以認知學習修正XCS建構具知識教育與機械學習之雙模式學習機制—以財務資料預測之知識學習為例
87
0
0
全文
(2) 以認知學習修正 XCS 建構具知識教育與機械學習之雙模式學習 機制—以財務資料預測之知識學習為例 Applying Cognitive Learning to Enhance XCS to Construct a Dual-Mode Learning Mechanism of Knowledge-Education and Machine-Learning — an Example of Knowledge Learning on Finance Prediction. 研 究 生:陳怡璋. Student:Yi-Chang Chen. 指導教授:陳安斌 博士. Advisor:Dr. An-Pin Chen. 國 立 交 通 大 學 資 訊 管 理 研 究 所 博 士 論 文. A Dissertation Submitted to Institute of Information Management College of Management National Chiao Tung University in Partial Fulfillment of the Requirements for the Degree of Doctor of Philosophy in Information Management July 2005 Taipei, Taiwan, the Republic of China. 中華民國九十四年七月.
(3) 以認知學習修正 XCS 建構具知識教育與機械學習之雙模式學習機制 —以財務資料預測之知識學習為例 學生:陳怡璋. 指導教授:陳安斌 博士. 國立交通大學資訊管理研究所. 摘要 自 1956 年以來,人工智慧所定義的機器學習與長久以來研究人類心智行為的心理 學所定義的學習,兩者明顯不同。由於電腦運算能力的提升,使得我們可以有能力再 次重新檢視學習的定義,以此希望可以達成更高效率與準確率的智慧學習模型。 本研究企圖以認知心理學之認知結構來修正自 1956 年以來人工智慧之發展,由於 人工智慧長期侷限於試誤學習之低效率學習模式,然而試誤學習於傳統心理學定義中 僅限於刺激與反應之經驗行為而已,由此學習模式所建構之任何機器學習,均只能認 定為經驗之適應模式而已,而較進階的種類,如演化式計算模型,也只是其能透過電 腦強大的運算能力來達成所謂的動態環境下之演化式學習模式,其中演化之特色只是 多考慮了外在環境的變化或內在參數的調整,而整個學習流程卻沒有進ㄧ步修正。這 也可說明,當各人工智慧之原始模型發展針對封閉式環境問題,都會有很好的表現, 但面對非封閉式問題時,卻只能經由大量實驗和透過參數的調整來片面獲取結果而無 法自圓其說。 認知心理學中較完整的認知學習發展是在 1986 年以後,相關研究指出有效率的學 習過程必須包含教育學習,而不再僅有透過試誤學習來達成。以此,本研究發展ㄧ套 修正傳統機器學習之學習流程-雙模式智慧型學習機制。另外,由於 XCS 系統是試誤 學習類之效果較佳及準確率較好的其中之ㄧ模型,透過以 XCS 為基礎加上本研究所提 之學習流程架構,繼而發展出一個有效率之智慧型學習模型(E&R-R model)。 最後,本研究試圖以較複雜的問題來進行實驗模擬,而該問題為運用財務資料以 建立財務預測知識模型,其模式為三種:XCS,R-R XCS 與 E&R-R XCS,透過三種 模型的準確率與最後報酬率之比較來驗證本研究所提出之學習流程的效能。初步驗 證,E&R-R XCS 均較 R-R XCS 和 XCS 之機制有顯著效能提升。 關鍵字:人工智慧,心理學,認知結構,試誤學習,教育學習,智慧型學習模型。. i.
(4) Applying Cognitive Learning to Enhance XCS to Construct a Dual-Mode Learning Mechanism of Knowledge-Education and Machine-Learning — an Example of Knowledge Learning on Finance Prediction Student: Yi-Chang Chen. Advisors: Dr. An-Pin Chen. Institute of Information Management National Chiao Tung University. Abstract From 1956, the learning definitions of Artificial Intelligence and Psychology to human mind/behavior are obviously different. Owing to the rapid development of the computing power, we have potential to enhance the learning mechanism. This work tries to apply the learning process of the cognition structure defined in Cognitive Psychology to enhance or modify the development of AI, of which the learning models are almost based on trial and error style. However, this kind of learning style is definably given to the experience behavior of stimulus and response in Psychology. Thus, the relative AI models based on such style are design as an experience-adaptation system. For better ones, e.g. evolution-base algorithms, they belonged to the system with more powerful computing power to the dynamical environment. Even so, it was considered not only outside environment but also internal parameter tuning. As for the entire learning process, it has never been enhanced. That is, various original AI models are easily to be developed to their own close-form problem. To the unclose-form problems, their distinct results only come from huge amounts of experiments and tuning their model’s parameters. As the result, it is not easy to make clear for the explanation to why or how. The desirable cognitive learning of cognitive psychology is the development that has started since 1986. The relative literatures have pointed out that teaching-base education would increase the learning efficiency, but trial and error style is not sufficient to learning. That is the reason we enhance the AI learning process to develop a dual-perspective learning mechanism. Furthermore, since XCS is a better accuracy model of AI, we have applied it as a basement and involve the enhanced model proposed to develop an intelligence-learning model. ii.
(5) Finally, this work is designed a test of the more complex problem, which is constructing a finance prediction knowledge model. By comparing to the accuracy and accumulative profit of XCS, R-R XCS and E&R-R XCS respectively, the results obtain the obvious outcome. That is, the proposed learning framework has enhanced the original mechanism. Keyword: Artificial Intelligence, Psychology, Cognition Structure, Trial and Error, Teaching-Base Education, Intelligence-Learning Model.. iii.
(6) 誌謝 博碩士生活終於告一段落,在這期間最要感謝的是指導教授 陳安斌老師,在這 幾年間,陳老師對我的指導,不單只有在學業方面,在待人處事上也處處提醒我:不 僅要時時感恩更要廣結善緣,這樣的過程,讓我不僅在學術研究上求得進步,更在生 活觀上得到提升;同時特別感謝諸位口試委員:陳鴻基老師、楊千老師、劉敦仁老師、 林妙聰老師以及鄭景俗老師,對於我的博士論文給予悉心指導;感謝您們提供寶貴的 建議,使得論文能更臻完整。 此外,從小到大,爸爸和媽媽給我無止境的關懷,一直是我求學過程中最大的後 盾,這是我最引以為傲的,對此,心中存有無限感激;大妹和小妹對我的鼓勵與支持, 她倆更是代替我隨伺爸媽身邊盡孝順心,這是我無以回報的;另外,特別值得ㄧ提的 是我那可愛的女朋友,總是會替我分憂解勞,她的聰明伶俐,讓我的研究生活充滿快 樂的點滴。對於這些家人,我深深感謝他們,謹以此論文與他們分享。 最後感謝研究室裡,不管是和我ㄧ起打拼的小組或是 APC LAB 之其他成員,你 們的存在,讓我的研究生活不會感到孤單;乃至於資管所的各位朋友,要感謝的人太 多,無法一一陳述,這些種種我都點滴在心頭,你們都是我生命中不可或缺的一部分, 我永遠感激你們!. 陳怡璋 2005/7/31. iv.
