MARVS Revisited: Incorporating Sense Distribution and Mutual Information into Near-Synonym Analyses
全文
(2) Siaw-Fong Chung and Kathleen Ahrens. depend on intuition with the aid of additional references such as dictionaries. Quantitative analyses, on the other hand, usually attempt to find out the differences between nearsynonyms through comparing the behaviors of the synonymous pairs such as by comparing the argument types of the pairs attested. Earlier work on synonyms tended to focus on providing descriptive information, such as that demonstrated by Collinson (1939, cited in Harris 1973:14), who attempted to list the possible differences between synonyms using nine elements: general/specific applicability, intensity, emotion, moral approbation, professionalism, written/non-written, colloquialism, local/dialect, and child talk. Today, the commonly agreed differences between synonyms are found within features such as connotations, implications, selectional restrictions, and syntactic variations (i.e. Cruse 1986, Lyons 1995, DiMarco, Hirst & Stede 1993, Edmonds 1999). Cruse, for example, considered selectional and collocational restrictions the “main effect of presupposed semantic traits of a lexical item,” which brings out the “syntagmatic companions” of words (1986:278-279). Near-synonyms, according to Cruse, are “lexical items whose senses are identical in respect of ‘central’ semantic traits, but differ… in ‘minor’ or ‘peripheral traits’” (1986:278-279). In fact, most synonyms are near-synonyms which share certain central similarities and peripheral differences. Perfect synonyms are considered rare (Taylor 2002:265). Later work on distinguishing near-synonyms used both descriptive as well as quantitative analyses. For instance, Taylor’s (2002) analysis of ‘tall’ and ‘high’ was carried out through psycholinguistic experimentation (acceptability rating tasks) in addition to descriptive analyses of the two adjectives. Taylor claimed that ‘tall’ and ‘high’ can be differentiated using MacLaury’s (1997, 2002) Vantage theory, which distinguish near-synonyms in terms of dominant/recessive meanings. For both these adjectives, the dominant meaning emphasizes the similarity of “a fixed landmark which is the human body sanctions the application of the word to a limited range of prominently upright entities”. However, ‘tall’ has restrictions on dimensional uses whereas ‘high’ has restrictions on positional uses. More statistical approaches to near-synonyms can be seen in the computational field. For example, a statistical analysis of near-synonyms by Church et al. (1994) use Mutual Information (MI), as well as substitutability in terms of T-scores to differentiate between the near-synonyms ‘request’ and ‘ask for’. MI values measure the degree of co-occurrences between terms, so as to determine whether a word is a collocate to another word. They found twenty-eight significant objects that collocate with both ‘request’ and ‘ask for’, among which are ‘aid’, ‘assistance’, ‘copy’, ‘dismissal’, and ‘extension’. In addition, Church et al. (1994) showed that near-synonyms can be compared in terms of their substituted words. For example, they found that ‘request’ has a higher substitutability value than ‘ask for’ when substituted by words such as ‘seek’,. 416.
(3) MARVS Revisited. ‘grant’, and ‘demand’. Therefore, substitution is one way to test the similarities and differences of near-synonyms, i.e. through comparing whether or not the same words can substitute for the arguments of the two compared near-synonyms. Also important in computational approaches to near-synonyms is the model suggested by Pustejovsky (1991). In this model, the meanings of the verbs can be generated from the nominals surrounding the verbs by examining the Qualia structures of the verbs, i.e. the structure of the nominals are co-compositional by four types of roles, shown in (1) below (Pustejovsky 1991:426-427). (1) a.. Constitutive role (the relation between an object and its proper parts such as ‘narrative’ for a novel) b. Formal role (role that distinguishes the object within a larger domain such as ‘book’ or ‘disk’ for a novel) c. Telic role (the purpose and function of the object such as ‘read’ for a novel) d. Agentive role (factors involved in bring about the object such as ‘artifact’ or ‘write’ for a novel). Based on these four roles, the author claims that the load of distinguishing verb meanings can be distributed to the nominals (or adjectives) surrounding the verbs. This model has later been used in various linguistic theories. The strength of this model comes from its prediction of the possibilities to distinguish a term from another based on their ‘part-of-relation’ (constitutive role), ‘kind-of-relation’ (formal role), ‘function relation’ (telic role), and ‘origin relation’ (agentive role). (These four relations are also stated in Croft & Cruse 2004:137.) When near-synonyms are concerned, the peripheral differences between a synonymous pair can occur at any of these four aspects. In other words, these four aspects provide alternative ways of stating the differences between a synonymous set, in addition to stating the differences in semantic features as was done in traditional semantics (i.e. [±female], [±animate], etc.) or that in the work of Collinson (1939). The MARVS model (Huang et al. 2000) shares several assumptions with recent work on lexical semantics. For example, the first assumption of MARVS is that lexical semantic information can be used to predict grammatical behavior (cf. Dowty 1991, Levin 1993, Goldberg 1995). An additional assumption is that lexical semantics is grammatically based and mediates conceptual structures (cf. Bresnan & Kanerva 1989, Zaenen 1993, Pustejovsky 1991). Thus, the MARVS model proposes that an adequate theory of verbal semantics must be able to represent directly semantic information in a way that can be connected to grammatical structures, such as through event structure. In. 417.
