Peer supports on the Web for activating the zone of proximal development
全文
(2) Peer supports on the Web for activating the zone of proximal development. Abstract Most web learning systems provide discussion forums for teachers and students to exchange ideas. Although students can attain higher levels of thinking or skills if they are scaffolded by experts, such learning systems cannot mediate and include proper students in peer support activities that activate a student’s Zone of Proximal Development (ZPD). Students must actively seek answers to their learning problems in the public discussion forum to attain higher levels of thinking or skills . This study presents a decision tree methodology for activating students’ ZPD by providing peer support in a web based learning system. The experience of and guidelines for utilizing the decision tree methodology for peer support are depicted by a group problem-solving example. Meanwhile, students’conversation dialogue and the communication network of peer supports during the group problem-solving activity are explored. The proposed methodology allows teachers to go online and use the data collected to efficiently analyze the effectiveness of peer help in activating ZPD.. Ⅰ. Introduction Vygotsky’s Zone of Proximal Development (ZPD) has been widely referred to as the theoretical basis of many learning models. Students’ZPD can be activated and attain higher levels of thinking or skills if students are scaffolded by experts. These experts can be teachers, adults or capable peers. ZPD is referred to as “the distance between the actual developmental level, as determined by independent problem solving, and the potential development level, as determined by problem solving under adult 2.
(3) guidance or in collaboration with more capable peers" (Vygotsky, 1978, p.86). Faced with a large number of students, teachers cannot effectively provide scaffold for each student. Consequently, educators have attempted to develop peer support (Baker J. & Dillon G., 1999) to activate ZPD in learning environments. For instance, Guerrero (Guerrero, 2000) intermediate ESL college students collaborating on revising a narrative text written by one student to attain to higher levels of writing. However, researchers (Berger, Cohen & Zelditch, 1972, Meeker, 1981, Cohen, 1994, Chiu, 2000) have shown that the differential status of individuals in groups affects social interaction and group problem solving. Therefore, mediating knowledge exchange among students based on student characteristics is essential to create peer support for activating students’ ZPD.. Web based courses are numerous and allow students to perform various learning activities in a virtual classroom (Hiltz, 1994), including reading, messaging, conferencing, accessing documents, and participating in interactive activities. Web based curriculum environment allows students to develop their learning portfolio and interact with peers through a web learning system. Such web learning systems can record learning activities in web logs. Meanwhile, powerful and convenient web based communication facilities allow most web learning systems to provide discussion forums for teachers and students to exchange ideas. Students may freely join the discussion to seek or provide peer support, but have no responsibility to help peers develop their ZPD, since the systems do not actively enforce peer support activities such as peer assessment (Boud, 1990, Tsai et al. 2001), expert locating function (Kautz, Selman, & Milewski, 1996) (Vivacqua, 1999) to help students locate experts when they encounter learning problems , and mediate knowledge exchange appropriately for the students’ learning status. This is despite the fact the web learning systems integrate learning portfolios (Paulson, Paulson, & Meyer, 1991) such as learning products, learning behavior and learning perception on the web to obtain the learning status of students.. Computers and communications technology have made possible numerous novel approaches to group. 3.
(4) work and study. An integrated collection of tools such as CSCW (Ellis, Gibbs, & Rein, 1991) and CSCL (McConnell, 1994) is designed on the Web to enhance group performance in any particular task/knowledge domain. However, current web learning systems do not consider the status of student knowledge development, or of differences among students. The systems do not contain a model of knowledge exchange among students with different characteristics. Consequently, these systems cannot mediate and include appropriate students in peer support activities to effectively activate a student’s ZPD. Students must actively seek solutions to their learning problems in the public discussion forum.. To effectively activate students’ ZPD, the web learning systems must maintain awareness of knowledge development, implement peer help activities, and mediate appropriate student engagement in the activities. The system should answer questions such as “Can a student solve a problem independently? ” and “Where can expert peers help a student?” Researchers (see page 3 in Chiu, 2000) have investigated how status differences, including those in academic status, peer friendships, and social status, influence the effectiveness of learning in a collaborative activity. The information of ”student participation in web learning activities”, “student evaluation of his/her skill or knowledge” and “student evaluation of others’ problem solving processes” may influence the effectiveness of peer support. In conventional classrooms, teachers can assess students’ development by interactive observation, teachers can not interact with students face to face in a web-based environment. In an on-line environment teachers must closely monitor students’ learning activities, and cannot easily collect students’ activity history and perceptions of their knowledge level to observe the effectiveness of peer help in web learning systems . This problem is commonly referred to as the development awareness problem.. The other problem teachers and the web site manager encounter is the lack of support for analyzing the effectiveness of peer support among students with different statuses. Teachers can predict students’ learning performance and adopt appropriate strategies at an early stage by analyzing available learning. 4.
(5) factors (Wilhelm, 1997). The web learning system requires knowledge of status differences among students that may improve the effectiveness of peer support. For instance, the learning system must answer questions such as “who can be assigned to help a student in solving his/her learning problem?”, “what behavioral factors affect knowledge exchange in peer support activities?”, “what type of student tends to provide help and solutions?”, and “what type of student tends to have conflicts with others?” Discovering the knowledge that impacts the peer support effect involves analyzing the relationship amongst students’ learning records, including achievement in various learning concepts, web learning behavior, learning and study strategies (Weinstein, 1987), self-evaluation of his own development status, and peer help history. Therefore, teachers and web site managers require comprehensive support for obtaining the knowledge required to mediate knowledge exchange among students. This problem is referred to as the mediation knowledge problem. This study presents a methodology to activate students’ ZPD by providing peer support in a web based learning system. The methodology utilizes decision tree technologies (Quinlan, 1993) to mediate knowledge exchange among students in the system. To obtain sufficient information for inducing the knowledge required to mediate knowledge exchange, a perception acquisition mechanism is employed to obtain students’ perceptions of their knowledge development level. Such a perception acquisition mechanism allows users to feedback their perceptions of the visited web pages to web site managers, and thus provide a basis for the structure of web sites to be amended. This study employs the above mechanism to obtain students’ self-reflections on their development level by requesting feedback regarding each learning concept and skill. Furthermore, recent web servers such as Microsoft Internet Information Server provide a log mechanism to record students’ learning behavior in web learning systems. The web learning system requires no special design to record learning behaviors, since web logs are a standard feature of most commercial web servers. Therefore, students’ activity history and their perception of their knowledge level are logged to solve the development awareness problem.. 5.