(7) Table of Contents 摘要 .............................................................................................................................................i Abstract.......................................................................................................................................ii 致謝 ...........................................................................................................................................iv Table of Contents........................................................................................................................v List of Tables ............................................................................................................................vii List of Figures..........................................................................................................................viii Chapter 1. Introduction...............................................................................................................1 1.1 Motivation ....................................................................................................................1 1.2 Purpose .........................................................................................................................3 1.3 Research Problem .........................................................................................................4 1.4 Organization .................................................................................................................4 Chapter 2. Literature Review......................................................................................................6 2.1 History of Classifier System.........................................................................................6 2.2 History of Cognitive Psychology .................................................................................9 2.3 Relationship of Cognitive Psychology and Classifier System ...................................13 Chapter 3. “Evolved Learning” of XCS and Learning of Cognition .......................................16 3.1 Introduction ................................................................................................................16 3.2 Evolved Learning .......................................................................................................16 3.2.1 Dynamical Evolved Learning..........................................................................17 3.2.2 Trial & Error Learning.....................................................................................17 3.3 Cognitive Learning .....................................................................................................19 3.3.1 Teaching Learning ...........................................................................................19 3.3.2 Reinforcement-Rehearsal (R-R) Learning.......................................................20 3.4 Information Process Theory .......................................................................................23 3.4.1 Short-Term Memory ........................................................................................24 3.4.2 Long-Term Memory ........................................................................................24 3.4.3 Working Memory.............................................................................................25 3.4.4 Accumulation of Knowledge ...........................................................................26 3.4.5 Summary..........................................................................................................26 3.5 Conceptual Framework ..............................................................................................27 Chapter 4. Education and R-R Model Based on XCS..............................................................31 4.1 XCS ............................................................................................................................32 4.2 R-R Learning Based XCS Model ...............................................................................34 4.3 Education & R-R Based XCS Model .........................................................................36 4.4 Assumption to Education Materials............................................................................38 4.5 Propositions ................................................................................................................39 v.
(8) Chapter 5. Simulation and Comparison of XCS, R-R XCS, and E&R-R XCS .......................42 5.1 Simulation on Finance Prediction ..............................................................................42 5.1.1 Prediction on Global Overnight Effect ............................................................42 5.1.2 Input Factors and Overnight Effect Theory.....................................................43 5.1.3 Prediction Model .............................................................................................44 5.2 Experiments ................................................................................................................46 5.2.1 Experiments .....................................................................................................46 5.2.2 XCS Experiments ............................................................................................50 5.2.3 R-R XCS Experiments ....................................................................................54 5.2.4 E&R-R XCS Experiments ...............................................................................58 5.3 Comparison and Discussions......................................................................................62 5.3.1 Models Self-Comparison.................................................................................62 5.3.2 Models Comparison.........................................................................................63 Chapter 6. Conclusions.............................................................................................................65 6.1 Result and Summary...................................................................................................65 6.2 Future Works ..............................................................................................................66 Reference ..................................................................................................................................67 Appendix A. Relevant XCS Statements ...................................................................................71 Appendix B. Knowledge Population........................................................................................77. vi.
(9) List of Tables Table 1. Arguments of Psychology to Soft Computing Techniques. ........................................12 Table 2. Arguments of Psychology to Classifier Systems. .......................................................15 Table 3. Dual-Mode Learning Model of Education-Dominated and R-R Perspectives...........30 Table 4. Encoding Rule to the Fluctuation of DJi and Twi ......................................................46 Table 5. Listing of Investment Strategies .................................................................................48 Table 6. Table of Predicted Advance-Decline Ratio of Twi Return and its Accuracy Indicator48 Table 7. Summary of XCS Experiments ..................................................................................62 Table 8. Summary of R-R XCS Experiments...........................................................................62 Table 9. Summary of E&R-R XCS Experiments .....................................................................62. vii.
(10) List of Figures. Figure 1. Evolution of Cognition Psychology..........................................................................10 Figure 2. Information Process Theory Proposed by Gagne......................................................24 Figure 3. Education Learning Flow and Reinforcement-Rehearsal Learning Flow.................28 Figure 4. Richard Atkinson and Richard Shiffrin proposed a theoretical model for the flow of information through the human information processor. ...................................................31 Figure 5. Dual perspective learning process of Education and R-R mechanism. ....................32 Figure 6. XCS Procedure..........................................................................................................34 Figure 7. R-R XCS Procedure. .................................................................................................36 Figure 8. E&R-R XCS Procedure. ...........................................................................................38 Figure 9. Theoretical Accuracy of XCS, R-R XCS, and E&R-R XCS. ...................................40 Figure 10. Theoretical-Accumulative Performance of XCS and R-R XCS .............................40 Figure 11. Theoretical-Accumulative Performance of XCS and E&R-R XCS........................41 Figure 12. Overnight Effect Phenomenon................................................................................43 Figure 13. Distribution of Historical Return of (a) DJi and (b) Twi.........................................45 Figure 14. Flow of XCS, R-R XCS and E&R-R XCS Experiments. .......................................47 Figure 15. Testing Data of Taiwan Weight Index from 2004/01 to 2004/09 ............................49 Figure 16. Strategy 1: Accuracy Ratio of XCS ........................................................................50 Figure 17. Strategy 2: Accuracy Ratio of XCS ........................................................................51 Figure 18. Strategy 3: Accuracy Ratio of XCS ........................................................................51 Figure 19. Strategy 1: Accumulative Profit of XCS.................................................................52 Figure 20. Strategy 2: Accumulative Profit of XCS.................................................................52 Figure 21. Strategy 3: Accumulative Profit of XCS.................................................................53 Figure 22. Strategy 1: Accuracy Ratio of R-R XCS.................................................................54 Figure 23. Strategy 2: Accuracy Ratio of R-R XCS.................................................................55 Figure 24. Strategy 3: Accuracy Ratio of R-R XCS.................................................................55 Figure 25. Strategy 1: Accumulative Profit of R-R XCS .........................................................56 Figure 26. Strategy 2: Accumulative Profit of R-R XCS .........................................................56 Figure 27. Strategy 3: Accumulative Profit of R-R XCS .........................................................57 Figure 28. Strategy 1: Accuracy Ratio of E&R-R XCS ...........................................................59 Figure 29. Strategy 2: Accuracy Ratio of E&R-R XCS ...........................................................59 Figure 30. Strategy 3: Accuracy Ratio of E&R-R XCS ...........................................................60 Figure 31. Strategy 1: Accumulative Profit of E&R-R XCS....................................................60 Figure 32. Strategy 2: Accumulative Profit of E&R-R XCS....................................................61 Figure 33. Strategy 3: Accumulative Profit of E&R-R XCS....................................................61 viii.
(11) Chapter 1. Introduction. 1.1 Motivation Traditionally, Artificial Intelligence, according to the definition of Computer Science, works as helpful machines to find solutions to complex problems in a more human-like fashion [1]. This generally involves adopted characteristics from human intelligence, and it applies them as algorithms in a computer friendly way. A more or less flexible or efficient approach can be taken depending on the requirements established, which influences how artificial the intelligent behavior appears. Those researches, for example: Neural Network, Fuzzy Approach, Genetic Algorithm, and so on, all focus on Soft Computing. Of course, XCS (Extend Classifier System) is also a hybrid approach with high performance to the accuracy and the rule evolution on the prediction application. However, up to now, the Artificial Intelligence Techniques based on Soft Computing have all involved the concept, trial and error method or stimulus-response method even the series of evolution approaches [2,3], to construct their learning models. For this aspect, if possible, this example, a Chinese idiomatic phrase-”An Illusory Snake in a Goblet”, is taken into consideration as an input-output pattern to training the learning model. The models are formed for sure. It is actually a wrong model trained by a bad experience. Besides, the parameters of those training models are exactly affected by the input dataset, especially the large difference of the training inputs and testing ones. Usually, in many researches it is chosen the high relation between the input and output datasets or given the strong assumption which is the inputs and outputs are relevant. Thus, a subjective black-box view and the tuning view are easily concluded [4]. The other sub-domain, Expert System, which’s primary goal is to make expertise 1.