(4) Siaw-Fong Chung and Kathleen Ahrens. addition, the lexical semantic information must have conceptual motivation (i.e. similar to the qualia structure as suggested by Pustejovsky 1991). Lastly, all lexical semantic attributes must be data-based, using information such as collocating structure, selectional constraints, or distributional patterns (Huang et al. 2000). Gathering these linguistic assumptions, MARVS turns out to be predictive when comparing words with almost synonymous meanings. This paper proposes that the distributional information of sense frequency and collocational-based information can further refine the steps needed to run a lexical semantic analysis of near-synonym verb pairs.. 2. Module-Attribute Representation of Verbal Semantics MARVS lays out eventive information in terms of event modules and role modules. (See Figure 1 below taken from Huang et al. 2000:24; arrows added.). Figure 1: Eventive information in MARVS Under each module (see arrows), there are attributes which further define the behaviors of the module. Some examples of role-internal attributes are [sentience], [volition], [affectedness], and [design]. Examples of event-internal attributes are [control] and [effect]. In Huang et al. (2000), two verbs of ‘put’ in Chinese (擺 b3i and 放 f4ng) are found to differ at the [design] of the role-internal attribute because the way of putting is different for the two verbs, with 擺 b3i, but not 放 f4ng, entailing the act of “putting. 418.
(5) MARVS Revisited. following a certain plan” as well “a resultant state”.1 The following diagram shows the differences between 擺 b3i and 放 f4ng (Huang et al. 2000:36). (2) MARVS for bai3 and fang4 bai3 •____ <Agent, Theme, Location> [design] fang4. •____. <Agent, Theme, Location>. The methodology of MARVS is corpus-based, and contrasts the differentiation between the near-synonyms in terms of attributes. Therefore, it has the combination of both the descriptive approach and the corpus-based approach. This work proposes two additional steps to MARVS in order to further clarify what is needed for an accurate verbal semantic analysis. The first step includes analyzing instances from corpus so as to establish the similarities and differences between near-synonyms. The second step suggests using Mutual Information (MI) values to look for argument types that collocate with the verbs and suggests criteria for the selection of collocates based on these values, as the usual results from the calculation of MI values contain noise which has to be filtered out manually. This paper will address these issues in detail through the use of examples of two verbs of ‘put’ (擺 b3i and 放 f4ng) following Huang et al. (2000), and Ahrens, Huang & Chuang (2003).. 3. Proposals for improving the MARVS analysis Scholars such as Tognini-Bonelli (2001) distinguish between corpus-based and corpus-driven analyses. Corpus-based analysis uses corpus as resources of examples for verifying intuition. Corpus-driven analysis, on the other hand, allows the discovery of new sentence patterns for the purpose of research. MARVS is a model that is corpus-based because it, to date, has been based on selective use of sentences from a large corpus (cf. Huang et al. 2000 and Ahrens, Huang & Chuang 2003). Ahrens, Huang & Chuang (2003), for example, suggested that the different meanings of the English ‘set’ and the Chinese 擺 b3i can be represented in MARVS. However, they only took a selection of example sentences from the corpus. The original steps of 1. Chinese terms of 擺 b3i and 放 f4ng are added in this paper. Huang et al. (2000) use bai3 and fang4 instead. Pinyin in this paper is generated based on the Pintone software (Teng, Cheng & Lin 2006).. 419.
(6) Siaw-Fong Chung and Kathleen Ahrens. MARVS are re-stated by Ahrens, Huang & Chuang (2003:470; underline and bold added). How do we determine these collateral differences? First, we examine these near-synonym pairs by first combing a corpus for all relevant examples of the words in question. These examples are then categorized according to their syntactic function. Third, each instance is classified into its argumentstructure type. Fourth, the aspectual type associated with each verb is determined. And fifth, the sentential type for each verb is also determined. The underlined step above shows that the study did not collect and analyze a set of random sentences from the corpus, but only extracted relevant examples needed. However, if all the meanings of all sentences in a random set of two near-synonyms are analyzed, one can obtain information such as (a) the meaning shared by the pairs (i.e. the ‘central semantic traits’) and (b) the differences in meanings between the pairs (i.e. the ‘peripheral traits’) (cf. Cruse 1986:278-279). Recent advances in corpora tools also allow for advances to be made in semantic analysis. For example, one can now obtain information about collocations in terms of MI values. This information can be found in the Academia Sinica Balanced Corpus of Modern Chinese (Sinica Corpus) for the analysis of the Chinese synonyms, as well as in the British National Corpus for the analysis of ‘set’ in English. 2 By adding this information, one can reduce manual work in generating the argument types for the synonyms (as was bolded in the quotation of methodology by Ahrens, Huang & Chuang (2003) above). Finally, one important issue that remains in MARVS is the arbitrariness of the attributes. However, since this is also a problem for traditional semantics as well as in most feature-identifying models, this issue will not be addressed in this paper. Instead, we shall focus on adding two additional steps to the original methodology for lexical semantic analysis in MARVS. To do so, this paper re-analyzes 擺 b3i and 放 f4ng so as to demonstrate how the additional steps support the original analysis.. 4. Reanalysis of 擺 b3i ‘put’ and 放 f4ng ‘put’ The revised steps for a near-synonym analysis in MARVS, with inclusion of two additional steps (the second and fourth steps) and the modification of the first step, are given in (3) below. The modified step and the two additional steps are in italic bold face. 2. Sinica Corpus is available at http://www.sinica.edu.tw/ftms-bin/kiwi1/mkiwi.sh while the British National Corpus is available at http://www.natcorp.ox.ac.uk/.. 420.