(6) The proposed methodology utilizes decision tree technology to assist teachers in analyzing the relationship among developmental status, web learning behavior, study strategies and knowledge exchange in peer help activities. Decis ion tree software can help teachers to extract rules for knowledge exchange during peer help activities, and the extracted rules can be represented as a flow-chart like decision tree. Both artificial neural networks (Rumelhart, Widrow, & Lehr, 1994) can help teachers to simulate and learn pedagogical knowledge based on past classroom processes. However, the pedagogical knowledge learned using neural network software, generally in the form of a complex mathematical function, is often difficult for humans to interpret. Learned pedagogical knowledge is more difficult to communicate to humans than learned decision rules (Mitchell, 1997). However, decision tree software learns pedagogical rules from past classroom processes based using the information processing model. The learned pedagogical rules are represented as a set of decision rules resembling a flow chart. Hence, this methodology allows teachers to go online and efficiently use the collected data to analyze the effectiveness of peer help in mediating knowledge exchange among students.. The rest of this paper is organized as follows. Section Ⅱ presents the web-based learning activity setting of peer support. Section Ⅲ then describes how decision tree software can help teachers and web site managers to mediate knowledge exchange in the learning environment. Next, Section Ⅳ presents teachers’ experience in using the developed web learning system and decision tree for peer help. Finally, conclusions are presented in Section Ⅴ.. 6.
(7) Ⅱ . Web learning activity setting for peer supports This study presents a web-based learning system with peer support functions used to activate students’ ZPD. The web learning system was designed to assist fifty-seven computer science students in learning an undergraduate course entitled Java Programming Language at National Central University (Chungli, Taiwan). Following instruction in a conventional classroom, students are required to participate in the learning activities provided by the web learning system. Figure1 illustrates the web learning activities that implement peer supports for activating ZPD. The web learning system essentially provides five learning activities, namely: reading a learning dictionary, self-reflection while reading the learning dictionary, homework, peer review of homework, and group problem-solving as described below:. Students Peer Supports for Activating Group ProblemZPD Solving & Discussion. Reading, Self-Reflection, Homework, Peer Review. Mediation Knowledge Learning Dictionary. Web Behavior. Peer Review. Academic Status. Homework. Student Portfolios. Figure 1. Web activity setting of peer support for activating ZPD. l. Learning dictionary: The web learning system summarizes 174 keywords related to the skills of basic structure, graphical user interface, control structure, loop, method, recursion, array,. 7.
(8) multi-subscripted array and sorting of Java programming language. Students can browse and consult the dictionary for explanations of these keywords, with the explanations including definitions, illustrations, and examples of the keywords.. l. Self-reflection: Students are instructed to conduct self-reflection through a perception acquisition mechanism while reading the explanation of a keyword. The mechanism allows students to evaluate and feedback their development level of learning concepts and skills by stating how they understand the dictionary keywords as either “totally understand”, “mostly understand”, “ mostly not understand”, or “totally not understand”. These keyword self-reflections are recorded in the web learning system for dispatching review work during peer review activity.. l. Homework and Peer Review: Students submit homework through the web learning system. Homework includes analysis of assigned programming problems, programs, performance analysis, and the results of progra m testing. These homework artifacts are then dispatched to students for peer review. To ensure that a student can review artifacts from students with different levels of knowledge development, students’ artifacts are clustered according to keyword self-reflections. Each student is assigned six homework artifacts from students with different keyword development levels . Students assess the assigned homework artifacts and identify the keyword concepts they can learn from the artifacts. Furthermore , students also identify the keywords these artifacts need to be corrected to help peers enhance their programming skills and knowledge.. l. Group problem-solving and discussion: Students are divided into fourteen groups to collaborate on solving a programming problem. Each group contains 3 or 4 students , and the collaborative problem-solving activity lasts for two hours. A group submits only an answer to the assigned problem to establish a common group goal. Students can discuss the manner of problem. 8.
(9) solving in a discussion forum and online chat room, and the on-line dialogue is logged in the database for detailed analysis.. Leont'ev’s activity system (Leont'ev, 1978) reveals that human activities exhibit a three-level structure, namely activity, action, and operation. Using this system, the discussion forum and online chat room in the web learning system are provided based on the IBIS discussion model (Conklin & Begeman, 1988) as an instrument to allow students to interact with each other and activate ZPD. In the IBIS model, as illustrated in Figure 2, students start with an issue, which can be referred to as activities. Many positions, which can be referred to as actions in the activity system, are then proposed to resolve the issue. Next, various arguments are proposed to support or object to a certain position. Students may also suggest detailed operations in java programs to perform an action. The IBIS model is effective in collaboratively resolving design problems . Such a group problem-solving and discussion activity. allows students to seek and provide peer help to activate ZPD by proposing issues and positions.. GENERALIZES, or SPECIALIZES QUESTIONS IS-SUGGESTED-BY POSITION. REPLACES, QUESTIONS,or IS-SUGGESTED-BY. ISSUE. RESPONDS-TO SUPPORTS. QUESTIONS IS-SUGGESTED-BY ARGUMENT. OBJECTS-TO. Figure 2. IBIS discussion model. To effectively mediate peer supports, the teacher and the web learning system must encompass the mediation knowledge to know how students interact with each other and how differences in web behavior, peer review, homework, and development level of learning keywords affect peer interaction. Analysis of historical activities in the web learning system can help a teacher and the web learning system understand how status differences affect knowledge exchange during the group. 9.