(12) available to decision makers and technicians who need answers quickly. There is never enough expertise to go around -- certainly it is not always available at the right place in the right time. The same systems in-depth knowledge of specific subjects can assist supervisors and managers with situation assessment and long-range planning. These knowledge-based applications of artificial intelligence have enhanced productivity in business, science, engineering, and even the military. Although, the development of those expert systems is the view of anti-extreme to construct domain knowledge first but, for the reason, they are lack of the flexibility and the adaption. In fact, each new deployment of an expert system yields valuable data for what works in which context, thus fueling the AI research that provides even better applications. Many researches, no matter Soft Computing techniques or Expert Systems try to consider into the human-like thinking way to make the simulation. But, from classic psychology, the human-mind researches are the researches to the human-behavior. Since Plato, Psychology is an unfathomable philosophy and those advanced AI researchers should concern this perfect development of Human Psychology, from simple to complex and from single factor to multiple ones. However, the traditional AI techniques are seldom focused on the high level of human-mind process and just paid attentions to the learning definition from the Empricalism Psychology. According to the development of Modern Psychology, the core of Psychology has been already transferred Empricalism-base into Information Process Theory of Human-Mind, Cognitive Psychology-base. As for the knowledge and the model construction, the teaching-base aspect has been involved as well to the learning process. Based on the aspect, this work tries to enhance the learning process of traditional AI techniques whose cognitive scotomas of learning definition, and it develops the novel learning model, involving the concept of Cognitive Psychology, which is utilized the high accuracy-prediction XCS model as the construction basement.. 2.
(13) 1.2 Purpose Among learning artificial intelligence techniques, no matter neural network, fuzzy approaches, or any hybrid methods, all the models are formed by trial and error learning way, the traditional definition of learning [1]. It is practicable to be implemented that those models are utilized to a close-form problem. As for the others to unclose-form problems, however, it is critical the set of their relative input and output pairs needs to be modified. The datasets used to train or test should be all verified first as well, which is a boring work to the model designers. Besides, the relative problems of those evolution artificial intelligence techniques are also faced to my pre-statement. It is more significant to concern the proper datasets as inputs effects the model construction. By Darwin’s Evolution Theory, Natural-Selection is easily to be concluded for the all organisms. The detailed steps could be realized that each obvious verified evolution result is always caused by the right things, the key factors, and the certain environment at the critical time. It is definitely not the random result. Take human evolution for instance, judged from the biotic evolution history of the earth – from the mitochondria, the cell, the microorganism, the multi-cell organism, …, the pithecanthrope, to Human, who dare to assure Human as the primate animal, still would own respectively two hands and two feet, each five fingers, if the history of the earth reshuffles?. That explains the reason, of which the dimension to solve problems could not be too complex, is that the training samples are not always sufficient to construct the model. Nevertheless, much Knowledge discovery, Theory verification and Theorem definition are aggregated and not disregarded. They are all continually historical accumulated. That is also the reason that the civilization is enhanced, the culture is accumulated, and knowledge is transmitted. Either the voluntary learning or the passive learning through education is the key cores in each process. Following the previous concept, moreover, the hybrid approach, XCS [5], has already been verified its prediction accuracy and its ability to dynamical 3.
(14) environment and it becomes the foundation of this work to construct the knowledge learning model. The above two assumptions/pre-statements are taken into consideration to develop the efficient knowledge learning model of the self-learning and the passive-learning. The methodologies are applying XCS with the reinforcement learning ability and involving the Human education [6] characteristic of Cognitive Psychology. Furthermore, it is the purpose to develop the high efficient learning model with the high accuracy knowledge accumulation is its purpose. The major contribution of this work is the proposed architecture. Once, the more accuracy ability of AI Techniques invented could be substituted for XCS and more performance would be more efficient.. 1.3 Research Problem The research issue will be arranged to develop the efficient knowledge learning model. First, the learning definition would be concluded from traditional AI, especially the classifier system. Second, in this work we would try to survey the psychology, thousands year of its development, as the basement to analyze the development of AI and the learning of human behavior. Moreover, this work focuses on Modern Psychology, Cognitive Psychology, to collect and induce and its learning concept to develop an enhanced model which increases the training process and the knowledge output. As for the design of the simulation, the traditional training/learning process of XCS model would be respectively compared to the proposed learning model and the education-learning proposed model. Finally, the performance would be verified.. 1.4 Organization The rest of this dissertation is organized as follows. In Chapter 2 we review the related work on Classifier System, Cognitive Psychology, and the Relationship of Cognitive Psychology and Classifier System. In Chapter 3, the cognitive learning from the evolved 4.
(15) learning is distinguished and the definition of memory from Cognitive Psychology is described. In Chapter 4 it presents the dual-mode learning mechanism by education (E) learning and reinforcement-rehearsal (R-R) learning based on XCS, which contains the description of XCS, R-R XCS, and E&R-R XCS. Chapter 5 compares the experiments with the three learning model. Nevertheless, the design of finance prediction simulation would be detailed first. Conclusions and future work are made in the final Chapter 6.. 5.
(16) Chapter 2. Literature Review. 2.1 History of Classifier System Learning classifier systems are a machine learning paradigm introduced by John H. Holland. They first appeared in 1978 in the paper “Cognitive Systems Based on Adaptive Algorithms” by Holland and Reitman [7]. However, before that, Holland [8] foreshadowed classifier systems in 1971. In learning classifier systems an agent learns through experiments to perform a certain task by interacting with a partially unknown environment, using rewards and other feedback to effect an internal evolutionary process which forms the rule-based model of the world. The agent senses the environment through its detectors; based on its current sensations and its past experience, the agent selects an action sent to the effectors in order to be performed in the environment. Depending on the effects of the agent's action, the agent occasionally receives a reward. The agent's general goal is to obtain as much reward as possible from the environment. In his pioneer work, Holland combined two ideas which later became key topics of the research in machine learning. The first idea was that Darwinian Theory of the survival of the fittest could be used to trigger the adaptation to the artificial system to an unknown environment. This idea later became the basis for many important research areas, such as Evolutionary Computation. The second idea was that an agent could learn to perform a task just by trying to maximize the rewards it receives from an unknown environment. This model of learning through “trial and error” interactions has been formalized and developed in the area of reinforcement learning, which is now a major branch of machine learning research. Learning classifier systems have been wielded all-around through out more than twenty years. In these two decades they have receiving the more and more attention by. 6.
(17) many researchers from many areas. In Holland's learning classifier system, there were a number of well-noted problems that prevented the system from achieving satisfactory performance in some cases. In 1987 Wilson [9] introduced a new type of “one-step” classifier system. Wilson showed that BOOLE could learn multiple disjunctive concepts faster than neural networks. Separately, Booker [10] introduced GOFER-1, a new type of classifier system that. In GOFER-1 the classifiers fitness is a function of both payoff and non-payoff information, and the genetic algorithm works in environmental niches instead of in the whole population. Wilson [11] observed that the architecture of learning classifier systems is too complex to permit carefully, revealing studies of the learning capabilities of these systems. Accordingly he simplified the original framework and then introduced ZCS, a zeroth level classifier system. After that, optimal performance in different applications was finally reported in 1995 when Wilson [5] invented the XCS classifier system. While XCS maintains Holland's essential ideas about classifier systems, it differs pretty much from all the previous architectures. First in XCS Q-learning is used to distribute the reward to classifiers, instead of a bucket brigade algorithm. Second, in XCS the genetic algorithm acts in environmental niches instead of on the whole population, as it does in the work of Booker on GOFER-1 [10]. The most important of all, in XCS the fitness of classifiers is based on the accuracy of classifier predictions instead of the prediction itself, a solution partially anticipated in the works of Frey and Slate [12] and Booker [10]. Wilson [5, 13] showed that by using classifier accuracy as the fitness of the genetic algorithm, XCS is able to evolve classifiers that are (i) accurate. They give an accurate prediction of the expected reward, and (ii) maximally general. They match as many situations as possible without being overgeneral. Anticipatory Classifier System (ACS), introduced by Stolzmann [14], differs greatly from other LCSs in that CS learns not only how to perform a certain task, but also learns an 7.