(7) MARVS Revisited. (3) Near synonym analysis in MARVS First, examine the near-synonym pairs by analyzing at least the first 100 examples from the corpus. Second, analyze the senses either according to intuition or the meanings in WordNet so that the similarities (i.e. the pair is nearly synonymous) and differences of sense can be identified. Third, categorize the examples according to their syntactic function. Fourth, classify its argument-structure type based on their collocation restrictions discovered through MI values. Fifth, determine the aspectual type associated with each verb. Sixth, determine the sentential type for each verb. First, we suggest that a random sample of sentences be collected from the corpus. The number of examples should be consistent for all the synonymous set attested. In the second step, we suggest that these examples are then analyzed either manually or by using a reference such as WordNet (http://wordnet.princeton.edu/). The purpose of this is to find out the similarities and differences in meanings between the synonymous set. This step is also important in that it proves that the items in the synonymous set are indeed synonyms, i.e. they share at least one similarity in meaning despite other differences. The fourth step suggests that collocations and MI values can be used as criteria to determine the arguments of the synonyms. As Palmer (2000) said, consistent concrete criteria have to be stated clearly for discovering sense distinction. The aim of adding our proposal is to make the MARVS model more operationalized and thus more easily applied to other verb pairs. In the next section, we shall take 擺 b3i and 放 f4ng as an example and demonstrate how these two additional steps can be conducted.3. 4.1 Sense distribution analyses First, to prove that 擺 b3i and 放 f4ng were synonymous in meaning, sentences containing these two verbs were extracted from the Sinica Corpus and analyzed. Since 擺 b3i and 放 f4ng are Chinese words, they should be searched as Chinese words in order to obtain all their senses. SinicaBow (Huang, Chang & Lee 2004) is one of the tools that provides a Chinese-English search interface for senses.4 For instance, the 3. 4. Similar steps can be applied to improve the analysis of the English ‘tall’ and ‘high’ which was carried out in Taylor (2002). SinicaBow or the Academia Sinica Bilingual Ontological WordNet is available at http:// bow.sinica.edu.tw/.. 421.
(8) Siaw-Fong Chung and Kathleen Ahrens. senses of 擺 b3i and 放 f4ng are given in (4) below when searched in SinicaBow (based on WordNet 1.7.1). (4) Senses from WordNet 1.7.1 obtained when searched in SinicaBow a. 擺 b3i ‘put’: 1: Arrange thoughts, ideas, temporal events, etc. 2: An apparatus consisting of an object mounted so that it swings freely under the influence of gravity b. 放 f4ng ‘put’: 1: Discharge or direct or be discharged or directed as if in a continuous stream 2: Put into a certain place: “Put your things here” 3: Locate However, since SinicaBow is a translated database from the English WordNet, there are some senses that could have been excluded if these senses are not found in English. For example, in examples (5) and (6) below, the use of 擺 b3i in (5a) is not the same as in (5b), and both are not easily represented using the meanings in (4a). Similarly, example (6a) is a meaning extension of 放 f4ng but it is not related to the real sense of ‘locate’ or ‘put in a certain place’. If only certain examples are chosen from the corpus, additional senses of 擺 b3i and 放 f4ng may be left out. However, through the collection of random sample sentences from the corpus, this problem is necessarily addressed, as all examples (not a selected few) appearing in the sample collected have to be dealt with. (5) a. b. (6) a. b.. 擺個姿勢 b3i ge z9sh= ‘to pose’ 擺棋子 b3i q0z- ‘to lay a piece in a board game’ 放著風箏 f4ng zhe f5ngzh5ng ‘flying kite’ 放椅子 f4ng y-zi ‘to put a chair (somewhere)’. In order to collect a sample of sentences, this paper takes the first 100 sentences for each verb from the Sinica Corpus (from the total of 233 for 擺 b3i, and 1,031 for 放 f4ng).5 The results are shown in Table 1.6 The senses in Table 1 were decided upon based on the first author’s intuition, since SinicaBow has the inherent limitations mentioned above. 5. 6. For the current approach, the sense analysis was carried out based on intuition. Further improvement of this method can be carried out by identifying the senses automatically. For example, Ker et al. (柯淑津等) (2007) is one of the studies that we can refer to when dealing with this issue. However, further research is still needed in this respect. Since there are 100 sentences, the number of instances in each sense is also the percentage of each sense.. 422.
(9) MARVS Revisited. Table 1: Analysis of sense distribution for 擺 b3i and 放 f4ng 擺 b3i ‘put’ Metaphorical usages Arrange for display Lay (baby, basin, book, dishes, garden, etc.) Put (things) Set up Pose Move. Total. % 27 25 15 12 12 5 4. 放 f4ng ‘put’ % Metaphorical usages 44 Put (things) 21 Let go (animals, person, hand, prey, etc.) 14 Discharge (bomb, fire, firework, kite, etc.) 9 Keep (meat, tea leaves, things) 3 Play (record, music, etc.) 3 Non-classified 3 Add 2 Locate (building) 1 100 Total 100. From Table 1, there is an overlapped meaning of ‘put (things)’ that appears for both 擺 b3i and 放 f4ng. Examples of this sense can be seen in (7) below. (7) a.. 錢 就 擺 在 房間 某 件 東西 裡面 qi2n ji* b3i z4i f2ngji1n m#u ji4n d!ngx9 l-mi4n money just put at room some Class. thing inside ‘The money is put inside something (a container) in the room.’ b. 杜象 把 這個 作品 d*xi4ng b3 zh8ge zu$p-n Duxiang BA this art.work 放 在 一個 木箱 裡 f4ng z4i y0ge m*xi1ng lput at one-Class. wood-case inside ‘Duxiang put this piece of art inside a wooden case.’. As can be seen in (7), the use of 擺 b3i and 放 f4ng in (7) can be substituted for one another. This overlapped meaning shows that 擺 b3i and 放 f4ng are near-synonymous. Nevertheless, only through our analysis that we can see 放 f4ng is used 21% as ‘put’ while 擺 b3i has only 12% of the instances being used as ‘put’. In both 擺 b3i and 放 f4ng, the majority of their senses (27% for 擺 b3i and 44% for 放 f4ng) contain metaphorical meanings, exemplified in (8) below. For 擺 b3i, its second highest meaning of ‘arrange for display’ is 25%, which is close to the percentage of its metaphorical use. Therefore, from this small sample, we can see that 放 f4ng is used more often as metaphor than 擺 b3i. However, a more large-scale analysis is still needed to validate this observation.. 423.