(10) problem-solving activities. The teacher can induce potential decision rules that explain the quantity of issue and position of IBIS discussion activities for different types of students. The induction involves analyzing the students’ features, pair interaction between students, and the relation between these features and knowledge exchange effectiveness. For instance, the teacher must answer the following questions to effectively mediate students : l. Does any pair of students with different keyword development levels have significant degree of knowledge exchange?. l. How students ’ web behavior, peer review performance, and keyword self-reflection influence knowledge exchange in the group problem-solving and discussion activity?. l. How students’ learning and study strategies, such as motivation, anxiety, and information processing, affect the degree of knowledge exchange in the group problem-solving activity?. Since the induction process involves extensive student features and activity history, the teacher and web site managers require analytical facilities to induce the relationship between student features, self-reflection, learning strategies, and IBIS discussion behavior, and must also estimate the effectiveness of knowledge exchange. This study explores how peers interact in the group problem-solving activity. Additionally, decision tree software programs provide a convenient support for discovering decision rules based on historical data. This study also discusses the use of decision tree technologies to mediate knowledge exchange in peer help activities. Thereafter, the teachers and web learning system can then utilize the discovered rules to estimate the effectiveness of peer support.. 10.
(11) Ⅲ . Mediating knowledge exchange using decision tree technologies Decision tree technology can assist teachers in induce the relation between knowledge exchange and students characteristics. The induction analysis identifies potential groups with similar characteristics and their performance in knowledge exchange during peer support activities. Decision tree software scans the entire data set and identifies any significant relationships among the historical data. Induction is made by continuously examining the distribution of each variable from the historical data. Induction is performed mainly according to the student entropy, namely the impurity of a group of students . The induction process of decision tree programs discovers the decision rules used to classify students into several groups according to the values of identified learning variables, such as keyword development level, login frequency, learning and study strategy (LASSI), and performance in peer reviews. Each group of students has the lowest entropy in some variables and knowledge exchange performance. The decision tree method is easily implemented and does not presume advanced statistical knowledge. Consequently, this method provides a relatively fast and simple means of analyzing the students’ peer support behavior online and discovering rules with the potential to improve the effect of knowledge exchange. Figure 3 illustrates how to apply decision tree technology to analyze student reactions during the peer support activity.. 11.
(12) Web learning Behavior Keyword Development Level. Decision Tree Software. deci. sion r. ule. High. Group2 Middle. decision rule 2. LASSI Pair interaction history. Group1. e1 rul n o isi d ec rule 2 d eci sion. 4. Group3. Group4. Low. Groups with similar features. Knowledge Exchange Effectiveness. Figure 3. Decision tree analysis – discovering potential groups and their trends of knowledge exchange effectiveness. Learning and study strategy analysis. Recently developed decision tree software programs such as C5.0/See5 (Quinlan, 1993) and IBM DB2 Intelligent Miner assist decision makers in exploring decision rules in historical records. Many business decision support systems, such as Blue Martini Software (1998) and Clementine (2000), employ decision tree technology to predict market share, assess financial risks, and diagnose faults . This study uses decision tree software C5.0 to discover how students with different learning and study strategies and keyword development levels exchange knowledge during group problem-solving activities.. The diagnosis of individuals’ learning process by researchers involved not only learning habits but also primary strategies such as information processing and problem-solving. Weinstein (Weinstein, 1987) developed learning and study strategies inventory (LASSI) to diagnose student motivation, time management, attitude, concentration, anxiety, information processing, selecting main ideas, self-testing, and test strategy. LASSI can be employed to diagnose student learning problems and problem solving. 12.
(13) abilities. Teachers and learning systems can provide different peer supports during group problem-solving activit ies according to students’ learning and study strategies. Statistical analysis reveals how individual learning strategies affect knowledge exchange among students. However, precisely how these strategies combine and affect knowledge exchange in the peer support activity requires a complex classification of students’ performance in knowledge exchange. Decision tree software programs provide instruments for discovering the decision rules that can classify student performance in peer support activities. Anxiety <= 1: :...Motivation <= 2: :...SelfTesting <= 2: Low (10.5/3.3)…………………………………………….G1 : SelfTesting > 2: High (6.1/1.0)……………………………………………… G2 Motivation > 2: :...SelfTesting <= 1: 1 (2.4/1.2) SelfTesting > 1: :...Attitude <= 1: 3 (2.8) Attitude > 1: :...InformationProcessing <= 2: 3 (2.5/1.0) InformationProcessing > 2: :...StudyAids <= 1: 3 (3.1) StudyAids > 1: High (6.9/2.0)………………………………… G3 Anxiety > 1: :...Anxiety > 2: Low (4.9/1.0)………………………………………………………….G4 : Anxiety <= 2: : :...Attitude <= 2: 1 (3.6/0.9) : Attitude > 2: 2 (2.2). G1: Performance =Low 10.5/3.3. 2 <=. Self-Testing. G2: PerformanceA =High 6.1/1.0. > 2. 2 <=. ..... Motivation. 1 <=. >2 <=1. Self-Testing. >1. .... Attitude. ..... 1 <= >1. 2 <=. InfoProcess >2. Anxiety. StudtAids >1. >2. Anxiety. G4: Performance =Low 4.9/1.0 .... <=2. 1 <= >1. G3: Performance =High 6.9/2.0. Figure 4. Decision tree regarding students ’knowledge exchange performance. This study uses a decision tree software C5.0 to discover how students with different learning and study strategies provide and seek peer support during group problem-solving activities. The decision. 13.
(14) tree analysis attempts to discover the relationship between students’ performance in knowledge exchange and learning and study strategies. Students’ performance in knowledge exchange are measured based on the logged dialogues of the group problem-solving activity. The knowledge exchange performance is classified into three categories, High, Middle, and Low, according to the number of issues and positions proposed by students. C5.0 is then used to analyze how student learning and study strategies influence knowledge exchange performance, including strategies such as attitude, motivation, time management, anxiety, concentration, information processing, selecting main idea, study aids, self testing, test strategies . After feeding the value of variables, C5.0 generates a decision tree to explain how different types of students behave in peer support activities.. Figure 4 illustrates the simplified decision tree induced by C5.0 that explains students ’ performance in knowledge exchange using their learning and study strategies. The induction accepts forty-five students’ features in attitude, motivation, time management, anxiety, concentration, information processing, selecting main idea, study aids, self testing, test strategies and knowledge exchange performance in group problem-solving activities. Each student group (black node) in the decision tree represents a type of student with generally similar knowledge exchange performance. A group of students in the decision tree thus depicts a decision rule for explaining knowledge exchange performance. The significant rules, those with over four students in corresponding groups in the decision tree, are described below: l. Group G1 refers to students who are not anxious (Anxiety <=1), have fairly low motivation (Motivation<=2) and self-testing ability (Self-Testing<=2), and moreover tend not to exchange positions and issues with others (Performance=Low). Indication 10.5/3.3 reveals that on average, among 10.5 students of this type, 7.2 had low knowledge exchange performance.. l. Student group G2 contains students very similar to those in G1, except that. 14.