(18) internal model of the dynamics of the environment or task. In ACS classifiers, there are not simple condition-action rules but they are extended by an effect part. The effect-part of a classifier is used to anticipate the environmental state which results from the execution of the classifier action. The model of the environment can be learned latently, that is it learned without any environmental reward, because the fitness of the classifiers depends on the accuracy of the anticipation. The classifier fitness is high if the next state is anticipated correctly while it is low if the anticipation is wrong. Besides genetic algorithms an Anticipatory Learning Process (ALP) is utilized for rule discovery which directly learns from the changes in the environment. ALP is a further development of a psychological learning theory, called anticipatory behavioral control [14]. ACS forms explicit condition-action-effect classifiers with a generalization capability in the classifier conditions. This leads to an internal model of the environment which consists of a minimal set of classifiers. The internal model can be used in many applications: (i) for mental acting and look ahead planning to improve learning, (ii) for action planning and goal directed planning in the absence of environmental reward, and many more. LCSs have been applied in many domains [15]. However, most of the results reported fall into three main areas: autonomous robotics; knowledge discovery; and computational economics. For a good instance, Holmes’ EpiCS [16] is an LCS specialized for classification and knowledge discovery tasks. It was developed from NEWBOOLE to meet the demands of epidemiologic data. EpiCS’s distinctive features include: (i) techniques for controlling over- and under-generalization of data, (ii) the use of differential negative reinforcement of false positive and false negative errors in classification, and (iii) a methodology for determining risk as a measure of classification. All of these features have led to the successful usage of EpiCS in knowledge discovery applications to actual clinical databases of various sizes and levels of complexity. EpiCS was able to (i) derive models that identified features that were associated with outcomes such as appropriate child 8.
(19) restraint in automobiles, (ii) occupational cancer (simulations), and (iii) head injury to children involved in automobile crashes (see [17] for an overview). Therefore, EpiCS appears to be a successful approach to apply evolutionary computation to the realm of knowledge discovery in databases. The development of new LCS models [18], successful in many domains, has led to a resurgence of this area during recent years. Overall, the recent results represent probably the most significant advances in LCS research presented so far. However, most work still need to be done; there are many interesting research directions to be explored, and many open challenges. Besides, owing to the origin of LCS is described as a cognitive system by Holland, next will be discussed with cognitive psychology.. 2.2 History of Cognitive Psychology Cognitive Psychology is concerned with advances in the studies of memory, language processing, perception, problem solving, and thinking. However, to explore the beginning of Cognitive Psychology should be traced to the field of psychology whose history diagram was shown as Figure 1. The earliest roots of psychology would be divided into two different approaches to understand the human mind: philosophy and physiology. The pre-evidences are the two Greek philosophers Plato (ca. 428-348 B.C.) and his student Aristotle (384-322 B.C.) who has profoundly affected modern thinking in psychology and in many other fields. Both of them are the originators of rationalist and empiricist. A rationalist is one who believes that there is a route to knowledge is through logical analysis. In contrast, Aristotle’s approach is that of an empiricist, the one who believes that we acquire knowledge via empirical evidence, obtained through experience and observation. In Aristotle’s view, then, it leads directly to empirical investigations of psychology, whereas Plato’s view foreshadows the various uses of reasoning in theory development. But, most psychologists today seek a synthesis of the two: They all base empirical observations 9.
(20) on theory but in turn of using these observations to revise their theories. To elaborate on Aristotle’s ideas, Kemp (1996, 2000) [19] attempted to locate cognitive processes in the brain and to prove to have little to do with our current understanding of the brain. Furthermore, The German philosopher Immanuel Kant (1724-1804) [20] began the discussing empricalism versus rationalism. His impact on philosophy interacted with the nineteenth-century scientific exploration of the body and how it works to produce profound influences the eventual establishment of psychology as a discipline in the 1800s. Root. Psychology. Plato, ca. 428-348 B.C.. Philoshiphy Psychology. Weber, 1834 Fechner, 1860 Maslow, 1950. Rationalism. Science Psychology. Wunld, 1879 Aristotle, 348-322 B.C.. Structurelism. Empiricalism. Biological Psychology. Darwin, 1859. Wertneimer, 1912. Gestalt Anti S-R. Pavlov, 1906 Watson, 1913 Skinner, 1938. Functionalism Changing & Dynamic. S-R. Behaviorism. Freud, 1900. Psychoanalysis Lashley, 1929 Hebb, 1949. Humanisticism. Piaget, 1985 Kohler, 1917. Cognitivism Information process theory Figure 1. Evolution of Cognition Psychology Wilhelm Maximilian Wundt was a German physiologist and Psychologist who made Psychology a field of its own. He was the first person in history to be called a. 10.
(21) “psychologist,” as well as the first person to teach a course in Physiological Psychology at Heidelberg in 1867. Wundt established psychology as a unique branch of science with its own questions and methods. Wundt was the first person to take all of the nineteenth century’s sprouting of the new psychology onto the old and creating his new science, and published a book on physiological psychology. The form of psychology Wundt called scientific metaphysics. This form of psychology would be used to integrate the empirical work in the lab with other scientific findings, reviewed by Piaget [6]. The philosophical and psychological developments lead to the emergence of cognitive psychology. Developments in other sub-fields also contributed to the development of cognitivism and modern psychology. Karl Spencer Lashley (1890-1958) [21, 22] studied topics not easily explained by simple conditioning, and to embrace methods other than the experimental manipulation of environment contingencies (Gardner, 1985). Lashley was deeply interested in neuroanatomy (the study of the structures of the brain) and in how the organization of the brain governs human activity. Lashley brashly challenged the behaviorist view that the human brain is a passive organ merely responding to environmental contingencies outside the individual; instead, he considered the brain an active and dynamic organizer of behavior. Donald Hebb (1949) [22, 23] was the first psychologist to provide a detailed, testable theory of how the brain could support cognitive processes. His influential work provides a strong foundation for some of the current trends in cognitive psychology. Behaviorists did not jump at the opportunity to agree with theorists like Lashley and Hebb. They thought that psychology should be the science of the behavior analysis but Human mind. From Watson [24] to Skinner [25], they applied their experimental analysis of behavior to almost everything, from learning to problem solving and even to the control of behavior in society. The other such as, Functionalism, was a major paradigm shift in the history of American psychology. As an outgrowth of Darwin’s evolutionary theory, the functionalist approach focused on the examination of the function 11.
(22) and purpose of mind and behavior. Rather than the structures of the mind, functionalism was interested in mental processes and their relation to behavior. William James, a functionalism, became known to influence the psychology. The following, G. Stanley Hall, Mary Calkins, and Edward Thorndike are spreading functionalist psychology as well. As for Gestalt psychology, it is founder is Max Wertheimer. Those psychologists started to focus on “pattern” from “Gestalt”, “Form”, and “Configuration”. They declared that Behavior is equal to the function of Human and Environment. Each pattern is sensitive to each case respectively. As this description, the definition of behavior is not purely only a set of “Stimulus-Response”. For these instances above, they all were the emergences of cognitive psychology. Generally, cognitive psychology is a science of the research of human cognitive process. The Switzerland philosopher Jean Piaget, originally a biologist, is now best remembered for his work on the development of cognition. Piaget (1985) [26] suggested that learning process is iterative, in which new information is shaped to fit with the learner's existing knowledge, and existing knowledge is itself modified to accommodate the new information. Table 1. Arguments of Psychology to Soft Computing Techniques. Psychology Branch Behaviorism[24,25]. Specific Arguments. Soft Computing Branch. Stimulus-Response (S-R). AI-based learning[1,2,27]. Neurology, and Brain Theory. Neural Network[27]. Darwin-Science. Natural Selection, Theory of. Genetic Algorithm,. Psychology, [James]. Evolution. Genetic Programming[32]. Biological Psychology[21,22,23]. Involve “Human” Factor and Anti Gestalt[6]. None S-R. 12.
