 illustrates relevant literature. 立. about herding and feedback trading, and builds research hypotheses we concern in. ‧ 國. 學. this paper. Section 3 describes the data from TAIFEX and empirical methodology.. ‧. Section 4 reports the empirical results. Section 5 presents conclusions.. n. er. io. sit. y. Nat. al. Ch. engchi. 3. i n U. v.
(9) 2. Literature Review and Hypotheses 2.1 Herding and Feedback Trading Fama (1970) proposes Efficient Market Hypothesis. It assumes getting information is free and all investors are rational, and then the price can immediately and fully reflect all related information. However, there are asymmetric information problems in reality financial markets, and not all investors are rational. Less-informed. 政 治 大. investors may mimic informed investors, and rely on public information such as. 立. herding and feedback trading exist in the financial markets.. 學. ‧ 國. security returns and trading volumes. Therefore, common behavior biases such as. ‧. Herding theory originates in social psychology. Asch (1951) carried out a. y. Nat. al. er. io. sit. famous experiment (conformity experiment), which concluded that people change. n. their original thinking and decision-making because of social pressure from a great. Ch. engchi. i n U. v. majority. In the field of finance, herding is a group of investors engaged in trading toward the same direction for a period of time (Nofsinger and Sias, 1999). Feedback trading can be divided into positive feedback trading (momentum strategy) and negative feedback trading (contrarian strategy). Positive feedback trading represents investors buying past winners and sell past losers. Negative feedback trading represents investors buying past losers and sell past winners. Herding and feedback trading occur in individual and institutional investors, 4.
(10) Nofsinger and Sias (1999) suggest that individual investors have herding behavior owing to irrational, but systematic responses to fads or sentiment, while institutional investors have herding owing to agency problems, fads, security characteristics. Nevertheless, positive feedback trading is not totally irrational. De Long, Shleifer, Summers and Waldmann (1990), Kim and Wei (2002) suggest that positive feedback trading may arise from extrapolative expectations about prices (decision-making. 政 治 大. based on projections of past price and price changes), stop-loss orders (automatically. 立. selling when price declines a certain point), investors’ investment position forced to. ‧ 國. 學. liquidations (when investors are incapable of meeting their margin calls), and. ‧. portfolio insurance (buying more securities on price increasing to add exposure to risk. Nat. io. sit. y. and selling on price declining to curtail exposure to risk). De Long et al. (1990). er. purpose that rational and informed speculators push up the market prices, and other. al. n. v i n C h strategies. Finally, investors will follow positive feedback e n g c h i U they drive prices away from fundamentals, and then informed speculators sell out and stabilize market prices. Herding and feedback trading may either stabilize or destabilize stock prices. Lakonishok, Shleifer and Vishny (1992) suggest that herding and positive feedback trading by institutional investors probably destabilizes stock prices, hence it increase long-run price volatility. Nevertheless, they also argue for the opinion that precisely opposes the previous one, and suggest that herding does not necessarily destabilize 5.
(11) stock prices. Herding can accelerate the adjustment of prices to fundamentals and result in more efficient markets. Shiller (1987), Shleifer and Summers (1990) suggest that positive feedback trading makes it easier to interpret the crash of 1987. Krugman (1997) and Bange (2000) describe that herding by foreign investors destabilizes market prices because they usually trade as a vast group. Due to their size, foreign investors can easily create disarray and panic in the markets when they exit.. 政 治 大. On the other hand, they can also overheat the markets. Choe, Kho and Stulz (1999). 立. suggest that investors engage in herding and feedback trading will push prices away. ‧ 國. 學. from the fundamentals and bring about excess volatility in the markets.. ‧. n. er. io. sit. y. Nat. al. Ch. engchi. 6. i n U. v.
(12) 2.2 Herding and Feedback Trading by Individual Investors Kaniel, Saar and Titman (2008), Barber and Odean (2008), Li, Rhee and Wang (2009) propose that compared to the institutional investors, individual investors are less-informed, so they rely more on public information while making investment decisions. They pay more attention to market prices; as a result, they are more vulnerable by the influence of behavior bias, market sentiment and attention-grabbing events.. 立. 政 治 大. Which strategy do individual investors tend to engage in? Many scholars have. ‧ 國. 學. different opinions upon whether individual investors tend to decide with positive. ‧. feedback or with negative feedback.. Nat. io. sit. y. Shefrin and Statman (1985) purpose disposition effect, and find that small. er. investors are reluctant to realize their losses, and they sell winners too early and hold. al. n. v i n losers for a prolonged period ofCtime. U consistent with the notion of h eThese h i are n g cresults. negative feedback trading. Odean (1998) also finds that individual investors have disposition effect. Li et al. (2009) present evidence that individual investors are traders who rely more on negative feedback on the Shanghai Stock Exchange (SSE) and the Shenzhen Stock Exchange (SZSE). Nofsinger and Sias (1999) posit that individual investors are likely to be affected by fad and fashion, so they are trend-chasers. Shleifer and Summers (1990) suggest 7.
(13) that individual investors probably will herd if they follow the same signals (the advices of brokers and financial gurus). Lakonishok et al. (1994) posit that individual investors might extrapolate past growth rates of glamour stocks and mainly buy past excellent-performance stocks (glamour strategy). However, individual investors can be divided into large small groups. The thesis aims to analyze whether the two groups apply the same strategy in trading. The. 政 治 大. following is the literature review regarding the topic. Easley and O'Hara (1987) argue. 立. that informed traders would prefer to trade large amounts at any given price.. ‧ 國. 學. Barclay and Warner (1993) support the hypothesis that informed-traders concentrate. ‧. on the medium-size. Coughenour and Shastri (1999) summarize some literature and. Nat. io. sit. y. conclude that there is a positive link between certain trade sizes and informed trading.. er. Lee, Lin and Liu (1999) analyze the trading patterns of investors on the TSE, and. al. n. v i n they divide individual investorsCinto h elarge h i Ugroups. They suggest that large n gandc small. trades are more informative than small trades. Thus, individual investors who belong to a large group are better-informed than individual investors of small ones. They find that large individual investors’ trading would affect stock returns, small individual investors tend to mimic informed traders and engage in negative feedback trading. Chou, Wang, Wang and Bjursell (2011) find that there are significant positive relationships between trade size and information effects and that large individual 8.
(14) trades share the same patterns of buy and sell like institutional investors on the Taiwan Futures Exchange.. 2.3 Herding and Feedback Trading by Institutional Investors Some scholars have found that institutional investors have significant herding. The following are interpretations of why herding is prevalent among institutional investors.. 政 治 大. First, institutional investors would neglect their own noisy information, infer. 立. Lakonishok et al., 1992; Nofsinger and Sias, 1999).. 學. ‧ 國. others’ quality information and herd (Bikhchandani, Hirshleifer and Welch, 1992;. ‧. Second, institutional investors receive the same information or use the same. Nat. behavior (Froot, Scharfstein and Stein, 1992).. n. al. Third, it is difficult to. er. io. sit. y. indicators to measure the trading performance. Therefore, they have similar trading. v i n C h the performanceU of fund assess engchi. managers from the. “strength” or “luck,” which gives rise to agency problems. Many managers hold the same subject and engage in the same trading strategy with other managers so as to keep their reputation, avoid lagging behind other peers, or “share the blame” with each other. The above-mentioned reasons drive managers to herd (Scharfstein and Stein, 1990; Lakonishok et al., 1992; Nofsinger and Sias, 1999). The following section will review some literature upon various kinds of feedback 9.
(15) trading by institutional investors. Lakonishok et al. (1992) put forward three possible conditions. First, managers may be fired as a result of poor performance for few quarters so they focus on short-term investment strategies as positive feedback trading. They are reluctant to do the fundamental strategies as negative feedback trading, which need a long time for it eventually to settle up. Moreover, managers raise the proportion of. 政 治 大. winners and decrease the proportion of losers in their portfolios and such. 立. manipulation will cause the “window dressing” effect.. ‧ 國. 學. Second, in comparison with individual investors, institutional investors have. ‧. up-to-date information and therefore they can assess the fundamentals more. Nat. sit er. io. sell overvalued ones.. y. accurately. They select negative feedback strategy, purchase undervalued stocks, and. al. n. v i n C hof institutional investors Third, the trading strategies e n g c h i U are divergent. There are. large enough feedback traders from both the positive and the negative and they will offset the influence on each other. Overall, institutional investors in the markets have no specific feedback strategy. With regard to herding empirical evidence, most scholars have similar results: institutional investors have significant herding behavior. Nofsinger and Sias (1999) conclude that no matter degree of herding or positive feedback trading among 10.