(15) they have extremely high self-testing ability (Self-Testing>2), and these students tend to actively exchange knowledge with others . Among 6.1 students of this type, an average of 5.1 (by the indication of 6.1/1.0) have high performance in knowledge exchange. l. Student group G3 includes students with relatively high motivation, self-testing ability, learning attitude, information processing skill, and study aid skills , and these students also tend to actively exchange knowledge with others. Among 6.9 students of this type, an average of 4.9 (by the indication of 6.9/2.0) perform well in knowledge exchange.. l. Student group G4 describes students who are extremely anxious (Anxiety >2), and these students tend not to exchange ideas with others. Among 4.9 students of this type, an average of 3.9 (by the indication of 4.9/1.0) have low knowledge exchange performance.. The teacher can examine the effectiveness of group problem-solving activity for each student by using the induced decision tree. The decision tree contains precise conditions of learning and study strategies in which the students could increase their levels of knowledge exchange. Therefore, the designers of the learning web system can also embed decision rules in the learning system to mediate students in group activities and thus optimize the effectiveness of group problem-solving activity.. Pair interaction analysis. The decision tree analysis of learning and study strategies depicts how individuals react to the group problem-solving activity. To determine how each individual interacts with peers of different development levels requires analysis of the pair difference of student knowledge status. The pair. 15.
(16) difference refers to the difference of development levels between two students determined by their homework. Since students submit their homework through the web learning system, teachers can assess students ’ homework online. Rather than using a score to represent students ’ development level, this study assesses students ’ keyword development levels by analyzing patterns in their homework. For instance, a student may incorrectly define static methods in her/his homework. Such patterns are identified and recorded to analyze their performance in knowledge exchange during the group problem-solving activities. Table 1 lists frequently occurring keyword development codes. Keyword development code. Related Keyword. Description. 31 32 70 72. String String Method Method. 10 12 50 51 80. Basic structure Basic structure For loop For loop GUI. 82. GUI. 20 21 22. Variable Variable Variable. Using function “CompareTo” to compare strings Without handling strings Correctly invoking methods Incorrectly defining or invoking methods Incorrectly dealing arrays when invoking methods Incorrectly defining static methods Using correct basic structure Using incorrect “{}” structure and applet structure Correctly using For loop Never using For loop Correctly using JTextField,JButton,JTextArea Correctly using JLabel,JTextField,JTextArea Correctly using JButton,JLabel,JTextField,JTextArea Correctly using JOptionPane,JTextArea Correctly using JButton,JTextField Correctly using JButton,JLabel,JTextField Correctly using JLabel,JtextField Incorrectly using JTextField Incorrectly using Container Incorrectly using getContentPane Lacking of “()” when using actionPerformed Incorrectly using JoptionPane Correctly declaring variables Using variable without declaration Variable declaration errors. Table 1. Keyword status identified by analyzing homework. Decision tree analysis can induce the match model of pairs thus optimizing peer help effectiveness. The match model reveals which pairs of students contribute more issues, positions, and arguments in the group problem-solving activity. Before decision tree analysis, a student’s keyword development levels are combined with the keyword development levels of his/her learning peers to create dual codes. For instance, if a student has a keyword development level of 31, while one of his/her peers has a keyword development level of 32 for the keyword String, this pair of students is encoded as 3132 to represent the keyword development differences. Decision tree software can help teachers to analyze the. 16.
(17) relationship between keyword development difference and knowledge exchange for corresponding pairs of students .. The analysis involves sixty-six pairs of keyword development levels and corresponding knowledge exchange records. Table 2 lists the rules of keyword development difference that explain the pair condition in which students perform well in knowledge exchange. For instance, decision rule 1 indicates the pairs in which one student has keyword development level of 31(used function “CompareTo” to compare strings) and 70 (correctly invoked methods) while the other could not handle strings (code 32) and used methods incorrectly (code 72). Among 7 of such pairs, 66.7% produced many positions during group problem-solving activity, allowing teachers to identify which status differences improved the knowledge exchange rate. Decision rule. Keyword Status Condition. Effectiveness. Cover/Correctness. 1. String = 3132 and. Position High. 7/0.667. Position High. 6/0.667. Position High. 4/0.829. Issue High. 4/0.834. Issue High. 6/0.636. Argument High. 4/0.697. Method = 7072 2. Constr = 1012 and String = 3132. 3. String = 3132 and For = 5051 and Method = 7072. 4. For = 5151 and GUI = 8082. 5. var = 2022 and GUI = 8080. 6. var = 2122 and Method = 7072. Table 2. Significant rules of keyword status difference. To actively implement peer support, the web learning system and teachers require mediation knowledge of status differences among students, which may enhance the effect of peer support. The mediation knowledge yielded by decision tree analysis can be used in two ways. First, this knowledge. 17.
(18) can be used to regulate collaborative learning activities. For instance, knowledge of the relationship between students ’ status and knowledge exchange performance can help teachers to organize students in group problem-solving activities to generate more issue and position knowledge exchange. Second, the knowledge can be embedded in web learning systems to automatically perform a teacher’s functions in activating peer supports. For instance, the system can automatically dispatch a learning question to a capable student who is likely to respond to others ’ questions. The knowledge yielded by decision tree analysis contains decision rules for inferring students ’ knowledge exchange performance. Therefore, decision tree software provides an operational inference mechanism to automatically perform the tasks of teachers.. 18.