(23) To sum up the statements, the roots of the cognitive movement are extremely varied: It includes gestalt psychology, behaviorism, even humanism; it includes thinkers from linguistics, neuroscience, philosophy, and engineering; and it especially involves specialists in computer technology and the field of artificial intelligence. Cognitive psychology is far more sophisticated and philosophical than behaviorism. It does, of course, have the tremendous advantage of being tied to the most rapidly developing technology we have ever seen -- the computer. But more and more people saw AI as ultimately being a good model for human beings, and they are confused about cognitive psychology and other sub-psychology. For the reason, to develop the new human-thinking model to aggregate knowledge should be understood the psychology theory first even cognitive psychology. After all, the history of psychology is more continuous and complete for a long time than AI techniques. In Table 1, this work tries to summary some relationship about sub-psychology to soft computing.. 2.3 Relationship of Cognitive Psychology and Classifier System In 1956 John McCarthy regarded as the father of AI, organized a conference to draw the talent and expertise of others interested in machine intelligence for a month of brainstorming. He invited them to Vermont for “The Dartmouth summer research project on artificial intelligence.” From that point on, because of McCarthy, the field would be known as Artificial intelligence. Artificial Intelligence (AI) belongs to the area of computer science focusing on creating machines that can engage on behaviors that humans consider intelligent. Today with the advent of the computer and 60 years of research into AI programming techniques, the dream of smart machines is becoming into reality, which is concluded from [27]. Learning Classifier Systems (LCSs) are a machine learning paradigm introduced by Holland (1986) [28], also the father of genetic algorithms. Before that, Holland and Reitman 13.
(24) (1978) [29] made their first appearance in the paper “Cognitive Systems Based on Adaptive Algorithms”. While there was still considerable research in the 1980s, the field began to wane at the end of the decade. In the early 1990s, learning classifier systems seemed too complicated to be studied, with few successful applications reported. In the mid 1990s the field appeared almost at a dead end. But, during the last five years, new enhanced models have been developed and new applications have been presented which caused a great resurgence of this area. No matter the father of AI, McCarthy or the father of LCSs, Holland, both of them led the development of AI techniques and LCSs respectively. They caused the confusing definition of learning in various AI researches. Those researches all emphasized that the learning process of AI techniques is cognition. Therefore, AI researches all were developed the learning approaches that own the cognitive concept. Actually, their cognitive concept only presents the trial and error learning. It deserves to be mentioned that McCarthy [30, 31] has ever tried to stand at philosophy or psychology to redefine the learning and knowledge representation and taken seriously the idea of actually making an intelligent machine. Furthermore, McCarthy went on to the notions of metaphysically and epistemologically adequate representations of the world and then to an explanation of can, causes, and knows in terms of a representation of the world. Besides, he also reviewed the work in philosophical logic in relation to problems of artificial intelligence and a discussion of his previous efforts to program “general intelligence” from the point of view of this paper. Such as the above description, McCarthy at least knew that artificial intelligence should be enhanced by philosophy at that time. Until Holland, cognitive system was mentioned the term, cognition, but not sufficient by the cognitive psychology. Maybe it is the reason that the concept of cognitive system was implemented and named to learning classifier systems (LCSs’s) by Holland [28]. However, cognitive psychology was brought into vogue after 1985 by Piaget [26]. And 14.
(25) more cognitive models would be invented after Piaget. Nevertheless, few learning classifier systems focused on them and just enhanced the original Holland’s one. In spite of the development of cognitive psychology, those following LCSs never refocused on the cognition definition, and the relative LCSs recognized their models owning the “cognitive” ability after Holland [28] (1978), for instance, ZCS or XCS and so on. Thereby, this work is given the strong suspicion that LCSs are not sufficient to the Cognition. That is, Table 2 additionally shows the description of the classifier systems for what aspects matching to the psychology by the suspicion. Without surveying the relative cognitive studies and reconcentrating the cognition definition and cognitive model, the novel cognitive system is not easily to develop. Especially, when the cognitive psychology develops based on the thousands years of the psychology history, and it has already mimicked the human mind by several approaches to discovery the cognition process of human and knowledge aggregation. Table 2. Arguments of Psychology to Classifier Systems. Psychology Branch. Specific Arguments. Soft Computing Branch. LCSs don’t have sufficient functions to the Cognition Mechanism, although it is based on solving the changeable issues in. Classifier Systems[this. Functionalism the dynamical environment. That is just satisfied to the Functionalism, but even Gestalt without “Human”.. 15. work].
(26) Chapter 3. “Evolved Learning” of XCS and Learning of Cognition. 3.1 Introduction Owing to the ambiguous of these two learning definitions, the evolved learning and the cognition learning are verified in different period. Besides, various kinds of researchers, such as biologists and philosophers gave the different definitions. Thus, identifying the learning definition would be the main job at the first job, and these two kinds of learning would be detailed next. Then, this work will combine their advantages to develop a high performance knowledge-learning framework that would be discussed first.. 3.2 Evolved Learning Traditional Evolved Learning is derived from Darwin’s Evolution Theory which is the widely held notion that all life is related and has descended from a common ancestor: Complex creatures evolve from more simplistic ancestors naturally time over time. In a nutshell, as random genetic mutations occur within an organism's genetic code, the beneficial mutations are preserved because they aid survival -- a process known as “Natural Selection.” These beneficial mutations are passed on to the next generation. Over time, beneficial mutations accumulate and the result is an entirely different organism. For this aspect, the evolved learning is easily described by Darwin. However, the learning result of this mechanism is sure, and optimal accuracy is verified. But the results would not be evolved the same by different times in insufficient samplings. In other words, the optimal results might be the certain case under the critical opportunity or effected by the certain 16.
(27) factors in a specific environment. 3.2.1 Dynamical Evolved Learning Genetic algorithms are based on a biological metaphor: They view learning as a competition among a population of evolving candidate problem solutions. A “fitness” function evaluates each solution to decide whether it will contribute to the next generation of solutions. Then, through operations analogous to gene transfer in sexual reproduction, the algorithm creates a new population of candidate solutions. John Holland's pioneering Adaptation in Natural and Artificial Systems [32] (1975) described how an analog of the evolutionary process can be applied to solving mathematical problems and engineering optimization problems using what is now called the genetic algorithm (GA). Holland had two aims: to improve the understanding of natural adaptation process, and to design artificial systems having properties similar to natural systems. The basic idea is as follow: the genetic pool of a given population potentially contains the solution, or a better solution, to a given adaptive problem. This solution is not “active” because the genetic combination on which it relies is split between several subjects. Only the association of different genomes can lead to the solution. No subject has such a genome, but during reproduction and crossover, new genetic combination occurs and, finally, a subject can inherit a “good gene” from both parents. Holland method is especially effective because he not only considered the role of mutation (mutations seldom improve the algorithms), but he also utilized genetic recombination, (crossover): these recombinations, the crossovers of partial solutions greatly improve the capability of the algorithm to approach, and eventually find, the optimum. 3.2.2 Trial & Error Learning Edward L. Thorndike (1943) [33] claimed that “A good simple definition or 17.