(10) Siaw-Fong Chung and Kathleen Ahrens. (8) a.. 把 全民 利益 擺 在 第一 b3 qu2nm0n l=y= b3i z4i d=y9 BA all.nation profit put at first.place ‘To put the interests of the whole nation at priority’ b. 霸 著 話題 不 放 b4 zhe hu4t0 b/ f4ng dominate ZHE discussion.topic Neg. let.go ‘To dominate the topic of discussion (without letting go)’. The metaphorical uses found in (8) were excluded for this analysis because they will create noise in the data, if they were included as part of the other meanings.7 The “non-classified” use in 放 f4ng refers to instances where 放 f4ng is used as a noun, as in (9). There are three instances of the use in (9). (9) 「放 的 哲學」 f4ng de zh6xu6 f4ng DE philosophy ‘The philosophy of f4ng’ Lastly, the results in Table 1 also show that 擺 b3i and 放 f4ng are similar in one meaning, but they differ in many other meanings. These differing meanings give clues as to how one synonym differs from another. In the next section, we shall demonstrate the use of MI values to find the argument types for these two near-synonyms.. 4.2 Mutual Information value In order to find out the MI values for the arguments that collocate most frequently with each verb, the MI values for all the search results (233 for 擺 b3i and 1,031 for 放 f4ng) were calculated by the internal system of the Sinica Corpus. The window size is set from -4 to 4 (i.e. 4 words on the left or right of the key word). The MI list shown has several columns, as shown in Table 2 below.8. 7. 8. It is likely that these are the instances that were skipped over in the data collected in Huang et al. (2000). A smaller window size, such as -1 to 1, was not used because this will exclude constructions such as BA-constructions. However, the window size of -4 to 4 might also have excluded topicalized nouns which also use 擺 b3i and 放 f4ng.. 424.
(11) MARVS Revisited. Table 2: Examples of MI values for 擺 b3i ‘put’9 MI Freq (y) Freq (x, y) y: 詞 y: 詞類 (a) 10.126 1 1 缸數 Na (b) 10.126 1 1 咬鳥卦 Na (c) 9.839 4 3 扭腰 VA (d) 9.433 2 1 花椒 Na (e) 9.279 7 3 炮竹 Na (f) 2.380 11,562 5 的 T. Gloss g1ngsh* ‘number of jars’ y3oni3ogu4 ‘fortune-telling with birds’ ni&y1o ‘twist one’s waist’ hu1ji1o ‘a type of pepper’ p4ozh/ ‘firecrackers’ de ‘DE’. Freq (y) is the number of times the words on the rightmost column appear in the whole corpus (including texts other than 擺 b3i). Freq (x, y) refers to the number of times the words y co-occur with the target word (x=擺 b3i). MI values refer to the probability of the words y collocate with x (cf. Church & Hanks 1990). For Sinica Corpus, the definition of the MI value is the calculation “between a key and the characters occurring in the specified window (i.e. the left and/or right context)” in the Sinica Corpus (Huang, Ahrens & Chen 1998:157). The MI values calculated by the Sinica Corpus is as in (10) below, “where N is the size of the corpus and m is the size of the selected window” (Huang et al. 1998:157): (10) I(x,y) = Log2 P(x,y) / P(x) · P(y) f ( x , y) / m ⋅ N ≈ Log2 f ( x ) / N ⋅ f ( y) / N = Log2 f(x,y) · N / m · f(x) · f(y) Even though MI values are indices showing whether x and y are associated, this paper suggests that one should not refer arbitrarily to MI values. This is to avoid including data that we do not need.10 For instance, in (a) and (b) of Table 2, when both x and y occurs once respectively, the probability of the two co-occurring together will be absolute and the MI value will be high. In order to avoid this problem, this paper sets two criteria for choosing the collocated arguments for the verbs (x). These two criteria are: (a) the freq (x, y) must be higher than 3 (i.e. the x and y co-occur at least three times in the whole corpus of 擺 b3i); and (b) the MI value should not be lower than 5. These threshold levels were set based on our observation of our data. These criteria, however, can be changed based on individual research, depending on how much information one needs to include. For the current 9 10. Note that these are not necessarily the top collocates. They are a sample from the results. This problem has been noted by many, Kilgarriff & Tugwell (2001) in particular, suggest an alternative way of measuring collocations by using saliency.. 425.
(12) Siaw-Fong Chung and Kathleen Ahrens. research, both 擺 b3i and 放 f4ng are searched using the same criteria and therefore, are delimited by the same conditions. These two criteria can help avoid selecting a common term such as de 的 ((f) in Table 2) which occurs so often in the whole corpus that the MI value becomes very low (even though the number of times it co-occurs with 擺 b3i is more then 5). Based on these criteria, the final selected arguments for 擺 b3i are shown in Table 3 below. Table 3: Collocated arguments for 擺 b3i ‘put’ MI 9.839 9.279 8.072 8.006 7.511 7.487 6.991 6.991 6.507 5.932 5.881 5.680 5.627 5.605 5.393 5.282 5.088. Freq (y) 4 7 39 25 41 56 184 92 112 199 279 256 270 644 341 381 1080. Freq (x, y) 3 3 5 3 3 4 8 4 3 3 4 3 3 7 3 3 7. y: 詞 扭腰 炮竹 地攤 平 書架 桌 桌 桌子 姿勢 門口 中間 左 右 起 東 西 往. y: 詞類 VA Na Nc VC Na Nf Na Na Na Nc Ncd Ncd Ncd Di Ncd Ncd P. Gloss ni&y1o ‘twist one’s waist’ p4ozh/ ‘firecrackers’ d=t1n ‘stall on the ground’ p0ng ‘smoothen’ sh%ji4 ‘book shelf’ zhu! ‘table’ zhu! ‘table’ zhu!z- ‘table’ z9sh= ‘posture’ m6nk#u ‘entrance’ zh!ngji1n ‘middle’ zu# ‘left’ y$u ‘right’ q- ‘up’ d!ng ‘east’ x9 ‘west’ w3ng ‘toward’. Compared to the previous Table 2, one can see that these two criteria remove the problematic lexical items such as 缸 數 g1ngsh* ‘the number of tubs’ 咬 鳥 卦 y3oni3ogu4 ‘a type of fortune-telling card picked up by a bird’. Items such as these two have high MI values with 擺 b3i because the only time they appear in the corpus, they co-occur with 擺 b3i. With our proposal set forth above, we excluded those that do not fit these criteria. When the same criteria applied to 放 f4ng, the results in Table 4 are obtained.. 426.