(19) Ⅳ. Experimental result The web learning system and peer support activities were evaluated at the end of the course (week 16) for their effectiveness in knowledge exchange. The primary data analysis techniques included issue and position knowledge exchange analysis, communication network (Milson, 1973) analysis of dialogue in group problem-solving, keyword self-reflection, behavioral analysis of web logs, and learning and study strategies inventory. The following charts describe students’ reactions in during the peer support activities.. Knowledge exchange during group problem-solving. Forty-six students participated in the group problem-solving activity. Analysis of peer dialogue confirms that twenty-eight students (61%) asked or answered questions from peers; twenty-one (46%) students asked for peer support by proposing issues ; twenty-one (46%) students provided peers support by proposing positions in response to the issues raised by others; and fourteen students (30%) actively provided and asked for peer supports during the group problem-solving activity. Students raised eighty-one issues during the two hour activity period. Of these issues, fifty-nine (73%) were responded while twenty-two (27%) were not. Students totally proposed a total of seventy-seven positions in response to the fifty-nine issues. Each issue stimulated an average of 1.54(91/59) positions in response. Therefore, most students ’ learning problems met with peer support. Figure 5 and Table 3 present students ’knowledge exchange behavior in the group problem-solving activity.. 19.
(20) Number. of. Number of students. students proposed. proposed. 14 Students. issues: 21 Students. positions:. 21 Students. Number of students participated in the activity: 46. Figure 5. Students ’knowledge exchange behavior in the group problem-solving activity. Issues proposed. 81. Issues answered. 59. Issues without answer. 22. Positions. 91. Positions per Issues. 1.54. Table 3. Issues and positions raised in group problem-solving activities. Table 4 summarizes the knowledge exchange behavior for each group. Knowledge exchange occurred in twelve groups (86%). Eight groups (57%) raised over five issues during the activity. Six groups (43%) proposed over five positions in response to raised issues. Seven students (in groups 1, 2, 5, 6, 12, 13, 14) merely responded to issues raised by others, and did not propose any issues themselves. Furthermore, another seven students (in groups 1, 5, 6, 13, 14) merely proposed issues themselves and did not respond to the issues raised by others. Therefore, 25% (by 7/28) of participating students who exchanged knowledge with peers acted solely to seek help themselves or to provide help to others. 1. 2. 3. 4. 5. 6. Issue (Problem). 13. 8. 2. 0. 3. 2 14 11. 3. 0. 7. 6. 7. 5. 81. Position (Solution). 12 18. 1. 0. 2. 1 13 17. 3. 0. 9 12. 1. 2. 91. 1. 0. 2. 1. 1. 0. 2. 1. 2. 21. Group. Issue Provider. 2. 3. 20. 7. 3. 8. 2. 9 10 11 12 13 14 Total. 1.
(21) Position Provider. 1. 4. 1. 0. 2. 1. 3. 2. 1. 0. 2. 2. 1. 1. 21. Provider. 0. 3. 1. 0. 1. 0. 3. 2. 1. 0. 2. 1. 0. 0. 14. Observed Students. 4. 4. 4. 3. 4. 2. 3. 3. 3. 3. 3. 3. 3. 4. 46. Intersection of Issue and Position. Table 4. Group knowledge exchange in group problem-solving activity. A questionnaire containing seven Likert-scale items was administered to measure the perceived usefulness (Davis, Bagozzi & Warshaw, 1989) of the web based group problem-solving system. Among thirty-three respondents, twenty-two (67%) believed that the web problem-solving system enabled them to learn concepts/skills more quickly; twenty-one (64%) thought the web problem-solving system would improve their learning performance; twenty-one (64%) felt that using the web problem-solving system would increase their learning productivity of related concepts/skills ; twenty-three (70%) considered that the web problem-solving system would enhance the effectiveness of learning related concepts/skills ; nineteen (54%) indicated that the web problem-solving system would facilitate learning; And twenty-one (64%) considered the web problem-solving system useful in their learning. Table 5 lists the all of the students’ responses on perceived usefulness, and indicates that the web learning system is useful. Question. Likely. Unlikely. 1. Using the web problem-solving system would enable me to learn concepts/skills more quickly.. 5. 9. 8. 6. 3. 0. 0. 4. 10. 7. 5. 4. 1. 0. 5. 4. 12. 8. 2. 0. 0. 3. 8. 12. 4. 2. 2. 0. 3. 11. 5. 7. 5. 0. 0. 4. 7. 10. 6. 3. 1. 0. 2. Using the web problem-solving system would improve my learning performance. 3.Using the web problem-solving system in my learning would increase my learning productivity of related concepts/skills. 4. Using the web problem-solving system would enhance my effectiveness on learning of related concepts/skills. 5. Using the web problem-solving system would make it easier to learn. 6. I would find the web problem-solving system useful in my learning. 21.
(22) Table 5. Perceived usefulness of the web problem-solving system. Interaction dialogue analysis Student dialogue records were analyzed to elucidate the peer interaction in the group problem-solving activity. Dialogue analysis indicates that students conducted nine main formats of interaction during the activity. Analytical results enable management and quality assessment of similar peer support activities. The interaction formats include issues , positions, arguments, group developments, responses, acceptance of responses, objections to responses, conflict, and support request, as described below: l. Issues represent what needs to be done and problems to be solved, and relate to the concepts and skills being learned by students. For instance, students may seek peer support to solve a learning problem by raising questions such as “What should I import in our program?”, “What does ‘increasing strings’ mean?”, and “How can I record the input words as a string after pressing a button?”.Positions represent methodologies for resolving an issue, and are answers from peers in response to issues that have been raised. Students may help others by responding to issues. For instance, students answered questions raised by others by proposing positions such as “Just import javax.swing.*”, “Use String str=e.getSourceCommand();” and “State c.getContentPane allows you to create a location in your window where information can be stored.”. l. Arguments represent opinions that support or object to a position. For instance, students comment on the positions of others by statements such as “Oh, right. Then I should use another variable” or “No, that’s more troublesome”Group development represents questions raised to coordinate members to work together. For instance, when students coordinated the process of problem solving, questions such as “Does anyone know how to write the program?”, “Who is my partner?”, ”Does anyone have any opinions on this problem?”, and “Who knows how to develop the algorithm?” were frequently raised in the dialogue.. 22.
