Sex Differences in Smoking Behavior Trajectory Patterns and Related Factors Among Older Adults in Taiwan
全文
數據
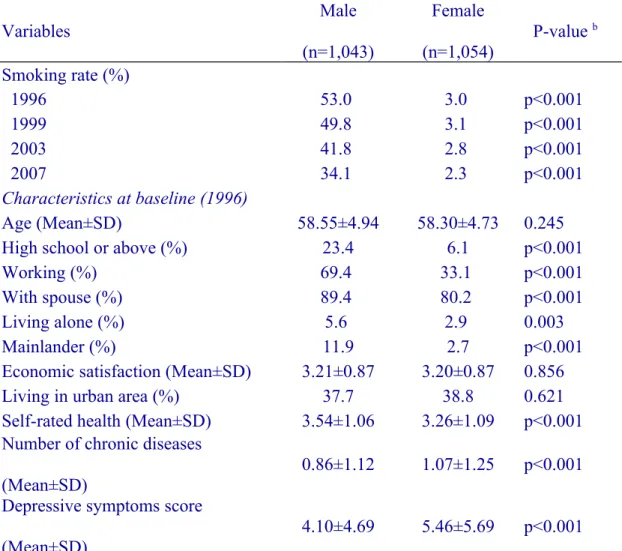
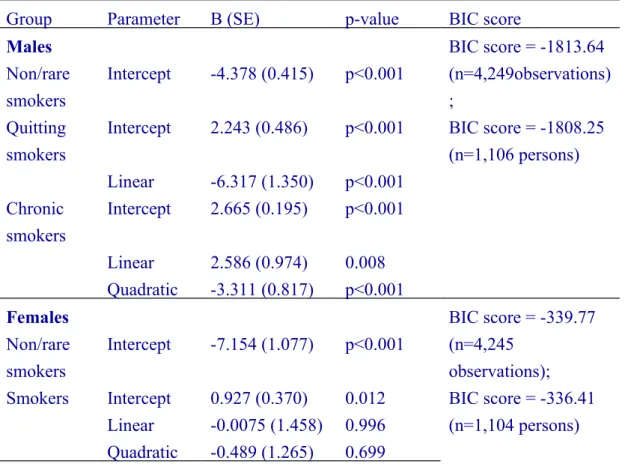
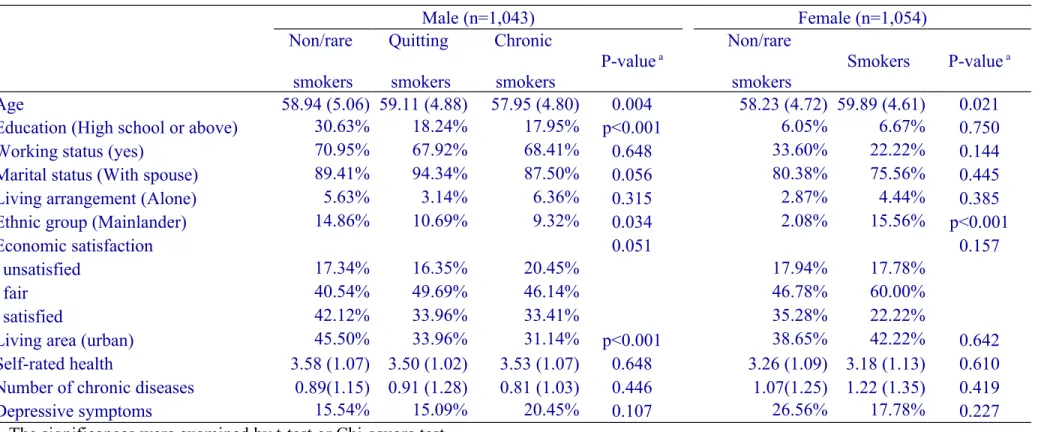

相關文件
Curriculum planning - conduct holistic curriculum review and planning across year levels to ensure progressive development of students’ speaking skills in content, organisation
Relevant topics include, but are not limited to: Document Representation and Content Analysis (e.g., text representation, document structure, linguistic analysis, non-English
Teacher / HR Data Payroll School email system Exam papers Exam Grades /.
Classifying sensitive data (personal data, mailbox, exam papers etc.) Managing file storage, backup and cloud services, IT Assets (keys) Security in IT Procurement and
However, in Taiwan, swelling can frequently occur in projects involving polished homogeneous tiles because of the large seasonal differences in temperatures and certain
• I referred to curriculum standards and 4 textbooks series (children and parents are from 4 schools) to develop research-based activities suitable for parent-child Math Camp.
(1) Parents in different ages, education backgrounds, vocations, and numbers of children in school show significant differences in teacher’s changes dimension related to
Using Structural Equation Model to Analyze the Relationships Among the Consciousness, Attitude, and the Related Behavior toward Energy Conservation– A Case Study