(16) institutional investors is higher than individual investors on NYSE. Lee et al. (1999) detect institutional investors would herd to other institutional investors on the TSE. Li et al. (2009) find that the extent of herding among institutional investors is larger than individual investors on the SSE and the SZSE. With respect to feedback trading empirical evidence, many scholars have different results. Lee et al. (1999) detect institutional investors are neither positive. 政 治 大. feedback traders nor negative feedback traders on TSE. Griffin, Harris and Topaloglu. 立. (2003) find that institutional investors are positive feedback traders in NASDAQ-100. ‧ 國. 學. Stocks. Sias (2004) learns institutional investors are positive feedback traders, but the. ‧. significance of herding is larger than the significance of positive feedback trading. Nat. io. sit. y. among institutional investors for all NYSE, AMEX and NASDAQ Stocks.. n. al. er. Li et al. (2009) find that institutional investors are negative feedback traders on the SSE and the SZSE.. Ch. engchi. i n U. v. Some scholars research into herding of investor types performed by different institutions. It is Krugman’s conclusion (1997) that foreign institutional investors have significant herding by which foreign investors will likely to destabilize the market. Bowe and Domuta (2004) conclude that the degree of herding among foreign institutional investors is larger than domestic institutional investors on the Jakarta Stock Exchange. 11.
(17) 2.4 Herding and Feedback Trading in different periods As a result of financial globalization, liberalization, and innovation, every country’s economy is closely linked with one another. In a short span of two decades, global and regional finance crises occur more frequently. Asian financial crisis of 1997 and financial tsunami of 2008 are standard examples. Some literature explores whether investors’ trading behavior would change before and after the crisis.. 政 治 大. The research of Choe et al. (1999) indicates that herding drops off during the. 立. crisis for lower liquidity stocks because investors are less capable of trading on. ‧ 國. 學. Korean Stock Market. They also detect that individual and foreign investors engage in. ‧. herding but with different patterns: individual investors are negative feedback traders,. Nat. io. sit. y. but foreign investors are positive feedback traders at the individual stock level before. er. the crisis. During the crisis, individual investors are still negative feedback traders but. al. n. v i n Ctrading the evidence of positive feedback investors becomes much weaker. h e nofgforeign chi U. Kurov (2008), Chau, Deesomsak and Lau (2011) document that the intensity of positive feedback trading increases in the periods of bullish because security markets are affected by emotion more intensely in the periods. Slam and Schuppli (2010) analyze the index futures markets of 32 developing and developed countries. They discover that the phenomenon of positive feedback trading seems to be the strongest during the period of financial tsunami in relation to the falling futures prices. The two 12.
(18) scholars infer that investors with long positions are difficult to meet margin calls when futures prices decline. Investors have no choice but sell futures contract, which causes futures prices to further go down.. 立. 政 治 大. ‧. ‧ 國. 學. n. er. io. sit. y. Nat. al. Ch. engchi. 13. i n U. v.
(19) 2.5 Hypothesis Hypothesis 1: Individual and institutional investors would engage in herding and feedback trading. Compared to the institutional investors, individual investors are less-informed, so they rely more on public information such as market prices while making investment decisions. Because of competition among institutional investors and reception of. 政 治 大. similar information, institutional investors have significant herding behavior.. 立. Hypothesis 2: Individual and institutional investors in small trade size are more. ‧ 國. 學. likely to engage in herding and feedback trading than large investors.. ‧. Small investors are less-informed traders in comparison with large investors.. Nat. io. sit. y. They mimic others’ trading behavior or pay more attention to public information such. n. al. er. as market returns to get information. Therefore, they are more likely to engage in herding and feedback trading.. Ch. engchi. i n U. v. Hypothesis 3: Investors intensify herding and feedback trading behavior during a period of expansion. The emotion of investors is stronger during a period of expansion, the intensity of herding and feedback trading is higher during a period of expansion than during a period of contraction.. 14.
(20) 3. Data and Methodology 3.1 Data Description The data consists of complete daily trading data for all market traders in the market of Taiwan Capitalization weighted stock index futures (TAIEX Futures) for 991 days, from April 2004 to March 2008. The sample period includes a full business cycle referring to Council for Economic Planning and Development (CEPD). A full. 政 治 大. business cycle contains precisely one period of expansion and one period of. 立. contraction. In practice, a period of contraction and a period of expansion should be. ‧ 國. 學. continued for at least 5 months, and a full business cycle should be continued for at. ‧. least 15 months. Our sample period for 11 months, from April 2004 to February 2005,. y. Nat. al. n. March 2008, is the 12th expansion defined by CEPD.. Ch. engchi. er. io. sit. is the 11th contraction defined by CEPD, and for 37 months, from March 2005 to. i n U. v. TAIFEX is operated under an automatic auction system, starting from 8:45am to 1:45 pm, Monday to Fridays (public holidays excluded). The price limits on TAIFEX is ±7% of the previous days’ closing price. Futures contracts in TAIFEX are traded in an electronic limited order market without market makers. The tick value of TAIEX Futures is NT$200. Delivery Months include the spot month, the next calendar month and next three quarterly months. The last trading day is the third Wednesday for each month. All contracts are exercised on expiration date automatically and settled for 15.
(21) cash. We select only nearby contracts in this study for that they are most liquid. Table 1 shows descriptive statistics per contract by trade type categories. Our data enables us to categorize four types of investors: individual investors, domestic institutions, future proprietary firms and foreign institutions. We can find that individual investors are the largest groups of investors, and their trades account for approximately 73% of the total trading volume in TAIEX Futures. The trades of. 政 治 大. domestic institutional investors, proprietary firm investors and foreign institutional. 立. investors account for approximately 1%, 19% and 7% of the total trading volume in. ‧ 國. 學. TAIEX Futures, respectively. On the other hand, the numbers of individual investors. ‧. and institutional investors varies drastically. There are 157,397 individual investors. Nat. io. sit. y. and 826 institutional investors transacted in TAIEX Futures during the sample period.. er. We also divide individual investors and institutional investors into groups of. al. n. v i n C h We rank investors large and small traders, respectively. e n g c h i U according to their trading volume during the entire sample period. We sum up the total trading volume from small size investors to large size investors orderly, and use total trading volume distribution to distinguish large traders and small traders. Investors’ cumulative distribution of total trading volume from 0 to 50 percent (approximately) is classified as small investors; others are classified as large investors.. 16.
(22) 3.2 Methodology We use Dratio to measure whether investors engage in herding and feedback trading. Dratio is proposed by Lakonishok et al. (1992).They use this measure excess demand of stock markets over a period of time. For a given type investors, 𝑖, Dratio defines it as: Dratio(𝑖, 𝑡) =[ Buy(𝑖, 𝑡) −Sell(𝑖, 𝑡) ] / [ Buy(𝑖, 𝑡) +Sell(𝑖, 𝑡) ]. (1). 政 治 大. Where Buy(𝑖) increases in futures position by all 𝑖 type of investors in day 𝑡.. 立. Sell(𝑖) decreases in futures position by all 𝑖 type of investors in day 𝑡. The range of. ‧ 國. 學. Dratio(𝑖, 𝑡) is between -1 to 1. For example, certain type of investors only buys in. ‧. one day, we know the value of Sell(𝑖, 𝑡) is 0, so subsequently, Dratio(𝑖, 𝑡) is 1. In. Nat. io. sit. y. short, Dratio is the net buy orders by all certain type of investors in one day.. er. We use VAR model with reduced form equations to analyze the daily data. al. n. v i n C hFutures return. In U changes in Dratio(𝑖, 𝑡) and TAIEX e n g c h i using VAR model to analyze the. data, we have to confirm that the time series data are stationary. We use Dickey-Fuller test and Augmented Dickey-Fuller test to examine whether the time series data are stationary. As a consequence, we can reject the null hypothesis of a unit root at the 1% significance level.2 It concludes that the time series data are stationary. We can use VAR model to examine the time series data.. 2. We don’t report the significant results here for brevity, but they are available upon request.. 17.