(23) l. Response represents a suggested answer to a group development question. For instance, students frequently respond to the development questions of others with comments such as “Actually, we can try page 289.” and “I’ve developed a draft graphical user interface. I think that’s enough.”. l. Acceptance of response represents the acceptance of a response. For instance, students frequently agreed to the responses of others with comments such as “okay! I will wait for you.” and “I agree to try page 289.”. l. Objections to response represents objection to responses. For instance, students frequently dis agreed with the responses of others with comments such as “I do not have JDK. How can I write that program?”, “No, I can not finish the first part of the program.” and “No, why don’t you transfer it into text file?”. l. Support request represents a request for resources and help from other group members. For instance, students frequently request resources with comments such as “I don’t have the example. Can you give it to me?” and “Has anyone finished the program? I have only part finished.”. l. Conflicts occurred among group members. For instance, dialogues such as “Go away, don’t interrupt the discussion.” and “idiot!!” occurred in the dialogue. These type of interaction occurred when a group members were unable to obtain support from other group members.. Dialogue analysis confirms that students frequently raised group development questions. Objections to responses are more common than acceptance. Conflicts occurred when individuals were unable to obtain resource support from peers. Besides position, students frequently ask for resource support from others. Table 6 lists the frequency of each type of interaction. Time sequence analysis of the dialogues indicates that positions generally outnumbered issues , and issues were proposed and positioned increasingly during the initial stage of the activity. However, following the middle of the initial stage, issues and positions decreased dramatically. Therefore, knowledge exchange was concentrated during the early stage of the group problem-solving activity. Figure 6 displays the time sequence of issues,. 23.
(24) positions, and arguments for all groups. Issue. 81. Position. 91. Argument. 30. Group development. 298. Response. 104. Acceptance of response. 59. Objection to responses. 80. Conflict. 33. Support request. 98. Table 6. Frequency of interaction dialogue. 25 20 ISSUE Position Argument. 15 10 5. 1:2 0~ 1:3 0 1:3 0~ 1:4 0 1:4 0~ 1:5 0 1:5 0~ 2:0 0 2:0 0~ 2:1 0 2:1 0~ 2:2 0 2:2 0~ 2:3 0 2:3 0~ 2:4 0 2:4 0~ 2:5 0 2:5 0~ 3:0 0 3:0 0~ 3:1 0 3:1 0~ 3:2 0 3:2 0~ 3:3 0. 0. Figure 6. Time sequence of issues, positions, and arguments. Time sequence analysis of dialogue also indicates two peaks of group development frequency. Initially, students frequently communicate to develop tentative solutions. Meanwhile, during the middle stages of group problem-solving activity, students began to communicate to refine these solutions. Frequency of response and support requests correspond with the frequency of group development. Support requests peaked around the middle of the activity because students require more resource support and solution revision from others after they have developed the initial solution. Time sequence analysis of the dialogue also indicates that conflicts were frequent during the initial stage of the activity, before the students began to propose issues and group developments. Frequency of response acceptance and. 24.
(25) objection are analogous to the frequency of responses. Figures 7 and 8 display the time sequence of group developments, responses, conflicts, and support requests for all groups.. 20 18 16 14 12 10 8 6 4 2 0 1:2 0~ 1:3 0 1:3 0~ 1:4 0 1:4 0~ 1:5 0 1:5 0~ 2:0 0 2:0 0~ 2:1 0 2:1 0~ 2:2 0 2:2 0~ 2:3 0 2:3 0~ 2:4 0 2:4 0~ 2:5 0 2:5 0~ 3:0 0 3:0 0~ 3:1 0 3:1 0~ 3:2 0 3:2 0~ 3:3 0. Group development Respondence Support request. Figure 7. Time sequence of group development, response, and support request. 14 12 10 8 6 4 2 0. Respondence Objection Conflict. 3:20~3:30. 3:10~3:20. 3:00~3:10. 2:50~3:00. 2:40~2:50. 2:30~2:40. 2:20~2:30. 2:10~2:20. 2:00~2:10. 1:50~2:00. 1:40~1:50. 1:30~1:40. 1:20~1:30. Respondence acceptance. Figure 8. Time sequence of acceptance of response, objection to response and conflicts. Communication network analysis. Student dialogue during the group problem-solving activity is analyzed to reveal the nature of student interaction. Meanwhile, group knowledge exchange is revealed via a communication network (Milson, 1973). The network contains directed lines linking two students, indicating that one proposed an issue and the other offered a suggestion in response. The number in the line indicates the number of issues answered. Figure 9 displays the communication network for each group.. 25.
(26) 2. 2. 1. 7. 1. 2. 5. 5. 7. 89089000. 89086900. 89089300 2. 5. 1 1 5. 89089100. 89127200. 7. 6. 6 3. 1. 89090000. G1. 89127800. 89127400. G2. 6. 5. 89087600. 89087800. G3. 5 89128000. 89087700. 1. 1 89090700. 5. 4 89090600 1. 1. 1. 2. 6. 89134300. 89134500. 89127600. G4. G5 3. 6. 1. 1. G6. 6. 5. 4. 2. 2. 3. 89087300. 3. 5. 89087100. 1. 89086800. 1. 1. 2. 5. 3. 89088900 89088000. 89088800. 89088100. 89088200. 89089700. 1. 2. 1. 1. 4. 1. 89090200. 89089400. 89089900. G8. G9. 2. G7. 1. 1. 5. 1 89089800. 4. 4. 5. 89086700. 89090100. 89087200. 6. 3. 89090400. 89090800. 89090300. G10 2. G11 4. 89087500. 1. 4. 5. 4. 5. G12. 5. 2. 88024000. 89090500. 89088500. 2. 1. 4. 6. 89090900. 89091000. G13. 4 89148300. G14. Figure 9. Communication network of knowledge exchange Keyword. Keywords. Self-reflection reflected. Login. Homework. Frequency. Peer Review. 26. & Mid-term. Final exam Students.