(28) description of a man's mind is that it is his connection system, adapting the responses of thought, feeling, and action that he makes to the situation he meets.” He worked on educational psychology and the psychology of animal learning. As a result of studying animal intelligence, he formulated his famous “law of effect”, which states that a given behavior is learned by trial-and-error, and is more likely to occur if its consequences are satisfying. Thorndike's early experiments (1898 - 1911)[34] involved a hungry cat put in box that contains a concealed mechanism operated by a latch learning involves the goal of the cat manipulating the latch, opening the door, finding food, and eating initial random behavior is followed by the cat “catching on” and quickly opening the door. Thorndike maintained that, in combination with the “law of exercise”, the notion that associations are strengthen by use and weakened with disuse, and the concept of instinct, the law of effect could be explained to all of human behavior in terms of the development of myriads of stimulus-response associations. Briefly it is worth briefly comparing trial and error learning with classical conditioning. In classical conditioning a neutral stimulus becomes association with part of a reflex. In trial and error learning no reflex is involved. A reinforcing or punishing event (a type of stimulus) alters the strength of association between a neutral stimulus and quite arbitrary response. The response is not to any part of a reflex. The behaviorist points out that human behavior could be explained entirely in terms of reflexes, stimulus-response associations, and the effects of reinforcers upon them entirely excluding 'mental' terms like desires, goals and so on was taken up by John Broadhus Watson[35]. As for the reinforcement learning of XCS [36], its major thread concerns learning by trial and error and started in the psychology of animal learning. This thread runs through some of the earliest work in artificial intelligence and led to the revival of reinforcement learning in the early 1980s. This thread began in psychology, where “reinforcement” theories of learning were common. Perhaps the only first person to succinctly express the 18.
(29) essence of trial-and-error learning was just Edward Thorndike [34]. This essence was taken to be the idea that actions followed by good or bad outcomes have their tendency to be re-selected altered accordingly. Additionally, in spite of the original development of each AI or the application of it, they more or less involved the trial & error method to invent their model. For instance, the most basic method of training a neural network is trial and error. Change the weighting of a random link by a random amount if the network isn't behaving the way it should. Undo the change and make a different one if the accuracy of the network declines. It takes time, but the trial and error method does produce results.. 3.3 Cognitive Learning Cognitive psychology is a theoretical perspective that focuses on the realms of human perception, thought, and memory. It portrays learners as active processors of information--a metaphor borrowed from the computer world--and assigns critical roles to the knowledge and perspective students bring to their learning. What learners do to enrich information, in the view of cognitive psychology, determines the level of understanding of that they ultimately achieve. Cognition is defined as “the mental process or faculty of knowing.” To help the students reach a cognitive state about a certain subject should be one of the goals of both teaching and learning. Thus, the below discussions were the teaching learning and the rehearsal learning. 3.3.1 Teaching Learning As articulated by Piaget (1969)[37], students learn better when they can discover knowledge through the way of inquiry and experimentation instead of acquiring facts presented by a teacher in class. Since the learner is portrayed as an active processor who explores, discovers, reflects, and constructs knowledge, the trend to teach from this 19.
(30) perspective is known as the constructivist movement in education. As Bruning (1995)[38] explains, “The aim of teaching, from a constructivist perspective, is not so much to transmit information, but rather to encourage knowledge formation and development of metacognitive processes for judging, organizing, and acquiring new information.” Several theorists have embellished this theme. Rumelhart (1981)[39], following Piaget, introduced the notion of schemata, which are mental frameworks for comprehension that function as scaffolding for organizing experience. At first, the teacher provides instructional scaffolding that helps the student construct knowledge. Gradually, the teacher provides less scaffolding until the student is able to construct knowledge independently. Recently, there has been some interests in developing formal models of teaching [40, 41, 42, 43, and 44] through which we can develop a better understanding of how a teacher can most effectively speed up the training process. Although, the formal models of teaching that have been introduced in the learning theory community is that they place stringent restrictions on the learner to ensure that the teacher is not just providing the learner with an encoding of the target. In particular, the teaching models allow the teacher to present a set of examples for which only the target function is consistent. Thus, teaching under these models is made unnecessarily difficult since the problem reduces to teaching an obstinate learner that tries as hard as possible not to learn while always outputting a hypothesis consistent with all previous examples. In other words, teaching learning is necessary to a learner to reduce the complexity learn process. 3.3.2 Reinforcement-Rehearsal (R-R) Learning Reinforcement Learning There are several kinds of learning theories from behaviorists. You may be familiar with “conditioned response theory” developed by Pavlov 1903, whereby a response that already occurs in the presence of one stimulus can be “conditioned” to occur following a 20.
(31) different stimulus. This learning theory is very important for emotional learning, but has little relevance to most learning of invariant tasks. Far more relevant is “reinforcement theory,” first developed by E. L. Thorndike (1913) [33] and further developed by B.F. Skinner (1956)[24] and others. In reinforcement theory, an invariant task is viewed as a “response” and is learned when it becomes “associated” with an appropriate stimulus. For example, “3.14” is a response that should become associated with “Pi”. This learning process occurs whenever “reinforcement” follows the response. For example, each time a learner responds with “3.14”, a reinforcer such as “Right!” or “Good!” or even just a smile with a nod will increase the probability of the learner responding the same way in the future. With sufficient repetition of these stimulus-response-reinforcement events, the response will come to occur automatically in the presence of the stimulus. Also, the learning classifier system is a machine learning system with close links to reinforcement learning and genetic algorithms. LCS consists of a population of binary rules on which a genetic algorithm altered and selected the best rules. Instead of a using fitness function, rule utility is decided by a reinforcement learning technique. Rehearsal Learning Besides the reinforcement learning, rehearsal learning differs from it. A rehearsal strategy is used by the repeated practice of information to learn it. When a student receives the specific information that needs to be learned, such as a list, often he will attempt to memorize the information by repeating it over and over. He may read the words out loud, or he may sub vocalize the information (read it in his own mind). The repeated practice increases the student's familiarity with the information. For many people, the learning of our social security number, our telephone number, or the items we want to pick up at the grocery store prompts us to use a rehearsal strategy. This strategy originally documented by Belmont and Butterfield (1971) [45] examines how regular review and recall techniques aid the transfer of information into LTM. Buzan 21.
(32) [46] goes on to propose a pattern that the rehearsal strategy should follow. By monitoring recall rates during, and immediately after learning has taken place and at timed intervals thereafter, Buzan concludes that “The first review should take place about 10 minutes after a one hour learning period and should itself take 5 minutes. This will keep recall high for approximately one day when the next review should take place, this time for a period of 2 to 4 minutes. After this, recall will probably be retained for approximately a week, when another 2 minutes review can be completed followed by a further review after about one month. After this time the knowledge will be lodged in LTM”. Rehearsal strategies can be used to learn relatively brief amounts of information, and is good for learning “foundation information” or “correct information”. Foundation and correct information is necessary to be learned before more complex learning can take place. If you are using rehearsal to teach information that contributes to a larger concept or skill, keep in mind that lots of practice may be required for the students to learn the information to a level of automaticity. After initial learning takes place, you will need to review many times to ensure that the students have retained the information. We have all memorized information that we have promptly forgotten when we stopped rehearsing. For example, it is more concerning that “3.14” is a “True” response that should become associated with “Pi”. This learning process occurs whenever “Rehearsal” follows the response. Contrary to the “Reinforcement”, “314” is a “False” response that should become associated with “Pi”. This learning process occurs whenever “Reinforcement” follows the response. It is still practicable in the reinforcement learning process. In spite of the mechanism of LCSs, it has the reward ability similar to the rehearsal learning as well. The truth of the rehearsal learning cognition is that teachers take the foundation or correct information to educate the students and students practice the information by themselves. The proper correct information or knowledge is worth to do the rehearsal. That is the difference of reinforcement and rehearsal. Furthermore, the fullness 22.