(23) The VAR model is described as follows: 𝑅𝑡 = 𝛼0 +𝛽1 𝑅𝑡−1 +𝛼2 𝐼𝑁𝐷𝑡−1 +𝛼3 𝐼𝑁𝑆𝑡−1 + 𝜀1𝑡. ((2). 𝐼𝑁𝐷𝑡 = 𝛽0 +𝛽1 𝑅𝑡−1 +𝛽2 𝐼𝑁𝐷𝑡−1 +𝛽3 𝐼𝑁𝑆𝑡−1 + 𝜀2𝑡. ((3). 𝐼𝑁𝑆𝑡 = 𝛾0 +𝛾1 𝑅𝑡−1 +𝛾2 𝐼𝑁𝐷𝑡−1 +𝛾3 𝐼𝑁𝑆𝑡−1 + 𝜀3𝑡. (4). 𝑅𝑡 denotes TAIEX Futures return in day 𝑡.( TAIEX 𝑡 − TAIEX 𝑡−1 ) / TAIEX 𝑡−1. 𝑅𝑡−1 is one lag of TAIEX Futures return in day 𝑡. 𝐼𝑁𝐷𝑡 is the net buy orders by. 政 治 大. individual investors in day 𝑡. 𝐼𝑁𝐷𝑡−1 is one lag of the net buy orders by individual. 立. investors in day 𝑡. 𝐼𝑁𝑆𝑡 is the net buy orders by institutional investors in day 𝑡.. ‧ 國. 學. 𝐼𝑁𝑆𝑡−1 is one lag of the net buy orders by institutional investors in day 𝑡.. ‧. The VAR model in Eq. (2) examines whether each type of investors can predict. Nat. io. sit. y. TAIEX Futures return. To make readers understand the model clearly, following is an. er. example of the net buy orders by individual investors: If 𝑅𝑡 and 𝐼𝑁𝐷𝑡−1 have. al. n. v i n significant positive relationship,Cthe U that individual investors are h ephenomenon n g c h ireads. capable of predicting TAIEX Futures return. The VAR model in Eq. (3) and (4) examines whether each type of investors engages in herding and feedback trading. If the relationship between 𝐼𝑁𝐷𝑡 and 𝑅𝑡−1 is positive and significant, it shows individual investors engage in positive feedback trading. When index rose last period, they buy in current period; when index fell last period, they sell in current period. If the relationship between 𝐼𝑁𝐷𝑡 and 𝐼𝑁𝐷𝑡−1 is positive and significant, it shows 18.
(24) individual investors engage in herding. They follow their trading. If the relationship between 𝐼𝑁𝐷𝑡 and 𝐼𝑁𝑆𝑡−1 is positive and significant, it shows individual investors mimic institutional investors’ trading behavior. Moreover, we divide individual investors and institutional investors into groups of large and small traders, respectively. Then we use VAR model to examine the effect of investors’ trade size on their trading behavior.. 政 治 大. The VAR model is described as follow:. 立. 𝑅𝑡 = 𝛼0 +𝛽1 𝑅𝑡−1 +𝛼2 𝐿𝐼𝑁𝐷𝑡−1 +𝛼3 𝑆𝐼𝑁𝐷𝑡−1 +𝛼4 𝐿𝐼𝑁𝑆𝑡−1 +𝛼5 𝑆𝐼𝑁𝑆𝑡−1 + 𝜀1𝑡. (5). ‧ 國. 學. 𝐿𝐼𝑁𝐷𝑡 = 𝛽0 +𝛽1 𝑅𝑡−1 +𝛽2 𝐿𝐼𝑁𝐷𝑡−1 +𝛽3 𝑆𝐼𝑁𝐷𝑡−1 +𝛽4 𝐿𝐼𝑁𝑆𝑡−1 +𝛽5 𝑆𝐼𝑁𝑆𝑡−1 + 𝜀2𝑡 (6). ‧. 𝑆𝐼𝑁𝐷𝑡 = 𝛾0 +𝛾1 𝑅𝑡−1 +𝛾2 𝐿𝐼𝑁𝐷𝑡−1 +𝛾3 𝑆𝐼𝑁𝐷𝑡−1 +𝛾4 𝐿𝐼𝑁𝑆𝑡−1 +𝛾5 𝑆𝐼𝑁𝑆𝑡−1 + 𝜀3𝑡 ((7). Nat. io. sit. y. 𝐿𝐼𝑁𝑆𝑡 = 𝛿0 +𝛿1 𝑅𝑡−1 +𝛿2 𝐿𝐼𝑁𝐷𝑡−1 +𝛿3 𝑆𝐼𝑁𝐷𝑡−1 +𝛿4 𝐿𝐼𝑁𝑆𝑡−1 +𝛿5 𝑆𝐼𝑁𝑆𝑡−1 + 𝜀4𝑡 ((8). er. 𝑆𝐼𝑁𝑆𝑡 = 𝜌0 +𝜌1 𝑅𝑡−1 +𝜌2 𝐿𝐼𝑁𝐷𝑡−1 +𝜌3 𝑆𝐼𝑁𝐷𝑡−1 +𝜌4 𝐿𝐼𝑁𝑆𝑡−1 + 𝜌5 𝑆𝐼𝑁𝑆𝑡−1 + 𝜀5𝑡 (9). al. n. v i n is the net buy orders byC large h eindividual i U in day 𝑡. 𝑆𝐼𝑁𝐷𝑡 n g c hinvestors. 𝐿𝐼𝑁𝐷𝑡. is the net. buy orders by small individual investors in day 𝑡. 𝐿𝐼𝑁𝑆𝑡 is the net buy orders by large institutional investors in day 𝑡 . 𝑆𝐼𝑁𝑆𝑡 is the net buy orders by small institutional investors in day 𝑡. Because institutional investors are categorized into three types of investors: domestic institutional investors, proprietary firm investors, foreign institutional investors. We use VAR model to examine whether each type of institutional investors 19.
(25) engage in similar herding and feedback trading behavior. The VAR model is described as follow: 𝑅𝑡 = 𝛼0 +𝛽1 𝑅𝑡−1 +𝛼2 𝐷𝑂𝑀𝐸𝑡−1 +𝛼3 𝑃𝑅𝑂𝑃𝑡−1 +𝛼4 𝐹𝑂𝑅𝐸𝑡−1 + 𝜀1𝑡. (10). 𝐷𝑂𝑀𝐸𝑡 = 𝛽0 +𝛽1 𝑅𝑡−1 +𝛽2 𝐷𝑂𝑀𝐸𝑡−1 +𝛽3 𝑃𝑅𝑂𝑃𝑡−1 +𝛽4 𝐹𝑂𝑅𝐸𝑡−1 + 𝜀2𝑡. ((11). 𝑃𝑅𝑂𝑃𝑡 = 𝛾0 +𝛾1 𝑅𝑡−1 +𝛾2 𝐷𝑂𝑀𝐸𝑡−1 +𝛾3 𝑃𝑅𝑂𝑃𝑡−1 +𝛾4 𝐹𝑂𝑅𝐸𝑡−1 + 𝜀3𝑡. ((12). 𝐹𝑂𝑅𝐸𝑡 = 𝛿0 +𝛿1 𝑅𝑡−1 +𝛿2 𝐷𝑂𝑀𝐸𝑡−1 +𝛿3 𝑃𝑅𝑂𝑃𝑡−1 +𝛿4 𝐹𝑂𝑅𝐸𝑡−1 + 𝜀4𝑡. (13). 政 治 大. 𝐷𝑂𝑀𝐸𝑡 is the net buy orders by domestic institutional investors in day 𝑡. 𝑃𝑅𝑂𝑃𝑡 is. 立. the net buy orders by proprietary firm investors in day 𝑡. 𝐹𝑂𝑅𝐸𝑡 is the net buy orders. ‧ 國. 學. by foreign institutional investors in day 𝑡.. ‧. n. er. io. sit. y. Nat. al. Ch. engchi. 20. i n U. v.
(26) 4. Empirical Results 4.1 Herding and feedback trading by individual investors and the effect of trade sizes Table 4 shows herding and feedback trading by individual investors during the entire sample period. In Panel A, we observe a significant positive correlation between TAIEX Futures return in last period and the net buy orders by individual. 政 治 大. investors. It demonstrates that individual investors are significant positive feedback. 立. traders. They are less-informed traders, so they pay more attention to public. ‧ 國. 學. information such as market prices. They buy TAIEX futures when market price rises. ‧. and sell it when market price falls. On the other hand, we observe individual investors. y. Nat. al. er. io. sit. tend to transact with lags and the opposing direction to institutional investors.. n. To examine the effect of trade sizes on herding and feedback trading behavior by. Ch. engchi. i n U. v. individual investors, we divide individual investors into large and small groups. Table 2 shows descriptive statistics per contract by trade size categories. We define two trade sizes of individual investors each as follows: individual investors whose total trading volumes are above 3,864 contracts belong to the large group, individual investors whose total trading volumes are below and equal to 3,864 contracts belong to the small group. We can see that the average trade sizes of large individual investors and small individual investors vary drastically. The average trade sizes of 21.