(13) MARVS Revisited. Table 4: Collocated arguments for 放 f4ng ‘put’ MI 8.080 7.851 7.828 7.541 7.486 7.423 7.173 6.991 6.905 6.815 6.711 6.560 6.519 6.480 6.283 6.257 6.228 6.024 5.905 5.846 5.767 5.727 5.565 5.560 5.489 5.461 5.412 5.376 5.362 5.362 5.343 5.326 5.173 5.113 5.034 5.022. Freq (y) Freq (x, y) 7 4 11 5 9 4 9 3 19 6 27 8 13 3 26 5 17 3 31 5 55 8 24 3 25 3 52 6 116 11 184 17 78 7 41 3 77 5 49 3 53 3 92 5 238 11 87 4 70 3 96 4 126 5 183 7 106 4 106 4 135 5 577 21 96 3 102 3 184 5 484 13. y: 詞 長假 水燈 倉 成交價 在一塊 假 紅龜 四海 盆 架子 風箏 心念 武松 人質 重心 桌 口袋 書架 心思 炸彈 枕頭 桌子 火 畝 肩膀 化妝品 抓住 羊 客廳 牛奶 心力 重點 浴室 包袱 封 下. y: 詞類 Na Na Na Na VH Na Na Nc Na Na Na Na Nb Na Na Na Na Na Na Na Na Na Na Nf Na Na VC Na Nc Na Na Na Nc Na Nf VC. Gloss ch2ngji4 ‘long holiday’ shu-d5ng ‘water lantern’ c1ng ‘warehouse’ ch6ngji1oji4 ‘transaction price’ z4iy0ku4i ‘be together’ ji4 ‘holiday’ h@nggu9 ‘red tortoise’ s=h3i ‘Four Seas’ p6n ‘basin’ ji4z- ‘shelf’ f5ngzh5ng ‘kite’ x9nni4n ‘thoughts’ w&s!ng ‘Wusong (pronoun)’ r6nzh= ‘hostage’ zh$ngx9n ‘focus’ zhu! ‘table’ k#ud4i ‘pocket’ sh%ji4 ‘book shelf’ x9ns9 ‘thoughts’ zh4d4n ‘bomb’ zh7nt@u ‘pillow’ zhu!z- ‘table’ hu# ‘fire’ m& ‘acreage’ ji1nb3ng ‘shoulder’ hu4zhu1ngp-n ‘cosmetics’ zhu1zh* ‘grab’ y2ng ‘goat’ k8t9ng ‘living room’ ni/n3i ‘milk’ x9nl= ‘mental and physical strength’ zh$ngdi3n ‘emphasis’ y*sh= ‘bathroom’ b1of/ ‘burden’ f5ng ‘seal’ xi4 ‘below’. Comparing the lists in Tables 3 and 4, one sees that more argument types were found for 放 f4ng when these same two criteria are used. The comparison is made more obvious by laying out the collocated arguments for the two verbs, as shown in Table 5 below.. 427.
(14) Siaw-Fong Chung and Kathleen Ahrens. Table 5: Collocated arguments for 擺 b3i and 放 f4ng 擺 b3i ‘put’ 扭腰 ni&y1o ‘twist one’s waist’ 炮竹 p4ozh/ ‘firecrackers’ 地攤 d=t1n ‘stall on the ground’. 中間 zh!ngji1n ‘middle’. 平 p0ng ‘smooth’ 起. 書架 sh%ji4 ‘book shelf’. 東. 桌 zhu! ‘table’. 西. 桌子 zhu!z‘table’. 往. 姿勢 z9sh= ‘posture’ 門口 m6nk#u ‘entrance’. 架子 ji4z‘shelf’ 風箏 f5ngzh5ng ‘kite’ 心念 x9nni4n ‘thoughts’. 心思 x9ns9 ‘thoughts’ 炸彈 zh4d4n ‘bomb’ 枕頭 zh7nt@u ‘pillow’. 羊 y2ng ‘goat’. 客廳 k8t9ng ‘living room’ 牛奶 ni/n3i y$u ‘right’ ‘milk’ 心力 x9nl= 武松 w&s!ng 桌子 zhu!z‘mental and q- ‘up’ ‘Wusong ‘table’ physical (pronoun)’ strength’ 重點 人質 r6nzh= d!ng ‘east’ 火 hu# ‘fire’ zh$ngdi3n ‘hostage’ ‘emphasis’ 重心 zh$ngx9n 畝 m& 浴室 y*sh= x9 ‘west’ 假 ji4 ‘holiday’ ‘focus’ ‘acreage’ ‘bathroom’ 肩膀 ji1nb3ng 包袱 b1of/ 紅龜 h@nggu9 桌 zhu! ‘table’ w3ng ‘toward’ ‘shoulder’ ‘burden’ ‘red tortoise’ 化妝品 四海 s=h3i 口袋 k#ud4i hu4zhu1ngp-n 封 f5ng ‘seal’ ‘Four Sea’ ‘pocket’ ‘cosmestics’ 書架 sh%ji4 抓住 zhu1zh* 盆 p6n ‘basin’ 下 xi4 ‘down’ ‘book shelf’ ‘grab’. 左 zu# ‘left’ 右. 放 f4ng ‘put’ 長假 ch2ngji4 ‘long holiday’ 水燈 shu-d5ng ‘water lantern’ 倉 c1ng ‘warehouse’ 成交價 ch6ngji1oji4 ‘transaction price’ 在一塊 z4iy0ku4i ‘be together’. In Table 5, the arguments in italics are those that overlap for both 擺 b3i and 放 f4ng ‘put’. We can see that only 書架 sh%ji4 ‘book shelf’, 桌 zhu! ‘table’ and 桌子 zhu!z‘table’ are found overlapping for these two verbs and these arguments are also found under the overlapped sense of ‘put (things)’ in Table 1. By identifying the selectional restriction through this way one can verify the following statement by Huang et al. (2000:35) that “the orientation of the placed object [of bai3] can be specified while only location can be specified for fang4.” This is seen in Table 5 above for orientations of 中間 zh!ngji1n ‘middle’, 左 zu# ‘left’, 右 y$u ‘right’, etc. (shaded in Table 5), all of which are not found in the list for 放 f4ng ‘put’. When carried out using these steps, one can then make more data-driven proposals within the MARVS model. In addition to Sinica Corpus, there are also other resources which can be used to find information pertaining degree of collocation between words. The Chinese Sketch Engine (Kilgarriff, Huang, Rychly, Smith & Tugwell 2005) is able to provide the collocated arguments for 擺 b3i and 放 f4ng through WordSketches (such that exemplified in. 428.