(27) 2.14/1.08. 159.47/48.59 393.36/142.03 13.89/3.87. 3.59/1.20. 74.47/13.79. 57. Type 1 1.93/0.36. 157/40.27. 442.00/90.48 15.4/2.05. 3.44/0.44. 77/13.79. 10. Type 2. 80/80.40. 213.00/196.65 11.4/5.31. 3.20/1.39. 61/21.16. 7. Type 3 1.81/0.36. 138/55.86. 352.00/130.10 14.4/2.61. 4.75/0.35. 80/4.94. 2. Type 4 2.45/0.33. 180/8.82. 459.00/152.57 12.7/1.85. 2.31/0.84. 66/11.97. 8. Type 5 3.06/0.38. 182/5.63. 429.00/59.44 14.4/2.40. 4.28/0.40. 81/9.21. 18. Type 6. 3.52/0.17. 183/3.54. 569/93.35. 11.9/6.77. 4.21/0.55. 80.4/18.11. 5. Type 7. 1.1/0.289. 117/65.30. 244.00/133.28 13.7/1.44. 4.87/0.13. 76/9.16. 3. 177/2.5. 382.00/22.33 10.6/7.36. 1.72/0.00. 68/11.31. 4. All. 0.21/0.35. Outlier 1.13/1.32. Table 7. Student portfolio clusters and characteristics (Mean/Standard Deviation). Students ’ portfolios are analyzed to reveal how students with different statuses differ in exchanging knowledge. The portfolios include students ’ development levels of keywords self-reflected during browsing learning dictionary, the number of keywords evaluated by students, the frequency of logging in to the learning system, homework and peer review scores, and mid-term and final exam scores. K-means cluster analysis identified seven different clusters of students , and Table 7 lists the characteristics of each cluster and the mean and standard deviation for each measure.. The characteristics of each cluster of students are described as follows: l. Cluster 1 students participated diligently in homework and peer review. Their mid-term and final exam score were around the middle of the range compared to the rest of the students, while their self-reflection on keywords status was lower than average.. l. Cluster 2 students obtained relatively low scores in the mid-term and final exams. Furthermore, they seldom logged on to the learning system and evaluated their keyword development levels themselves.. l. Cluster 3 students obtained relatively high scores in the mid-term and final exams. Despite this however, they tended not to self-reflect their keyword status in the learning system.. l. Cluster 4 students rated their keyword development levels highly, but their mid-term exam results did not correspond with this evaluation.. l. Cluster 5 students rated their keyword status highly and obtained corresponding high scores in the mid-term and final exams.. l. Cluster 6 students resemble cluster 5 students, but tended to log on to the system very frequently.. 27.
(28) l. Cluster 7 students obtained middling scores in the final exam and higher scores in the mid-term exam.. Meanwhile, they seldom logged on to the system and self-reflected their keyword status.. Students from clusters 3, 5, 6, and 7 are more able than those from clusters 2 and 4. Students in cluster 1 are characterized by active participation in learning activities such as homework and peer review. Each student in the communication network is tagged to the node corresponding to the cluster number of the student’s portfolio in Fig. 9. Analysis of the communication network and students’ portfolios indicates that the communication network of a group may differ according to the distribution of students’ ability levels in the group. Communication network formats and ability level distribution are described below:. l. Centralized knowledge exchange: where a student became the center of knowledge exchange in a group. This student suggested many responses to issues raised by others, and other students frequently requested this student’s support. In such group, the student occupying the position of knowledge center has well-established abilities to solve related problems , while other students have middling or above average problem solving abilities. Group 1 in Fig. 9 is an example of this sort of group.. l. Distributive knowledge exchange: where knowledge exchange did not converge on a single student. In such a group all of the students exchange knowledge with each other, and usually have middling or above average problem solving abilities. However, some individuals were unable to solve the problem assigned. Consequently, students had numerous very distributive knowledge exchanges during the group problem-solving activity. Groups 2, 7, and 12 are examples of such a knowledge exchange group.. l. Group development impediment: Groups 3, 4, 6, and 10 are examples of this sort of knowledge exchange group, which involves little group development, provision of responses , and acceptance. 28.
(29) of responses among group members. In such a group, students generally have middling or above average problem solving abilities. However, students did not converge on a common approach to solving the assigned problem. Consequently, knowledge exchange to develop the ZPD of others. was very limited in such groups.. l. Ability impediment: Group 13 is an example of this sort of knowledge exchange group. In such a group, students generally have very limited problem solving abilities. Conflicts among members are frequent in such groups, generally occurring when a student was unable to obtain support from others. Therefore, knowledge exchange is also very limited in such groups.. l. Partial knowledge exchange: Groups 8, 9, 11, and 14 are examples of this sort of knowledge exchange group. In such groups, the range of problem solving abilities is diverse. Knowledge exchange occurred among students with middling or above abilities, while students with limited abilities were unable to participate. Another example of such a group was group 5. This group also contained students with diverse levels of problem solving abilities, but they failed to converge on a common process for solving the assigned problem. Students in this group tended to object to the responses of others rather than to accept them. In this case, students with middling or above abilities suffered from limited knowledge exchange.. Analysis of students ’ knowledge exchange behavior and their portfolios also confirms that the difficulty and the abilities required to solve the assigned problems may affect knowledge exchange among students. Communication network and student portfolio analysis confirms that student cluster 1 tended to actively exchange knowledge with peers during the group problem-solving activity. Among ten such students, six actively exchanged knowledge with others (proposed issues or positions more than three times), and a further t wo exchanged knowledge at least once. However, students in clusters 2 and 4 did not exchange knowledge with peers very actively. Among the seven students in cluster 2,. 29.
(30) only two of them exchanged knowledge even once, while among the eight students in cluster 4, only one exchanged knowledge more than three times, and two did so once. The above results may arise partly because the experiment did not assign different problems to students according to their core abilities. The students in clusters 1, 5, and 6 had sufficient ability to exchange knowledge with others and develop their ZPDs. Figure 10 lists the complete results concerning the knowledge exchange for different clusters of students .. 7 6. Knowledge Exchange>3. 5 4. 0<Knowledge Exchange <=3 Knowledge Exchage=0. 3 2 1 0 Cluster Cluster Cluster Cluster Cluster Cluster Cluster 1 2 3 4 5 6 7. Figure 10. Knowledge exchange behavior for students of seven clusters. Ⅴ. Conclusion As students learn and communicate on the World Wide Web, learning systems require mechanisms to mediate and include appropriate students in peer support activities to activate students’ ZPD. This study has described how teachers and learning systems should effectively mediate peer help and activate ZPD by managing knowledge exchange. This work proposed a decision tree methodology for discovering the rules of knowledge exchange concerning individual learning strategies and pair differences of students . The experiment and guidelines for utilizing the decision tree methodology in peer support is demonstrated by a group problem-solving example. The experiment revealed the format of dialogues of. 30.