(27) large individual investors are over one hundred times more than the average trade sizes of small individual investors By looking at Panel B of Table 4, we find that only lag of the net buy orders by large individual investors can explain TAIEX futures return in current period. The statistics result is slightly significant. It implies that large individual investors are informed traders and they have capability of predicting market returns. Besides, we. 政 治 大. observe that large individual investors are neither positive feedback nor negative. 立. transact with lags to small individual investors.. 學. ‧ 國. feedback traders. It is interesting that we find that large individual investors tend to. ‧. According to Panel C, we observe that small individual investors are significant. Nat. io. sit. y. positive feedback traders. On the other hand, we observe small individual investors. er. tend to transact with lags and the opposing direction to large individual investors and. al. n. v i n C hresult is consistentUwith the result of Lee et al. small institutional investors. The engchi (1999). In short, individual investors are significant positive feedback traders but engage in herding not significantly. We find that large individual investors are informed traders and their trading behavior is independent and different from average individual investors’ trading behavior. Small individual investors are less-informed traders, and they tend to engage in positive feedback trading to get more attention. 22.
(28) 4.2 Herding and feedback trading by institutional investors and the effect of trade sizes Table 8 shows herding and feedback trading by institutional investors during the entire sample period. In Panel A, we observe a significant negative correlation between TAIEX Futures return in last period and the net buy orders by institutional investors. It demonstrates that institutional investors are significant negative feedback. 政 治 大. traders. What is more, we find that they engage in herding to themselves. Because of. 立. institutional investors have significant herding behavior.. 學. ‧ 國. the competition among institutional investors and reception of similar information,. ‧. To examine the effect of trade sizes on herding and feedback trading behavior by. Nat. io. sit. y. institutional investors, we also divide institutional investors into large and small. er. groups. Table 2 shows institutional investors whose total trading volumes are above. al. n. v i n 577,850 contracts belong to theClarge investors whose total U h e group, h iinstitutional n g cand trading volumes are below and equal to 577,850 contracts belong to the small group. We can see that the average trade sizes of large individual investors and small. individual investors vary widely. The numbers of large institutional investors account for 1.5% of the numbers of all institutional investors. However, the total trading volumes of large institutional investors account for more than half of the total trading volumes of all institutional investors. 23.
(29) By looking at Panel B of Table 8, we observe that large institutional investors are positive feedback traders. There is slight evidence to indicate that large institutional investors follow their lag trading, but they would not follow other types of investors. Meanwhile, Panel C shows that small institutional investors are significant negative feedback traders. In addition, we find that they engage in herding not only to themselves but also to large individual investors.. 政 治 大. In brief, institutional investors have significant herding and negative feedback. 立. trading behavior. Large institutional investors’ trading behavior is not consistent with. ‧ 國. 學. average institutional investors’ trading behavior. Small institutional investors have the. ‧. most significant herding behavior among all types of investors because of competition. Nat. n. al. er. io. sit. y. among them and reception of the similar information.. Ch. engchi. 24. i n U. v.
(30) 4.3 Herding and feedback trading in different periods We divide the entire sample period into a period of contraction and a period of expansion and examine whether each type of investors change their trading behavior in different phases of the business cycle. Table 3 and Table 7 present descriptive statistics for all dependent variables of VAR models. We can see that average return during the entire sample period is 0.03%, average return during a period of. 政 治 大. contraction is -0.02%, and average return during a period of expansion is 0.05%.. 立. Table 5 and Table 6 separately present individual investors’ trading behavior. ‧ 國. 學. during a period of contraction and a period of expansion. By looking at Panel A to. ‧. Panel C of Table 5, we find that herding almost disappears among individual. Nat. io. sit. y. investors during a period of contraction. Panel A to Panel C of Table 6 discover that. er. herding prevails over individual investors during a period of expansion. Large. n. al. individual investors have. v i n noCcapability h e n gofc hpredicting i U market. returns during a. recession. Small individual investors are positive feedback traders both during periods of contraction and expansion. However, the evidence of positive feedback trading is much weaker during a period of contraction. Table 9 and Table 10 present institutional investors’ trading behavior during periods of contraction and expansion, respectively. According to Panel A to Panel C of Table 9 and Table 10, we observe herding by institutional investors is more 25.
(31) significant during a period of expansion than a period of contraction except for large institutional investors. Large institutional investors engage in herding and positive feedback trading during a period of contraction but the phenomenon is slightly significant. Small institutional investors herd to themselves and large individual investors significantly during a period of expansion. Nevertheless, small institutional investors do not follow themselves anymore and the evidence of herding to large. 政 治 大. individual investors is weaker during a period of contraction. Besides, we observe. 立. that small institutional investors are significant negative feedback traders both during. ‧ 國. 學. periods of contraction and expansion.. ‧. In sum, investors react asymmetrically to periods of contraction and expansion.. Nat. io. sit. y. Herding and feedback trading prevail more over almost all types of investors during a. er. period of expansion except for large institutional investors. Chau et al. (2011) suggest. al. n. v i n that feedback trading is largelyCcaused h e nbygthec presence h i U of sentiment-driven trading.. Because the emotion of investors is stronger during a period of expansion than during a period of contraction, the intensity of herding and positive feedback trading increase during a period of expansion. Choe et al. (1999) suggest that investors are less capable of trading as a result of market liquidity shortfall during a period of contraction. Therefore, the phenomenon of herding is less significant during a period of contraction than during a period of expansion. 26.
(32) 4.4 Herding and feedback trading by different institutional investors In prior to the examination, we find that institutional investors have significant herding and positive feedback trading behaviors. However, institutional investors can be categorized into three types of investors: domestic institutional investors, proprietary firm investors, foreign institutional investors. In this part, we discuss whether these three types of institutional investors all engage in herding and negative feedback trading.. 立. 政 治 大. Table 11 presents descriptive statistics for all dependent variables of VAR models. ‧ 國. 學. with these three types of institutional investors. We can discover that the range of. ‧. FOREt is -0.99 to 1.00, and kurtosis is negative. It implies that foreign institutional. Nat. sit er. io. extremely the same.. y. investors are better-informed traders, and that the direction of trading by them is. al. n. v i n C hfeedback trading byUdifferent types of institutional Table 12 reports herding and engchi. investors during the entire sample period. In Panel A, we observe that no lag of the net buy orders by all types of institutional investors has significant evidence to explain TAIEX futures return in current period. In Panel B, we discover that domestic institutional investors tend to transact with lags and the opposing direction to themselves. On the other hand, domestic institutional investors are neither positive feedback nor negative feedback traders. 27.
(33) In Panel C, we find that proprietary firm investors do not engage in herding and feedback trading. In Panel D, we observe that foreign institutional investors have significant herding and negative feedback trading behavior. They buy when market prices falls and sell following increase in market prices. The intensity of herding among foreign institutional investors is larger than other types of institutional investors. The result is consistent with the evidence of Bowe and Domuta (2004).. 政 治 大. In a word, only foreign institutional investors engage in herding. They are more. 立. likely to follow the same type of institutional investors than different types of. ‧ 國. 學. institutional investors. The result accords with the result of Sias (2004). Moreover, we. ‧. observe that only foreign institutional engage in negative feedback trading among all. Nat. n. al. er. io. sit. y. types of institutional investors.. Ch. engchi. 28. i n U. v.
(34) 5. Conclusions In this study, we use a unique futures dataset from April 2004 to March 2008 to examine whether each type of investors engages in herding and feedback trading. We have obtained several interesting results. First, there are different trading patterns between individual investors and institutional investors. Individual investors are positive feedback traders. They follow. 政 治 大. market returns to get information. Institutional investors engage in herding because of. 立. competition among them, and they are negative feedback traders.. ‧ 國. 學. On the other hand, we examine the effect of investors’ trade size. We find that. ‧. large investors’ trading behavior is independent and different from trading behavior of. y. Nat. al. er. io. sit. average investors who belong to their trader type. This may result from the fact that. n. large investors are the informed. However, Small investors’ trading behavior is. Ch. engchi. i n U. v. consistent with trading behavior of average investors who belong to their trader type. Compared to large investors, they engage in herding and feedback trading more. We think that they are less-informed traders, and they mimic informed traders and pay more attention to public information such as market returns to get information. Furthermore, we divide the entire sample period into a period of contraction and a period of expansion. We find that investors react asymmetrically to periods of contraction and expansion. Herding and feedback trading prevail more among small 29.
(35) investors during a period of expansion than a period of contraction. Finally, we find that only foreign institutional investors engage in herding. They are more likely to follow the same type of institutional investors than different types of institutional investors. The result accords with the result of Sias (2004). Besides, only foreign institutional investors are significant negative feedback traders among all types of institutional investors. Other types of institutional investors are neither. 政 治 大. positive feedback nor negative feedback traders.. 立. ‧. ‧ 國. 學. n. er. io. sit. y. Nat. al. Ch. engchi. 30. i n U. v.