(33) explanation of information process theory, the narrow terms of cognitive psychology would be detailed next.. 3.4 Information Process Theory There are at least two major kinds of cognitive theory relevant to learning invariant tasks:. information-processing. theory. and. schema. theory.. According. to. the. information-processing model of learning (see Figure 2), there is a series of stages by which new information is learned (Gagne, 1985) [47]. Information is received by receptors (such as the eyes and ears), from which it is passed to the sensory register where all of it is held, but for only a few hundredths of a second. At this point of view, selective perception acts as a filter which causes some aspects of the information to be ignored and others to be attended to. For example, the ears (receptors) receive the sounds comprising “Pi equals 3.14,” along with various other background sounds, and all those sounds are passed on to the sensory register in the brain. Then through the selective perception process, some of the information (hopefully the “Pi equals 3.14”) is attended to the part. That information which is attended to is transformed and passed on to short-term memory, which can only contain a few items of information at a time (depending on their complexity). For instance, if “Pi equals 3.14” is attended to, it is then passed on to short-term memory, where it might be said to “echo” for a few seconds, and the echoing can be prolonged through rehearsal.” Items can persist in short-term memory for up to about 20 seconds without rehearsal, but with constant rehearsal they can be retained indefinitely. Finally, the information may be passed on to long-term memory. This process is called encoding to memorize. For example, if appropriate encoding processes are exercised to link the “Pi equals 3.14” with prior knowledge, then the information is passed on to long-term memory. In the traditional model of human memory (Atkinson and Shiffrin, 1968 [48]; Waugh and D. A. Norman, 1968 [49]), immediate free recall yields items directly retrieved 23.
(34) from a temporary short-term memory (STM) and items retrieved by retrieval cues from a more durable storage in long-term memory (LTM).. Feedback attention Stimilus. Sensor. STM. filter. Limitedcapacity channel. organizing elaboration. LTM. Feedback Figure 2. Information Process Theory proposed by Gagne [47], [50]. 3.4.1 Short-Term Memory Short-term memory (STM) lasts from a few seconds to a minute; the exact amount of time may vary somewhat. For instance, when you are trying to recall a telephone number that was heard a few seconds earlier, the name of a person who has just been introduced, or the substance of the remarks just made by a teacher in class, you are calling on short-term memory. STM is assumed to have a limited capacity (G. A. Miller, 1956)[51], and when attention is diverted to another demanding task, information originally stored in STM becomes unavailable. 3.4.2 Long-Term Memory By contrast, long-term memory (LTM) lasts from a minute or so to weeks or even years. From long-term memory you can recall general information, which is valuable information, usually called to knowledge, about the world that you learned on previous occasions, memory for specific past experiences, specific lectures previously learned, and the like. The storage capacity of LTM is assumed to be vast and much more durable than that of STM. Storage in LTM is assumed to be primarily associative, relating different items to one another and relating items to attributes of the current situation (current context). The 24.
(35) time required for storage of a new retrievable memory trace in LTM has been estimated to be relatively long--about ten seconds (Simon, 1973)[52]. 3.4.3 Working Memory In addition to LTM and STM, models of working memory (WM) have focused on the availability of information in STM which has limited to the capacity. No model of WM can reasonably allow greater working capacity during performance of a specific task than the maximal capacity of working memory measured in a pure memory task. That is, the capacity of WM must be much less than STM (G. A. Miller, 1956). Such a severe limit on WM might seem far too restrictive to allow for human performance levels. Newell and Simon (1972) [53] proposed a production-system architecture for cognitive processes that has influenced most subsequent efforts to build models and theories. In this architecture the conditions of a large number of productions (condition-action pairs) are matched against the currently active elements (working memory). Such as Anderson's (1983) [54] ACT*, WM is the transiently activated portion of LTM. The limits on the number of elements in WM are not determined by a fixed number but rather by the amount of available activation. In his work on building ACT* models of cognitive processing Anderson found that WM can sometimes contain over 20 units at one time. To reconcile such a large capacity of WM with the much smaller capacity of STM, Anderson argued as follows: The activation of elements decays very rapidly. For this reason the number of units that can be actively maintained long enough to be included in immediate recall is much less than all of the information activated at the start of recall. Most investigators argue, however, that the capacity of WM must be far greater than the capacity of traditional STM (Newell, 1990 [55]). In spite of the discussion of limited capacity of WM, the function of WM would be flexible to a branch from LTM or STM. 25.
(36) 3.4.4 Accumulation of Knowledge In the “Knowledge Society”, there are two constants – continuous change and increasing volumes of information. Knowledge and skill currency can only be maintained in an era of such rapid change through active engagement in lifelong learning and the deployment of effective learning strategies. This subject draws upon relevant recent research and theory in the area of cognitive psychology to provide the knowledge and skills necessary to move beyond rhetoric to effective educational practice. Furthermore, cognitive psychology defined Cognition as the acquisition of knowledge [56]. In the others, cognitive psychology defined Knowledge as the storage and organization of information in memory [57]. The awareness of that knowledge more than heuristics or search strategies is at the core of much human cognitive functioning has also led applied researchers to pay much more attention to memory based knowledge structures. Accuracy of the model outcome is determined considerable by the amount and nature of the knowledge available and how effectively a model retrieves this knowledge from LTM. Upon the research of human information processing theory, understanding the mechanism of memory is necessary to how the knowledge is stored. 3.4.5 Summary Using a computer as a metaphor for memory, the short-term phase is RAM (highly volatile and easily lost when some others else are entered), but long-term memory is such as a hard drive or diskette (the information is stored there even after the machine is turned off). This metaphor is especially helpful because a computer knows the address of each bit of information because of the manner information is entered. It is essential that information placed into a student's long-term memory be linked in a way that the student can retrieve it 26.
(37) later. The teacher who should understand the relationship between memory and retrieval can lay out a lesson plan to assist the student in the process and enhance his learning. As the pre-statement portrayed, while rehearsal is important to short-term memory, it can also be used to transfer information to long-term. Elaborating or making material memorable will also enhance the student's learning process. The effective teacher will elaborate and rehearse material so that the student can remember the information more easily. That is the reason the input material is high relevant to memorize to form valued-information, knowledge. Organization of material into long-term memory involves sorting, relating, arranging, and grouping information so that it can be worth to been memorized. It is important to note that most application AI models have more trouble remembering/learning of what data they should remember/learn. Therefore, as the effective teacher will help the memory process by introducing the student to various organizational techniques. And if great teaching effects intended learning outcomes, learning is achieving those intended outcomes. However, the learning of teaching style is more various than traditional learning.. 3.5 Conceptual Framework During the Middle Period (mid 1900s), Knowledge is just thought of as the transformation of sensory inputs into associated thought, and the realization that sensory inputs are transformed prior to storage. In the early twentieth century, Knowledge is still considered as a framework of stimulus and response (S-R). The profound breakthrough of this period is that by studying S-R, one can gain insight into the working of cognitive knowledge. This research and its viewpoint of knowledge learning are largely based on narrow term of cognitive psychology, information processing theory. Besides, S-R of cognitive psychology research is historically analogous to the black box testing. Following these two aspects, this work applied the cognitive learning to modify the learning process of 27.
(38) traditional soft techniques to increase the efficiency of forming knowledge storage. Furthermore, according to the accuracy ratio of LCSs model, we choose its best ones, XCS, as a kernel of that black box, we tested as a memorizing/learning model. We combine the information process theory and learning type to initial the concept of the dual learning mode framework, shown as Figure 3. It contains two parts: Knowledge Education learning and Reinforcement-Rehearsal(R-R) learning.. Figure 3. Education Learning Flow and Reinforcement-Rehearsal Learning Flow. To construct an effective learning model, two aspects should be considered. Table 3 concludes some attributes of the proposed dual perspective model, Education and R-R perspective. The first is education base summarized by the teaching literatures. Knowledge is worth to be used as materials to teach students/train models. Nevertheless, using knowledge rule to build an expert system is not sufficient flexibility. That is, the learning/training model which utilized the knowledge materials as inputs is taken into consideration to form a learning model with education perspective. In this part, the model is as a learner, and the model operator as a teacher. The memory belongs to long-term memory (LTM) with permanent store. Knowledge transmission and the model that learns others’ thinking are the two major purposes. As for the superficial knowledge by the other learning model with reinforcement28.