(31) group activities and communication networks. Cluster analysis of students’ portfolio revealed that the communication network of a group may differ according to the distribution of ability levels within the group, and the difficulty of solving the assigned problem. The teacher and the learning system can examine the effectiveness of the group problem-solving activity and mediate students to optimize that effectiveness. Therefore, teachers employing a web-based learning system can also extend the use of decision tree technology for other peer support settings with relative ease.. Acknowledgements The authors would like to thank the National Science Council of the Republic of China for financially supporting this research under Contract No. NSC 90-2511-S-155-004.. Reference Baker J. & Dillon G. (1999). Peer Support on The Web, Innovations in Education and Training International, 36:1, pp. 65-79. Berger J., Cohen B. P. & Zelditch M. (1972) Status characteristics and social interaction, American Sociological Review, 37:241-56. Blue Martini Software(1998), URL is http://www.bluemartini.com/ . Boud D. (1990). Assessment and the promotion of academic values, Studies in Higher Education, 17, 185-200. Chiu M. M. (2000). Effect of status on solutions, leadership, and evaluations during group problem solving, Sociology Education, 73(3), pp. 175-195. Clementine (2000) URL is http://www.isl.co.uk/. Cohen E. G. (1994). Reconstructing the classroom: Conditions for productive small groups, Review of Educational Research, 63:1-35. Conklin J., & Begeman M. L. (1988). gIBIS: A Hypertext Tool for Exploratory Policy Discussion, ACM Transactions on Information Systems, 6:4, pp.303-331. Davis, F.D., Bagozzi R.P. and Warshaw P.R. (1989). User acceptance of computer technology:A comparison of two theoretical models, Management Science , 35( 8) , pp.982-1003. Ellis C. A., Gibbs S. J., & Rein G. L. (1991). Groupware: some issues and experiences, Communication of the ACM, 34(1), 35-58. Hiltz S. R. (1994) The virtual classroom: learning without limits via computer network , Alex. 31.
(32) Publishing Corporation, Norwood NJ. Kautz H., Selman B. & Milewski A. (1996). Agent Amplified Communication, in Proc. of the 13th National Conf. on Artificial Intelligence, pp. 3-9, Portland, Oregon. Leont'ev, A. N. (1978). Activity, consciousness, and personality. Englewood Cliffs: Prentice-Hall. Maria C. M. De Guerrero (2000). Activating the ZPD: Mutual Scaffolding in L2 Peer Revision, The Modern Language Journal, Vol 84 (1), p51-68. McConnell D. (1994). Managing open learning in computer supported collaborative learning environments, Studies in Higher Education, Vol. 19 Issue 3, p341-359. Meeker, B. F. (1981). Expectation states and interpersonal behavior, in Social Psychology: Social Perspectives, edited by M. Rosenberg and R. H. Turner, Pp. 290-319, New York: Basic Books. Milson, F.(1973). An introduction to group work skill, London: Routledge and Kegan Paul. Mitchell T. M.(1997). Decision tree learning in Machine Learning, McGraw-Hill Company, 1997. Pauls on F. L., Paulson P. R., and Meyer C. A. (1991). What makes a portfolio a portfolio?, Educational Leadership, 48:5, pp. 60-63. Quinlan J. R. (1993). C4.5 Programs for machine learning, Morgan Kaufmann Publishers, San Mateo, California. Rumelhart D., Widrow B. & Lehr M.(1994). The basic ideas in neural networks, Communication of the ACM, 37:3, pp. 87-92. Tsai C.C., Liu E. Z. F., Lin S. S. J., & Yuan S. M.(2001). A networked peer assessment system based on a Vee heuristic, Innovation in Education and Teaching International, 38-3, 220-230. Vivacqua A. S. (1999), Agents for Expertise Location, in Proc. of AAAI Spring Symposium on Intelligent Agents in Cyberspace, Technical Report SS-99-03, Stanford, CA, USA. Vygotsky, L. S. (1978). Mind in society: the development of higher psychological processes. Cambridge: Harvard University Press. Weinstein, C.E.,Learning and Study Strategies Inventory (LASSI), User's Manual, 1987. Wilhelm K. H. (1997). Use of an expert system to predict language learning success, System: An International Journal of Educational Technology and Applied Linguistics, 25:3, pp. 317-334.. 32.
(33)
數據
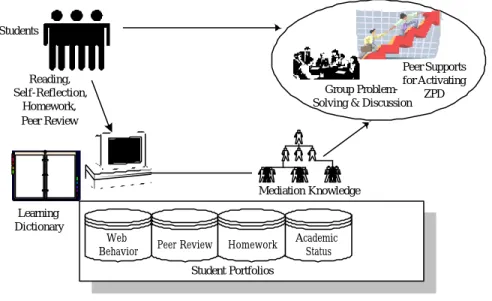



Outline
相關文件
• To introduce the Learning Progression Framework (LPF) as a reference tool for designing a school- based writing programme to facilitate progressive development
stating clearly the important learning concepts to strengthen the coverage of knowledge, so as to build a solid knowledge base for students; reorganising and
A Journey on Learning the Arts for Senior Secondary Student.
Like the proximal point algorithm using D-function [5, 8], we under some mild assumptions es- tablish the global convergence of the algorithm expressed in terms of function values,
• elearning pilot scheme (Four True Light Schools): WIFI construction, iPad procurement, elearning school visit and teacher training, English starts the elearning lesson.. 2012 •
• Environmental Report 2020 of Transport Department, Hong Kong: to provide a transport system in an environmentally acceptable manner to align with the sustainable development of
Teachers can design short practice tasks to help students focus on one learning target at a time Inferencing task – to help students infer meaning while reading. Skimming task –
DVDs, Podcasts, language teaching software, video games, and even foreign- language music and music videos can provide positive and fun associations with the language for