(36) Table 1 Descriptive statistics per contract by trade type categories The table presents descriptive statistics for four types of Taiwan Stock Exchange Capitalization Weighted Stock Index (TAIEX) futures investors: (1) Individual investors; (2) Domestic institutional investors; (3) Proprietary firm investors; (4) Foreign institutional investors. The sample period is for 991 days, from April 2004 to March 2008.The. 政 治 大. sample period includes a full business cycle referring to Council for Economic Planning and Development (CEPD). A full business cycle contains a period of contraction and a period of expansion.. ‧ 國. Individual Investors Investors. 315,771. Sell. 34,560,103. 25,381,306. 319,098. Total. 69,049,168. 50,594,457. 634,869. 2,392,448. 6,421,414. 2,438,285. 19.04%. 6.94%. 18.58%. 7.06%. 630. 67. 129. 321.44. 1,007.73. 193,867.30. 374.48. 51,053.94. 640.63. 13,107.07. 4,874.60. 100%. 73.10%. Sell. 100%. 73.44%. Number of traders 157,397. al. 0.92%. n. Buy. Ch. 0.92%. engchi. sit. 4,830,733. io. 12,989,109. Percentage of total trading volume. 158,223. Investors. 6,567,695. y. 25,213,151. ‧. 34,489,065. Nat. Buy. Total. Foreign Institutional. Proprietary Firm Investors. er. Total trading volume. Domestic Institutional. 學. All Investors. 立. i n U. v. Average trading volume per person Mean. 436.4. Daily trading volume (991 days) Mean. 69,676.25. 31.
(37) Table 2 Descriptive statistics per contract by trade size categories The table presents descriptive statistics for large and small size investors of Taiwan Stock Exchange Capitalization Weighted Stock Index (TAIEX) futures investors: (1) Large individual investors; (2) Small individual investors; (3) Large institutional investors; (4) Small institutional investors. The sample period is for 991 days, from April. 政 治 大. 2004 to March 2008. The sample period includes a full business cycle containing a period of contraction and a period of expansion defined by Council for Economic Planning and Development.. ≥3,864. <3,864. ≥577,850. 25,295,361. 9,650,271. a l155,913 i12v n Ch engchi U. n. Total. 1,484. Average trading volume per person Mean. 17,048. <577,850. y. sit. io. Number of traders. Small Institutional Investors. 8,804,440. er. 25,299,096. ‧. Large Institutional Investors. Total trading volume Total. 學. Small Individual Investors. Nat. Large Individual Investors Criteria to distinguish Size. ‧ 國. 立. 162. 804,189. 32. 814. 10,816.
(38) Table 3 Descriptive statistics of individual investors The table presents descriptive statistics for all dependent variables of VAR model for individual investors. The sample period includes a full business cycle for 991 days, from April 2004 to March 2008, a period of contraction for 226 days, from April 2004 to February 2005, a period of expansion for 765 days, from March 2005 to March 2008.. 政 治 大. Business cycle is defined by Council for Economic Planning and Development. 𝑅𝑡 is TAIEX Futures return in day 𝑡. 𝐼𝑁𝐷𝑡 is the net buy orders by all individual investors. 𝐿𝐼𝑁𝐷𝑡 is the net buy orders by large individual investors. 𝑆𝐼𝑁𝐷𝑡 is the net buy orders by small individual investors. Min.. Max.. 學. Panel A: Full business cycle. 立. S.D.. ‧ 國. Mean. Skewness. Kurtosis. 0.0140. -0.0699. 0.0616. -0.5668. 3.5496. 𝐼𝑁𝐷𝑡. -0.0051. 0.0466. -0.2404. 0.1625. -0.0660. 1.7593. 𝐿𝐼𝑁𝐷𝑡. -0.0015. 0.0458. -0.1844. 0.1923. -0.0575. 1.7442. 𝑆𝐼𝑁𝐷𝑡. -0.0086. 0.0803. -0.3915. 0.2715. -0.2130. 1.5257. -0.0699. 0.0613. -0.3637. 3.4476. -0.2404. 0.1625. -0.2262. 4.2288. -0.5251. 0.9601. -0.0150. 1.4731. 𝐼𝑁𝐷𝑡. -0.0047. 0.0459. 𝐿𝐼𝑁𝐷𝑡. -0.0013. 0.0462. 𝑆𝐼𝑁𝐷𝑡. -0.0091. 0.0776. Panel C:Period of expansion. y. al. sit. 0.0155. n. -0.0002. io. 𝑅𝑡. er. Panel B: Period of contraction. ‧. 0.0003. Nat. 𝑅𝑡. iv 0.1132 n C-0.1676 h e n g c h i U0.2291 -0.3261. 𝑅𝑡. 0.0005. 0.0135. -0.0699. 0.0616. -0.6448. 3.4964. 𝐼𝑁𝐷𝑡. -0.0052. 0.0468. -0.1982. 0.1528. -0.0214. 1.1126. 𝐿𝐼𝑁𝐷𝑡. -0.0016. 0.0457. -0.1844. 0.1924. 0.0830. 2.0029. 𝑆𝐼𝑁𝐷𝑡. -0.0084. 0.0811. -0.3902. 0.2715. -0.2645. 1.5480. 33.
(39) Table 4 Analysis for herding and feedback trading by individual investors for a full business cycle The table analyzes herding and feedback trading by individual investors from April 2004 to March 2008. The sample period includes a full business cycle referring to Council for Economic Planning and Development. 𝑅𝑡 is TAIEX Futures return in day 𝑡. 𝐼𝑁𝐷𝑡 is the net buy orders by all individual investors. 𝐿𝐼𝑁𝐷𝑡 is the net buy orders by large. 政 治 大. individual investors. 𝑆𝐼𝑁𝐷𝑡 is the net buy orders by small individual investors. *Significant at the 10%. **Significant at the 5%. ***Significant at the 1%.. 𝐼𝑁𝐷𝑡−1. 𝐼𝑁𝑆𝑡−1. 學. Panel A: individual investors. 立. 𝑅𝑡−1. ‧ 國. Intercept. -0.0256. 0.0059. 0.0042. S.D.. 0.0004. 0.0352. 0.0176. 0.0070. 𝐼𝑁𝐷𝑡. -0.0044***. 0.2678**. -0.0027. -0.0603***. S.D.. 0.0015. 0.1171. 0.0586. 0.0231. Intercept. 𝑅𝑡−1. 𝐿𝐼𝑁𝐷𝑡−1. 𝑆𝐼𝑁𝐷𝑡−1. 0.0202*. -0.0019. y. sit. er. io. Panel B: large individual investors. ‧. 0.0003. Nat. 𝑅𝑡. 𝐿𝐼𝑁𝑆𝑡−1. 𝑆𝐼𝑁𝑆𝑡−1. 0.0003. 0.0029. 0.0038. 0.0037. -0.0084. 0.0047. 𝑅𝑡. 0.0003. -0.0342. S.D.. 0.0004. 0.0358. 𝐿𝐼𝑁𝐷𝑡. -0.0009. -0.1582. S.D.. 0.0014. 0.1178. 0.0356. 0.0318. 0.0126. 0.0123. n. al. iv 0.0097 n C0.0108 h e n g c h i U0.0795** -0.0870**. Panel C: small individual investors 𝑆𝐼𝑁𝐷𝑡. -0.0077***. 0.9345***. -0.2432***. -0.0011. -0.0284. -0.0939***. S.D.. 0.0024. 0.2010. 0.0608. 0.0542. 0.0215. 0.0209. 34.
(40) Table 5 Analysis for herding and feedback trading by individual investors during a period of contraction The table analyzes herding and feedback trading by individual investors. Our sample period for 11 months, from April 2004 to February 2005 is 11 th contraction defined by Council for Economic Planning and Development. 𝑅𝑡 is TAIEX Futures return in day 𝑡. 𝐼𝑁𝐷𝑡 is the net buy orders by all individual investors. 𝐿𝐼𝑁𝐷𝑡 is the net buy orders. 政 治 大. by large individual investors. 𝑆𝐼𝑁𝐷𝑡 is the net buy orders by small individual investors. *Significant at the 10%. **Significant at the 5%. ***Significant at the 1%.. 𝐼𝑁𝐷𝑡−1. 𝐼𝑁𝑆𝑡−1. 學. -0.0239. -0.0526. -0.0085. S.D.. 0.0010. 0.0697. 0.0515. 0.0188. 𝐼𝑁𝐷𝑡. -0.0033. 0.0380. 0.2619*. 0.0133. S.D.. 0.0029. 0.1979. 0.1462. 0.0534. Intercept. 𝑅𝑡−1. 𝐿𝐼𝑁𝐷𝑡−1. 𝑆𝐼𝑁𝐷𝑡−1. 0.0128. -0.0316. sit. er. io. Panel B: large individual investors. ‧. -0.0003. Nat. 𝑅𝑡. y. Panel A: individual investors. 立. 𝑅𝑡−1. ‧ 國. Intercept. 𝐿𝐼𝑁𝑆𝑡−1. 𝑆𝐼𝑁𝑆𝑡−1. -0.0034. -0.0023. 0.0090. 0.0098. -0.0320. 0.0026. 𝑅𝑡. -0.0003. -0.0477. S.D.. 0.0010. 0.0718. 𝐿𝐼𝑁𝐷𝑡. 0.0000. -0.2598. S.D.. 0.0030. 0.2112. 0.0792. 0.0837. 0.0265. 0.0289. n. al. iv 0.0285 n C0.0269 h e n g c h i U0.1220 -0.1137. Panel C: small individual investors 𝑆𝐼𝑁𝐷𝑡. -0.0071. 0.6796*. -0.2180*. 0.1186. -0.0114. -0.0529. S.D.. 0.0050. 0.3481. 0.1305. 0.1380. 0.0436. 0.0477. 35.