(15) MARVS Revisited. Table 6).11 This function of Sketch Engine provides lists of collocates according to different grammatical relations such as ‘subject’, ‘object’, ‘modifier’, etc. Table 6 below shows examples of object arguments of 擺 b3i and 放 f4ng in the Chinese Sketch Engine. Table 6: WordSketches for the objects of 擺 b3i and 放 f4ng from the Chinese Sketch Engine Objects. 擺 b3i ‘put’ Gloss. ‘stall’ ‘open-air 流水席 li/shu-x0 banquet’ 低姿態 d9z9t4i ‘low profile’ 姿勢 z9sh= ‘posture’ 姿態 z9t4i ‘posture’ 長龍 ch2ngl@ng ‘long queue’ ‘to set up 攤販 t1nf4n business of a vendor’ 烏龍* w%l@ng ‘oolong tea’* 攤位 t1nw8i ‘stall’ 桌 zhu! ‘table’ 宴席 y4nx0 ‘banquet’ 攤 t1n. Freq Saliency. Objects. 放 f4ng ‘put’ Gloss. 257. 77.72. 鞭炮 bi1np4o. ‘firecrackers’. 363. 71.49. 49. 56.8. 水燈 shu-d5ng. ‘water lantern’. 178. 71.06. 32 26 32 20. 44.94 37.61 32.91 30.81. 天燈 ti1nd5ng 高利貸 g1ol=d4i 鴿子 g5zi 風聲 f5ngsh5ng. 224 139 123 51. 69.16 65.56 59.49 44.95. 23. 28.42. 12. 38.69. 12 21 15 9. 26.84 26.58 26.45 25.84. 26 35 805 31. 38.45 38.11 35.74 35.26. 19. 35.13. 110. 32.93. 14. 29.38. 6. 28.16. 派頭 p4it@u. ‘style’. 5. 23.1. 架子 ji4zi. ‘shelf’. 7. 20.11. 排場 p2ich3ng. ‘ostentation’. 5. 19.85. 5. 17.38. 2. 16.41. 擂台 l6it2i 小食攤 xi3osh0t1n. ‘a ring for contests in martial arts’ ‘small food stall’. ‘flying lantern’ ‘usury’ ‘dove’ ‘rumors’ ‘words said 厥辭 ju6c0 without serious thoughts’ 山雞 sh1nj9 ‘pheasant’ 炮 p4o ‘cannon’ 人 r6n ‘human’ 長線 ch2ngxi4n ‘long string’ 沖天炮 ‘towering ch!ngti1np4o cannon’ 比率 b-l} ‘percentage’ 和平鴿 ‘dove as a h6p0ngg5 symbol of peace’ 線狀菌 xi4nzhu4ngj*n. ‘string-like fungus’. Freq Saliency. In Table 6, we can see the most salient collocates for the ‘object’ arguments of 擺 b3i and 放 f4ng.12 Among these collocates, there are some which are also found in 11. 12. The Chinese Sketch Engine is available at http://wordsketch.ling.sinica.edu.tw/ while the English Sketch Engine is available at http://www.sketchengine.co.uk/. In 擺 b3i and 放 f4ng, one collocate was removed from each list. The removed collocates are 香案 xi1ng’4n ‘joss-tick case’ (in 擺香案 b3ixi1ng’4n ‘the case of placing joss stick’). 429.