(39) rehearsal (R-R) perspective, it would be sum up the general experiences which come from stimulus-response actions. R-R perspective learning model is just like traditional soft computing techniques. Normally, utilizing huge amounts data as inputs to the “learning” model is the machine learning type which major method is trial and error style. In this part, it owns working memory (WM) and short-memory (STM). WM is a pre-storage of the stimulus-response. STM is a storage that maintains the short-term information in the model for rehearsal. If it is possible, the relative experiences would be concluded to rules which could be verified to form knowledge. Objectively, the entire process of this part is no efficiency because of trial and error method. That is because of the complexity of the learning, this work portrays the Education and R-R learning model to increase the efficiency of knowledge transmission and the accuracy of experience rules generation.. 29.
(40) Table 3. Dual-Mode Learning Model of Education-Dominated and R-R Perspectives Learning Attributes. Education Perspective. R-R Perspective. Subject. To Teach/Train the Model. Model as Learner Centered. Input source. Knowledge. Raw Data. Learning type. Teaching Style Learning. Trial and Error Learning. Process steps. Model Memorizes Knowledge. Model Summarizes Experiences. Model type. Model as Memorizer. Model as Processor. Memory type. Rote Long Term Memory. Active Short Term Memory. Memory Capacity. Unlimited. Limited. Practice type. Repetitive. R-R. Output. Knowledge is stored. Experiences Rule is Created, but Need to be Verified. Instruction type. Sequential Instruction. Adaptive Learning. Training Flow. Operator Learning. Thinking Type. Model Learn Others' Thinking. Model Develop and Reflect on its Self-Own Thinking. Knowledge. Knowledge Transmission. Knowledge Formation Verified Experiences. Operator type. Operator as a teacher. Operator as a Data Inputter. Model type. Mechanistic/Training. Organismic/Evolution. Performance. High Efficiency. Low Efficiency. Flexibility. Low, Difficult to modify. High Flexibility but Need to Be Verified. Manages. 30. Model Model Self-Tuning Pre-parameter. the. by. by.
(41) Chapter 4. Education and R-R Model Based on XCS According to the cognition theory, knowledge transmission by education is proven as a high efficiency mechanism of learning to human. As the human learning, the teaching-base learning style to form knowledge should be paid more attention on. As for machine learning, the machine is a software system running on a computer that could provide the ability to large continuous logical processes, while many kinds of learning algorithms are analogous to the human trial and error learning. Thus, this work combines the advantages of these two aspects to propose a dual perspective learning model which is implemented the conceptual framework that describes in chapter 3. rehearsal Stim ilus. Sensor. ST M (lim ited capacity ). retrieval. L TM (perm anent m em ory store). R esponse output. Figure 4. Richard Atkinson and Richard Shiffrin 1968 [48] proposed a theoretical model for the flow of information through the human information processor. The dual perspective learning concept is knowledge education and reinforcementrehearsal respectively. In addition to involve the cognition theory, information process theory (IPT) [47], [48], the relationship of LTM and STM are rehearsal and retrieval, shown as Figure 4. Although LTM has ever been mentioned by the original LCS, the definitions of LCS’s LTM and IPT’s LTM are different. In Fact, the function of LCS’s STM is just equal to the function of IPT’s WM, and the same aspect, LCS’s LTM is equal to IPT’s STM. As for LTM of IPT, it indeed owns an unlimited capacity to store information which is different from LCS’s memory. While the inference of these memories is derived, the conceptual framework would enhanced by more considering the retrieval relation of LTM and STM, shown as Figure 5. LTM is presented to store knowledge base, and rules are collected in 31.
數據
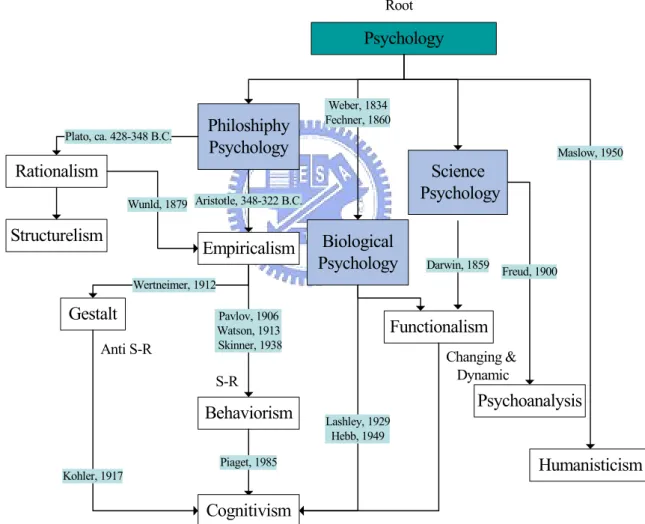
![Figure 2. Information Process Theory proposed by Gagne [47], [50].](https://thumb-ap.123doks.com/thumbv2/9libinfo/8130352.166219/34.892.158.794.205.360/figure-information-process-theory-proposed-gagne.webp)

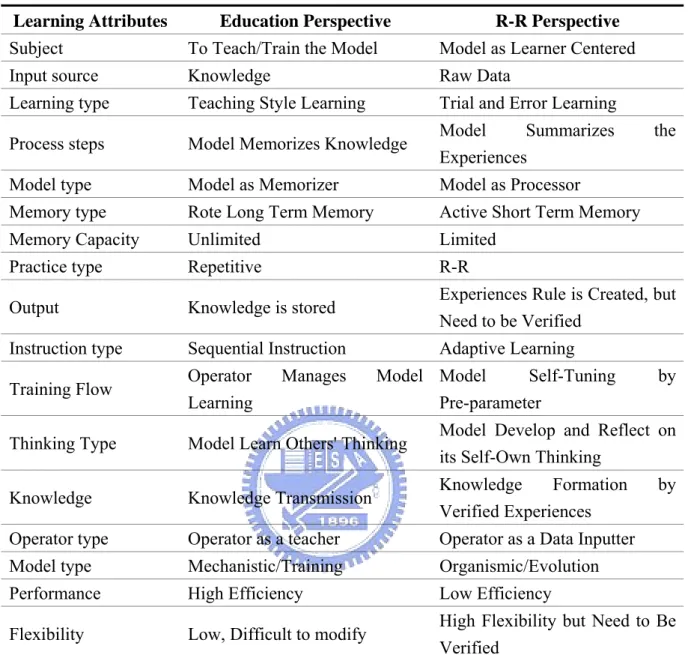
+7
相關文件
哈佛大學教授夏爾(Jeanne Chall)1983 年曾以六個階段描述兒童學習 閱讀的歷程,這六個階段又可分成兩大部份,分別是: 「學習如何讀」(learn to read ),「透過閱讀學習知識」(read to
問題類型 非結構化問題 結構化問題 結構化問題 結構化問題 學習能力 不具學習能力 不具學習能力 自錯誤中學習 自錯誤中學習 學習能力 不具學習能力 不具學習能力
學習範疇 主要學習成果 級別 級別描述 學習成果. 根據學生的認知發展、學習模式及科本
多修之學 分數得認 列為自由 選修. 得修習各
多修之學 分數得認 列為自由 選修. 得修習各
Rebecca Oxford (1990) 將語言學習策略分為兩大類:直接性 學習策略 (directed language learning strategies) 及間接性學 習策略 (in-directed
The difference resulted from the co- existence of two kinds of words in Buddhist scriptures a foreign words in which di- syllabic words are dominant, and most of them are the
透過六個學習範疇,建 構 學科知識 、發展 共通 能力 、以及培養正面的