(41) Table 6 Analysis for herding and feedback trading by individual investors during a period of expansion The table analyzes herding and feedback trading by individual investors. Our sample period for 37 months, from March 2005 to March 2008 is 12 th expansion defined by Council for Economic Planning and Development. 𝑅𝑡 is TAIEX Futures return in day 𝑡. 𝐼𝑁𝐷𝑡 is the net buy orders by all individual investors. 𝐿𝐼𝑁𝐷𝑡 is the net buy orders. 政 治 大. by large individual investors. 𝑆𝐼𝑁𝐷𝑡 is the net buy orders by small individual investors. *Significant at the 10%. **Significant at the 5%. ***Significant at the 1%.. 𝐼𝑁𝐷𝑡−1. 𝐼𝑁𝑆𝑡−1. 學. Panel A: individual investors. 立. 𝑅𝑡−1. ‧ 國. Intercept. -0.0185. 0.0170. 0.0055. S.D.. 0.0005. 0.0415. 0.0184. 0.0074. 𝐼𝑁𝐷𝑡. -0.0047***. 0.3385**. -0.0381. -0.0688***. S.D.. 0.0017. 0.1456. 0.0646. 0.0258. Intercept. 𝑅𝑡−1. 𝐿𝐼𝑁𝐷𝑡−1. 𝑆𝐼𝑁𝐷𝑡−1. 0.0201*. 0.0032. y. sit. er. io. Panel B: large individual investors. ‧. 0.0005. Nat. 𝑅𝑡. 𝐿𝐼𝑁𝑆𝑡−1. 𝑆𝐼𝑁𝑆𝑡−1. 0.0007. 0.0030. 0.0042. 0.0041. 0.0004. 0.0093. 𝑅𝑡. 0.0005. -0.0234. S.D.. 0.0005. 0.0425. 𝐿𝐼𝑁𝐷𝑡. -0.0011. -0.1259. S.D.. 0.0016. 0.1461. 0.0410. 0.0356. 0.0145. 0.0140. n. al. iv 0.0104 n C0.0119 h e n g c h i U0.0795** -0.0683*. Panel C: small individual investors 𝑆𝐼𝑁𝐷𝑡. -0.0077***. 1.0631***. -0.2364***. -0.0116. -0.0349. -0.0997***. S.D.. 0.0028. 0.2504. 0.0702. 0.0609. 0.0249. 0.0240. 36.
(42) Table 7 Descriptive statistics of institutional investors The table presents descriptive statistics for dependent variables of VAR model for institutional investors. The sample period includes a full business cycle for 991 days, from April 2004 to March 2008, a period of contraction for 226 days, from April 2004 to February 2005, a period of expansion for 765 days, from March 2005 to March 2008.. 政 治 大. Business cycle is defined by Council for Economic Planning and Development. 𝑅𝑡 is TAIEX Futures return in day 𝑡. 𝐼𝑁𝑆𝑡 is the net buy orders by all institutional investors. 𝐿𝐼𝑁𝑆𝑡 is the net buy orders by large institutional investors. 𝑆𝐼𝑁𝑆𝑡 is the net buy orders by small institutional investors. Min.. Max.. 學. Panel A: Full business cycle. 立. S.D.. ‧ 國. Mean. Skewness. Kurtosis. 0.0140. -0.0699. 0.0616. -0.5668. 3.5496. 𝐼𝑁𝑆𝑡. 0.0107. 0.1163. -0.5449. 0.3998. -0.1141. 0.5995. 𝐿𝐼𝑁𝑆𝑡. 0.0097. 0.1518. -0.5374. 0.6045. 0.0614. 1.1782. 𝑆𝐼𝑁𝑆𝑡. 0.0120. 0.2134. -0.6289. 0.6304. -0.1160. -0.1158. -0.0699. 0.0613. -0.3637. 3.4476. -0.5449. 0.2831. 1.2758. 4.2288. -0.1423. 0.2936. -0.3018. -0.3506. 𝐼𝑁𝑆𝑡. 0.0111. 0.1250. 𝐿𝐼𝑁𝑆𝑡. 0.0014. 0.1523. 𝑆𝐼𝑁𝑆𝑡. 0.0091. 0.2384. Panel C:Period of expansion. y. al. sit. 0.0155. n. -0.0002. io. 𝑅𝑡. er. Panel B: Period of contraction. ‧. 0.0003. Nat. 𝑅𝑡. iv 0.4190 n C-0.4622 h e n g c h i U0.4528 -0.6289. 𝑅𝑡. 0.0005. 0.0135. -0.0699. 0.0616. -0.6448. 3.4964. 𝐼𝑁𝑆𝑡. 0.0105. 0.1137. -0.3564. 0.3998. 0.0878. 0.2999. 𝐿𝐼𝑁𝑆𝑡. 0.0085. 0.1518. -0.5374. 0.6045. 0.1217. 1.4625. 𝑆𝐼𝑁𝑆𝑡. 0.0129. 0.2056. -0.6165. 0.6304. -0.0263. -0.0655. 37.
(43) Table 8 Analysis for herding and feedback trading by institutional investors for a full business cycle The table analyzes herding and feedback trading by institutional investors from April 2004 to March 2008. The sample period includes a full business cycle referring to Council for Economic Planning and Development. 𝑅𝑡 is TAIEX Futures return in day 𝑡. 𝐼𝑁𝑆𝑡 is the net buy orders by all institutional investors. 𝐿𝐼𝑁𝑆𝑡 is the net buy. 政 治 大. orders by large institutional investors. 𝑆𝐼𝑁𝑆𝑡 is the net buy orders by small institutional investors.*Significant at the 10%. **Significant at the 5%. ***Significant at the 1%.. 𝐼𝑁𝐷𝑡−1. 𝐼𝑁𝑆𝑡−1. 學. Panel A: institutional investors. 立. 𝑅𝑡−1. -0.0256. 0.0059. 0.0042. S.D.. 0.0004. 0.0352. 0.0176. 0.0070. 𝐼𝑁𝑆𝑡. 0.0094***. -0.8180***. 0.1924. 0.2249***. S.D.. 0.0036. 0.2922. 0.1461. 0.0577. Intercept. 𝑅𝑡−1. 𝐿𝐼𝑁𝐷𝑡−1. 𝑆𝐼𝑁𝐷𝑡−1. al. sit. er. io. Panel B: large institutional investors. y. 0.0003. Nat. 𝑅𝑡. ‧. ‧ 國. Intercept. iv 0.0097 n C0.0108 h e n g c h i U-0.0554 -0.1005. 𝐿𝐼𝑁𝑆𝑡−1. 𝑆𝐼𝑁𝑆𝑡−1. 0.0003. 0.0029. 0.0038. 0.0037. 0.0787*. -0.0576. 0.0003. -0.0342. S.D.. 0.0004. 0.0358. 𝐿𝐼𝑁𝑆𝑡. 0.0089*. 0.9977**. S.D.. 0.0048. 0.3929. 0.1189. 0.1060. 0.0419. 0.0409. n. 𝑅𝑡. 0.0202*. -0.0019. Panel C: small institutional investors 𝑆𝐼𝑁𝑆𝑡. 0.0100. -3.1938***. 0.9920***. 0.0966. 0.0808. 0.3445***. S.D.. 0.0064. 0.5241. 0.1586. 0.1414. 0.0559. 0.0546. 38.