(16) Siaw-Fong Chung and Kathleen Ahrens. Table 5 (in italics).13 The saliency value (fourth and eighth columns in Table 2) is more powerful than the MI value because it removes the problematic examples in Table 2 earlier with its formula (cf. Kilgarriff & Tugwell 2001). However, Sketch Engine is not sense-tagged. This means that a sense distribution analysis must be carried out based on intuition. Nevertheless, Sketch Engine allows the analysis of senses directly from the collocates of Wordsketches, instead of reading line by line in the concordance results. This is because Sketch Engine is based on a large corpus (i.e. the Gigaword Corpus with more than one billion characters) and this makes the collocates more reliable. For example, from Table 6, we claim that the top senses for the collocates with the top saliency values (攤 t1n for 擺 b3i and 鞭炮 bi1np4o for 放 f4ng) are ‘arrange for display’ and ‘discharge’ respectively (cf. Table 1 earlier). In Table 6, there are also several collocates which have closer meanings to some of the words in previous Table 5 (darker shades). For instance, 擺地攤 b3id=t1n ‘to set up a stall with goods laid on the ground’ in Table 5 is similar to 擺攤 b3it1n ‘to set up a stall’ in Table 6. In addition, 擺攤販 b3it1nf4n ‘to set up the business of a vendor’ is similar to 擺小食攤 b3ixi3osh0t1n ‘to set up a little food vendor’. Similarly, for 放 f4ng ‘put’, 放水燈 f4ngshu-d5ng ‘to discharge a water lantern’ is found in both Tables 5 and 6. Moreover, 放水燈 f4ngshu-d5ng ‘to discharge a water lantern’ is also similar to 放天燈 f4ngti1nd5ng ‘to discharge a flying lantern’. However, a better analysis of the collocates will need to divide the long saliency list into significant and non-significant collocates so as to compare which senses are more salient than the others, as was done in Chung (2007). We thus propose this for future research.. 5. Conclusion MARVS has both features of descriptive and quantitative analyses and this paper strengthens the quantitative aspect by proposing additional evidence for Huang et al. (2000) and Ahrens, Huang & Chuang (2003)’s MARVS-based analysis of 擺 b3i and 放 f4ng. We propose two additional criteria for near-synonym analyses and suggest that. 13. and 後稅 h$ushu= ‘later-tax’ (in 先放後稅 xi1nf4ngh$ushu= ‘first release (goods) then tax (someone)’), which are both wrongly parsed. In addition, there are some segmentation issues. One of them is 擺烏龍 b3iw%l@ng ‘absentminded’ where 烏龍 w%l@ng (asterisk) cannot be segmented from 擺 b3i ‘put’. Otherwise, 烏龍 w%l@ng can only be translated as ‘oolong tea’. Despite these problems, the Sketch Engine usually is able to display the most salient collocates. These are only part of the lists. More overlapped collocates may be found later in the lists.. 430.
(17) MARVS Revisited. these criteria further allow the operationalization of the steps used to identify contrasts in near-synonyms. In addition, we propose that analysis of sense distribution and MI values can be used to state the differences between two near synonymous verbs. The new steps proposed for MARVS combine a corpus-driven, quantitative approach with traditional semantics. This paper further integrates MI values (Church et al. 1994) into the MARVS analysis and also suggests clear criteria for the selection of MI values. Thus, this study not only provides clarification to a previously established lexical-semantic model, but also contributes methodology-wise to computational linguistic research.. References Ahrens, Kathleen, Chu-Ren Huang, and Yuan-hsun Chuang. 2003. Sense and meaning facets in verbal semantics: a MARVS perspective. Language and Linguistics 4.3: 469-484. Bresnan, Joan, and Jonni Kanerva. 1989. Locative inversion in Chichewa: a case study of factorization in grammar. Linguistic Inquiry 20.1:1-50. Chung, Siaw-Fong. 2007. A Corpus-driven Approach to Source Domain Determination. Taipei: National Taiwan University dissertation. Church, Kenneth W., and Patrick Hanks. 1990. Word association norms, mutual information and lexicography. Computational Linguistics 16.1:22-29. Church, Kenneth W., William Gale, Patrick Hanks, Donald Hindle, and Rosamund Moon. 1994. Lexical substitutability. Computational Approaches to the Lexicon, ed. by Beryl T. Sue Atkins & Antonio Zampolli, 153-177. Oxford & New York: Oxford University Press. Collinson, W. E. 1939. Comparative synonymics: some principles and illustrations. Transactions of the Philosophical Society 1939:54-77. Croft, William, and Alan D. Cruse. 2004. Cognitive Linguistics. Cambridge & New York: Cambridge University Press. Cruse, D. Alan. 1986. Lexical Semantics. Cambridge & New York: Cambridge University Press. DiMarco, Chrysanne, Graeme Hirst, and Mandred Stede. 1993. The semantic and stylistic differentiation of synonyms and near-synonyms. Proceedings of the AAAI Spring Symposium on Building Lexicons for Machine Translation, 114-121. Dowty, David. 1991. Thematic proto-roles and argument selection. Language 67.3: 547-619.. 431.
(18) Siaw-Fong Chung and Kathleen Ahrens. Edmonds, Philip. 1999. Semantic Representations of Near-Synonyms for Automatic Lexical Choice. Toronto: University of Toronto dissertation. Goldberg, Adele E. 1995. Constructions: A Construction Grammar Approach to Argument Structure. Chicago: University of Chicago Press. Harris, Roy. 1973. Synonymy and Linguistic Analysis. Toronto: University of Toronto Press. Huang, Chu-Ren, Ru-Yng Chang, Shiang-Bin Lee. 2004. Sinica BOW (Bilingual Ontological Wordnet): Integration of Bilingual WordNet and SUMO. Paper presented at the 4th International Conference on Language Resources and Evaluation (LREC2004). Lisbon, Portugal. Huang, Chu-Ren, Kathleen Ahrens, and Keh-Jiann Chen. 1998. A data-driven approach to the mental lexicon: two studies on Chinese corpus linguistics. Bulletin of the Institute of History and Philology 69.1:151-179. Huang, Chu-Ren, Kathleen Ahrens, Li-li Chang, Keh-Jiann Chen, Mei-chun Liu, and Mei-Chih Tsai. 2000. The module-attribute representation of verbal semantics: from semantics to argument structure. Computational Linguistics and Chinese Language Processing 5.1:19-46. Kilgarriff, Adam, and David Tugwell. 2001. WORD SKETCH: extraction and display of significant collocations for lexicography. Proceedings of the ACL Workshop on COLLOCATION: Computational Extraction, Analysis and Exploitation, 32-38. Toulouse, France. Kilgarriff, Adam, Chu-Ren Huang, Pavel Rychly, Simon Smith, and David Tugwell. 2005. Chinese Word Sketches. Paper presented at the ASIALEX 2005: Words in Asian Cultural Context. Singapore. Levin, Beth. 1993. English Verb Classes and Alternations: A Preliminary Investigation. Chicago: University of Chicago Press. Liu, Mei-chun. 2003. From collocation to event information: the case of Mandarin verbs of discussion. Language and Linguistics 4.3:563-585. Lyons, John. 1995. Linguistic Semantics: An Introduction. Cambridge & New York: Cambridge University Press. MacLaury, Robert E. 1997. Vantage theory in cognitive science: an anthropological account of categorization and similarity judgment. Proceedings of the Interdisciplinary Workshop on Similarity and Categorization, ed. by Michael Ramscar et al., 157-163. Edinburgh: University of Edinburgh. MacLaury, Robert E. 2002. Introducing vantage theory. Language Sciences 24.5-6: 493-536. Palmer, Martha. 2000. Consistent criteria for sense distinctions. Computers and the Humanities 34.1-2:217-222.. 432.