(44) Table 9 Analysis for herding and feedback trading by institutional investors during a period of contraction The table analyzes herding and feedback trading by institutional investors. Our sample period for 11 months, from April 2004 to February 2005 is 11th contraction defined by Council for Economic Planning and Development. 𝑅𝑡 is TAIEX Futures return in day 𝑡. 𝐼𝑁𝑆𝑡 is the net buy orders by all institutional investors. 𝐿𝐼𝑁𝑆𝑡 is the net buy. 政 治 大. orders by large institutional investors. 𝑆𝐼𝑁𝑆𝑡 is the net buy orders by small institutional investors. *Significant at the 10%. **Significant at the 5%. ***Significant at the 1%.. 𝐼𝑁𝐷𝑡−1. 𝐼𝑁𝑆𝑡−1. 學. -0.0239. -0.0526. -0.0085. S.D.. 0.0010. 0.0697. 0.0515. 0.0188. 𝐼𝑁𝑆𝑡. 0.0091. -0.2855. -0.2308. 0.0990. S.D.. 0.0081. 0.5519. 0.4076. 0.1489. Intercept. 𝑅𝑡−1. 𝐿𝐼𝑁𝐷𝑡−1. 𝑆𝐼𝑁𝐷𝑡−1. al. sit. er. io. Panel B: large institutional investors. ‧. -0.0003. Nat. 𝑅𝑡. y. Panel A: institutional investors. 立. 𝑅𝑡−1. ‧ 國. Intercept. iv 0.0285 n C0.0269 h e n g c h i U0.1675 0.2848. 𝐿𝐼𝑁𝑆𝑡−1. 𝑆𝐼𝑁𝑆𝑡−1. -0.0034. -0.0023. 0.0090. 0.0098. 0.1456*. -0.0466. -0.0003. -0.0477. S.D.. 0.0010. 0.0718. 𝐿𝐼𝑁𝑆𝑡. 0.0153. 1.6800**. S.D.. 0.0099. 0.6969. 0.2613. 0.2763. 0.0874. 0.0955. n. 𝑅𝑡. 0.0128. -0.0316. Panel C: small institutional investors 𝑆𝐼𝑁𝑆𝑡. 0.0025. -3.2533***. 0.9254**. -0.4891. 0.0159. 0.2234. S.D.. 0.0150. 1.0520. 0.3944. 0.4170. 0.1319. 0.1441. 39.
(45) Table 10 Analysis for herding and feedback trading by institutional investors during a period of expansion The table analyzes herding and feedback trading by institutional investors. Our sample period for 37 months, from March 2005 to March 2008 is 12 th expansion defined by Council for Economic Planning and Development. 𝑅𝑡 is TAIEX Futures return in day 𝑡. 𝐼𝑁𝑆𝑡 is the net buy orders by all institutional investors. 𝐿𝐼𝑁𝑆𝑡 is the net buy orders. 政 治 大. by large institutional investors. 𝑆𝐼𝑁𝑆𝑡 is the net buy orders by small institutional investors. *Significant at the 10%. **Significant at the 5%. ***Significant at the 1%.. 𝐼𝑁𝐷𝑡−1. 𝐼𝑁𝑆𝑡−1. 學. Panel A: institutional investors. 立. 𝑅𝑡−1. -0.0185. 0.0170. 0.0055. S.D.. 0.0005. 0.0415. 0.0184. 0.0074. 𝐼𝑁𝑆𝑡. 0.0098**. -1.0128***. 0.2468. 0.2441***. S.D.. 0.0041. 0.3546. 0.1573. 0.0629. Intercept. 𝑅𝑡−1. 𝐿𝐼𝑁𝐷𝑡−1. 𝑆𝐼𝑁𝐷𝑡−1. al. sit. er. io. Panel B: large institutional investors. y. 0.0005. Nat. 𝑅𝑡. ‧. ‧ 國. Intercept. iv 0.0104 n C0.0119 h e n g c h i U-0.1168 -0.2210. 𝐿𝐼𝑁𝑆𝑡−1. 𝑆𝐼𝑁𝑆𝑡−1. 0.0007. 0.0030. 0.0042. 0.0041. 0.0590. -0.0494. 0.0005. -0.0234. S.D.. 0.0005. 0.0425. 𝐿𝐼𝑁𝑆𝑡. 0.0073. 0.5839. S.D.. 0.0055. 0.4870. 0.1365. 0.1185. 0.0483. 0.0466. n. 𝑅𝑡. 0.0201*. 0.0032. Panel C: small institutional investors 𝑆𝐼𝑁𝑆𝑡. 0.0118*. -3.1147***. 0.9562***. 0.2034. 0.0921. 0.3572***. S.D.. 0.0070. 0.6264. 0.1756. 0.1524. 0.0622. 0.0599. 40.
(46) Table 11 Descriptive statistics of all types of institutional investors The table presents descriptive statistics for all dependent variables of VAR model for all types of institutional investors. The sample period includes a full business cycle referring to Council for Economic Planning and Development. 𝑅𝑡 is TAIEX Futures return in day 𝑡. 𝐷𝑂𝑀𝐸𝑡 , 𝑃𝑅𝑂𝑃t , 𝐹𝑂𝑅𝐸𝑡 are the net buy orders by domestic. 政 治 大. institutional investors, proprietary firm investors, foreign institutional investors in day 𝑡, respectively.. Min.. Max.. Skewness. Kurtosis. 0.0003. 0.0140. -0.0699. 0.0616. -0.5668. 3.5496. 𝐷𝑂𝑀𝐸𝑡. -0.0121. 0.2727. -0.8398. 0.8356. 0.0484. 0.1497. 𝑃𝑅𝑂𝑃𝑡. 0.0126. 0.1270. 0.5290. -0.4529. 0.1303. 1.0683. 𝐹𝑂𝑅𝐸𝑡. 0.0183. 0.4085. 1.0000. -0.9907. 0.0342. -0.3764. io. sit. y. ‧. Nat. n. al. er. 𝑅𝑡. 立. ‧ 國. S.D.. 學. Mean. Ch. engchi. 41. i n U. v.
(47) Table 12 Analysis for herding and feedback trading by different types of institutional investors The table analyzes herding and feedback trading by different types of institutional investors from April 2004 to March 2008. The sample period includes a full business cycle referring to Council for Economic Planning and Development. 𝑅𝑡 is TAIEX Futures return in day 𝑡. 𝐷𝑂𝑀𝐸𝑡 , 𝑃𝑅𝑂𝑃t , 𝐹𝑂𝑅𝐸𝑡 are the net buy orders by domestic institutional. 政 治 大. investors, proprietary firm investors, foreign institutional investors in day 𝑡, respectively. *Significant at the 10%. **Significant at the 5%. ***Significant at the 1%.. Panel B: domestic institutional investors -0.0123. S.D.. 0.0086. -0.9809. -0.1146***. 0.7105. 0.0322. io. Panel C: proprietary firm investors. 0.0016. Nat. 𝐷𝑂𝑀𝐸𝑡. 0.0360. 0.0123***. 0.3183. S.D.. 0.0040. 0.3293. al. -0.0070. n. 𝑃𝑅𝑂𝑃𝑡. Panel D: foreign institutional investors. Ch. n engchi U 0.0149. 𝐹𝑂𝑅𝐸𝑡−1. -0.0013. 0.0008. 0.0041. 0.0012. -0.0540. -0.0112. y. 0.0004. 0.0001. sit. S.D.. -0.0179. er. 0.0003. 𝑃𝑅𝑂𝑃𝑡−1. ‧. 𝑅𝑡. 𝐷𝑂𝑀𝐸𝑡−1. 學. Panel A: returns. 立. 𝑅𝑡−1. ‧ 國. Intercept. iv. 0.0800. 0.0228. 0.0510. -0.0151. 0.0371. 0.0106. 𝐹𝑂𝑅𝐸𝑡. 0.0129. -3.3876***. 0.0515. 0.0423. 0.3164***. S.D.. 0.0124. 1.0210. 0.0463. 0.1150. 0.0328. 42.
(48) References Asch, S. 1956. Studies of independence and conformity: A minority of one against a unanimous majority. Psychological Monographs 70 (9). Bange, M.M., 2000. Do the portfolios of small investors reflect positive feedback trading? Journal of Financial and Quantitative Analysis 35, 239–255. Barber, B.M., Odean, T., 2008. All that glitters: The effect of attention and news on the buying behavior of individual and institutional investors. Review of Finance Studies 21 (2), 785-818. Barclay, M.J., Warner, J.B., 1993. Stealth trading and volatility: Which trades move prices? 1111Journal of Financial Economics 34, 281-305. Bikhchandani, S., Hirshleifer, D., Welch, I., 1992. A theory of Fads, Fashion, Custom, and. 政 治 大 Bowe M., Domuta D., 2004. Investor herding during financial crisis: A clinical study of the 立 1111Jakarta Stock Exchange. Pacific-Basin Finance Journal 12, 387– 418. 1111Cultural Change as Informational Cascades. Journal of Political Economy 100, 992-1026.. ‧ 國. 學. Chau, F., Deesomsak, R., Lau, M.C.K., 2011. Investor sentiment and feedback trading: Evidence from the exchange-traded fund markets. International Review of Financial. ‧. Analysis 20, 292–305.. Choe, H., Kho, B.C., Stulz, R.M., 1999. Do foreign investors destabilize stock markets? The. y. Nat. Korean experience in 1997. Journal of Financial Economics 54, 227–264.. sit. Chou, R.K., Wang, G.H.K., Wang, Y.Y., Bjursell, J., 2011. The impacts of large trades by. al. n. Pacific-Basin Finance Journal 19, 41–70.. Ch. er. io. trader types on intraday futures prices: Evidence from the Taiwan Futures Exchange.. i n U. v. Coughenour, J., Shastri, K., 1999. Symposium on market microstructure: A Review of. engchi. Empirical Research. Financial Review 34, 1-28.. De Long, J.B., Shleifer, A., Summers, L.H., Waldmann, R.J., 1990. Positive feedback investment strategies and destabilizing rational speculation. Journal of Finance 45, 379– 395. Easley, D., O'Hara, M., 1987. Price, trade size, and information in securities markets. Journal of Financial Economics 19, 69-90. Fama, E.F., 1970. Efficient Capital Markets: A Review of Theory and Empirical Work. Journal of Finance 25, 383-417. Froot, K.A., Scharfstein, D.S., Stein, J.C., 1992. Herd on the street: Inefficiencies in a market with short-term speculation. Journal of Finance 47, 1461-1484. Griffin, J., Harris, J.H., Topaloglu, S., 2003. The Dynamics of Institutional and Individual Trading. Journal of Finance 58, 2285-2320.. 43.
(49) Kaniel, R., Saar, G., Titman, S., 2008. Individual Investor Trading and Stock Returns. Journal of Finance 63, 273-310. Keynes, J.M., 1936. The General Theory of Employment, Interest, and Money. (Macmillan, London, U.K.). Kim, W., Wei, S.J., 2002. Foreign portfolio investors before and during a crisis. Journal of International Economics 56, 77–96. Krugman, P., 1997. Seven habits of highly defective investors. Fortune, December 27 Kurov, A., 2008. Investor Sentiment, Trading Behavior and Informational Efficiency in Index Futures Markets. Financial Review 43, 107-127. Lakonishok, J., Shleifer, A., Vishny, R., 1992. The impact of institutional trading on stock prices. Journal of Financial Economics 32, 23–43. Lakonishok, J., Shleifer, A., Vishny, R., 1994. Contrarian Investment, Extrapolation, and Risk. Journal of Financial Economics 49, 1541-1578.. 政 治 大 emerging market: An empirical analysis. Journal of Banking & Finance 23, 701-725. 立 Li, W., Rhee, G., Wang, S.S., 2009. Differences in Herding: Individual vs. Institutional. Lee, Y.T., Lin, J.C., Liu, Y.J., 1999. Trading patterns of big versus small players in an. ‧ 國. 學. Investors in China. Working Paper, School of Accounting and Finance, The Hong Kong Polytechnic University, Kowloon, Hong Kong, China.. ‧. Nofsinger, J.R., Sias R.W., 1999. Herding and Feedback Trading by Institutional and Individual Investors. Journal of Finance 54, 2263-2295.. y. sit. 1775-1798.. Nat. Odean,T., 1998. Are investors reluctant to realize their loses? Journal of Finance 53,. al. n. Review 80, 465–479.. er. io. Scharfstein, D.S., Stein, J.C., 1990. Herd behavior and investment. American Economic. Ch. i n U. v. Shefrin, H., Statman, M., 1985. The disposition to sell winners too early and ride losers too. engchi. long: theory and evidence. Journal of Finance 40, 777-790.. Shiller, R.J., 1988. Portfolio Insurance and Other Investor Fashions as Factors in the 1987 Stock Market Crash. NBER Macroeconomics 3, 287-297. Shleifer, A., Summers, L.H., 1990. The Noise Trader Approach to Finance. Journal of Economic Perspectives 4, 19-33. Sias, R.W., 2004. Institutional Herding. Review of Financial Studies 17, 165-206. Slam, C.A., Schuppli, M., 2010. Positive feedback trading in stock index futures: International evidence. International Review of Financial Analysis 19, 313–322.. 44.
(50)
數據
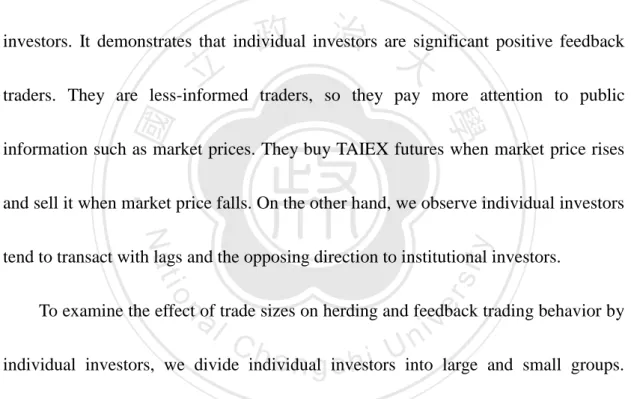
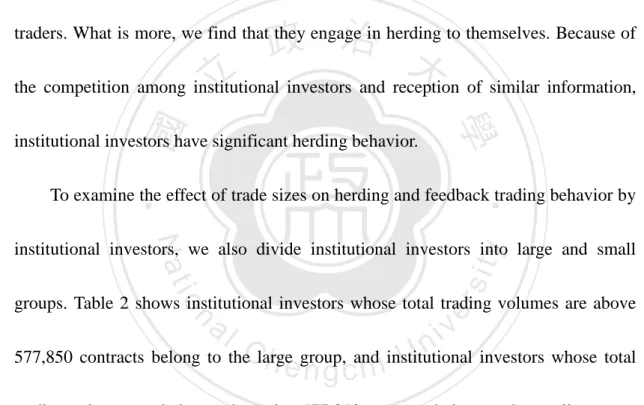
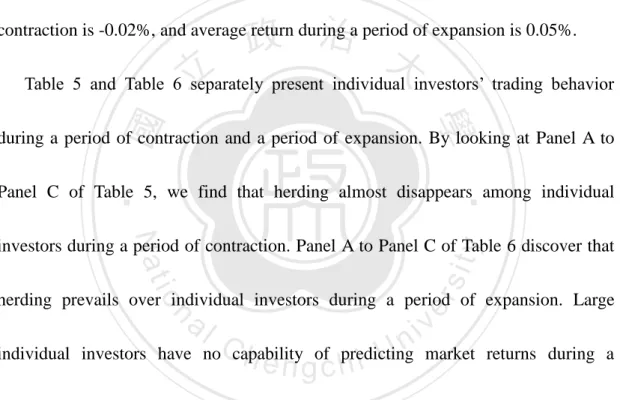
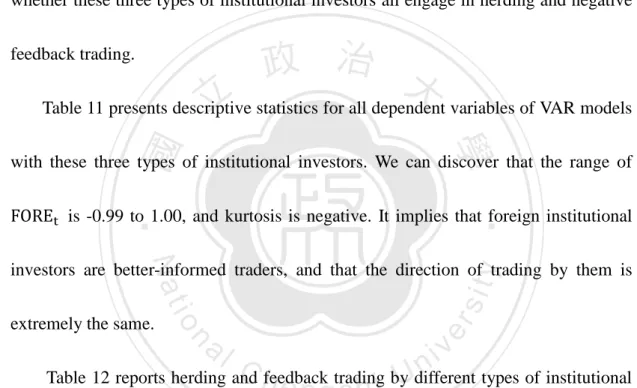
Outline
相關文件
“xilu”still seems vague and caught in“the mythical background.”The present paper looks for traces of the character“lu”in works of the relevant period and demonstrates by way
為加入歐盟,土國長期以來執行與歐盟經貿市場調和政 策,歐盟亦成為土國最大外資來源、最大外銷市場。土 歐於
6 Gregory Schopen, “The Good Monk and His Money in a Buddhist Monasticism of ‘the Mahāyāna Period’,” in Buddhist Monks and Business Matters: Still More Papers on
paper, we will examine the formation of the early Buddhist scribal workshop in Liangzhou during the Northern Liang period based on the archaeological discovery of
Piecewise polynomial interpolation: divide the interval into a collection of subintervals and construct different approximation on each subinterval. The simplest piecewise
Wang, Solving pseudomonotone variational inequalities and pseudocon- vex optimization problems using the projection neural network, IEEE Transactions on Neural Networks 17
Define instead the imaginary.. potential, magnetic field, lattice…) Dirac-BdG Hamiltonian:. with small, and matrix
During the period of Jin Dynasty and Northern and Southern Dynasties, minorities migrated into Central Plain and established different regimes in north China. With the