(19) MARVS Revisited. Pustejovsky, James. 1991. The generative lexicon. Computational Linguistics 17.4: 409-441. Taylor, John R. 2002. Near synonyms as co-extensive categories: ‘high’ and ‘tall’ revisted. Language Sciences 25.3:263-284. Teng, Shou-hsin, Chin-Chuan Cheng, and Chin-Hsi Lin. 2006. Pintone 2006. [Computer Software]. Taipei: Graduate Institute of Teaching Chinese as a Second Language, National Taiwan Normal University. Tognini-Bonelli, Elena. 2001. Corpus Linguistics at Work. Studies in Corpus Linguistics, 6. Amsterdam & Philadelphia: John Benjamins. Tsai, Mei-Chih, Chu-Ren Huang, Keh-Jiann Chen, and Kathleen Ahrens. 1998. Towards a representation of verbal semantics: an approach based on near-synonyms. Proceedings of the 10th Conference on Computational Linguistics and Speech Processing (ROCLING-10), 34-48. Taipei: Association for Computational Linguistics and Chinese Language Processing. Zaenen, Annie. 1993. Unaccusativity in Dutch: an integrated approach. Semantics and the Lexicon, ed. by James Pustejovsky, 129-161. Dordrecht: Kluwer. 柯淑津, 黃居仁, 洪嘉馡, 劉詩音, 簡卉伶, 蘇依莉. 2007.〈中文詞義全文標記語料 庫之設計與雛形製作〉,第十九屆自然語言與語音處理研討會海報。台北: 國立台灣大學。. [Received 29 November 2006; revised 26 September 2007; accepted 1 November 2007] Siaw-Fong Chung Graduate Institute of Linguistics National Taiwan University 1, Roosevelt Road, Sec. 4 Taipei 106, Taiwan siawfongchung@gmail.com Kathleen Ahrens Graduate Institute of Linguistics National Taiwan University 1, Roosevelt Road, Sec. 4 Taipei 106, Taiwan kathleenahrens@yahoo.com. 433.
(20) Siaw-Fong Chung and Kathleen Ahrens. MARVS理論再探: 以量化觀點比較近義詞的詞義頻率與搭配詞共現值 鍾曉芳. 安可思. 國立台灣大學. 在 MARVS (Module-Attribute Representation of Verbal Semantics) 這個理 論裡,動詞的分辨是以事件訊息為基礎,而事件訊息主要包括了事件模組和 角色模組。黃居仁等 (2000) 曾以 MARVS 檢查近義詞的語意,並建議使用 此理論來凸顯近義詞間的差別。本文則針對 MARVS 的理論,加強其分析結 果,並加入兩個新的步驟。這兩個步驟分別是:一、量化比較和分析近義詞 在語料庫的詞義,二、透過 Mutual Information 的計算比較不同近義詞的搭 配詞。這兩個步驟能增加 MARVS 在語義分析的可檢驗性,也更能奠定其理 論運用在自動擷取詞彙語意上的基礎。 關鍵詞:近義詞,MARVS,詞義,搭配詞共現值,擺 b3i,放 f4ng. 434.
(21)
數據
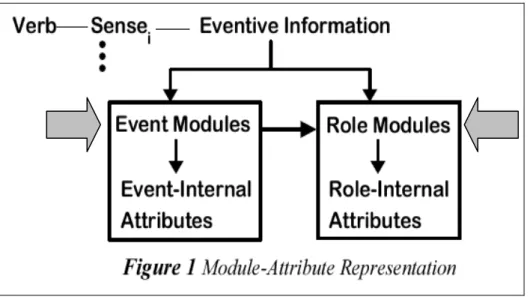



相關文件
In the context of public assessment, SBA refers to assessments administered in schools and marked by the student’s own teachers. The primary rationale for SBA in ICT is to enhance
--coexistence between d+i d singlet and p+ip-wave triplet superconductivity --coexistence between helical and choral Majorana
In Paper I, we presented a comprehensive analysis that took into account the extended source surface brightness distribution, interacting galaxy lenses, and the presence of dust
Professor of Computer Science and Information Engineering National Chung Cheng University. Chair
2 Department of Materials Science and Engineering, National Chung Hsing University, Taichung, Taiwan.. 3 Department of Materials Science and Engineering, National Tsing Hua
Do you agree with the proposed changes for the Compulsory Part of Information and Communication Technology curriculum.. Agree Disagree
Teacher / HR Data Payroll School email system Exam papers Exam Grades /.
Classifying sensitive data (personal data, mailbox, exam papers etc.) Managing file storage, backup and cloud services, IT Assets (keys) Security in IT Procurement and